Prediction of Return of Spontaneous Circulation in a Pediatric Swine Model of Cardiac Arrest Using Low-Resolution Multimodal Physiological Waveforms.
IEEE journal of biomedical and health informatics(2023)
摘要
Monitoring physiological waveforms, specifically hemodynamic variables (e.g., blood pressure waveforms) and end-tidal CO2 (EtCO2), during pediatric cardiopulmonary resuscitation (CPR) has been demonstrated to improve survival rates and outcomes when compared to standard depth-guided CPR. However, waveform guidance has largely been based on thresholds for single parameters and therefore does not leverage all the information contained in multimodal data. We hypothesize that the combination of multimodal physiological features improves the prediction of the return of spontaneous circulation (ROSC), the clinical indicator of short-term CPR success. We used machine learning algorithms to evaluate features extracted from eight low- resolution (4 samples per minute) physiological waveforms to predict ROSC. The waveforms were acquired from the 2nd to 10 minute of CPR in pediatric swine models of cardiac arrest (N = 89, 8-12kg). The waveforms were divided into segments with increasing length (both forward and backward) for feature extraction, and machine learning algorithms were trained for ROSC prediction. For the full CPR period (2 to 10 minute), the area under the receiver operating characteristics curve (AUC) was 0.93 (95% CI: 0.87-0.99) for the multivariate model, 0.70 (0.55-0.85) for EtCO2 and 0.80 (0.67-0.93) for coronary perfusion pressure. The best prediction performances were achieved when the period from the 6 to the 10 minute was included. Poor predictions were observed for some individual waveforms, e.g., right atrial pressure. In conclusion, multimodal waveform features carry relevant information for ROSC prediction. Using multimodal waveform features in CPR guidance has the potential to improve resuscitation success and reduce mortality.
更多查看译文
关键词
cardiac arrest,pediatric swine model,spontaneous circulation,physiological,low-resolution
AI 理解论文
溯源树
样例
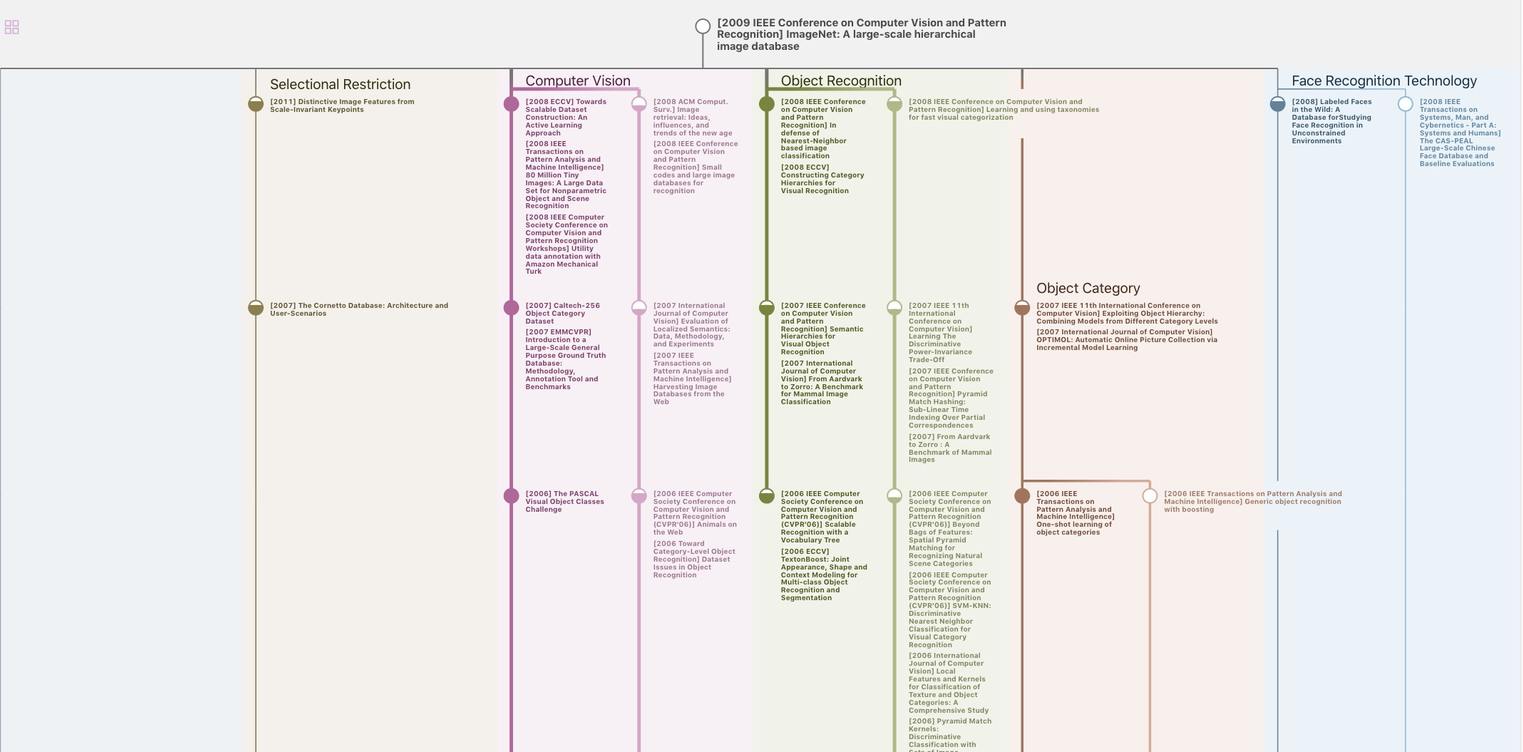
生成溯源树,研究论文发展脉络
Chat Paper
正在生成论文摘要