Syntactic vs Semantic Linear Abstraction and Refinement of Neural Networks
CoRR(2023)
摘要
Abstraction is a key verification technique to improve scalability. However, its use for neural networks is so far extremely limited. Previous approaches for abstracting classification networks replace several neurons with one of them that is similar enough. We can classify the similarity as defined either syntactically (using quantities on the connections between neurons) or semantically (on the activation values of neurons for various inputs). Unfortunately, the previous approaches only achieve moderate reductions, when implemented at all. In this work, we provide a more flexible framework where a neuron can be replaced with a linear combination of other neurons, improving the reduction. We apply this approach both on syntactic and semantic abstractions, and implement and evaluate them experimentally. Further, we introduce a refinement method for our abstractions, allowing for finding a better balance between reduction and precision.
更多查看译文
关键词
semantic linear abstraction,neural,refinement,networks
AI 理解论文
溯源树
样例
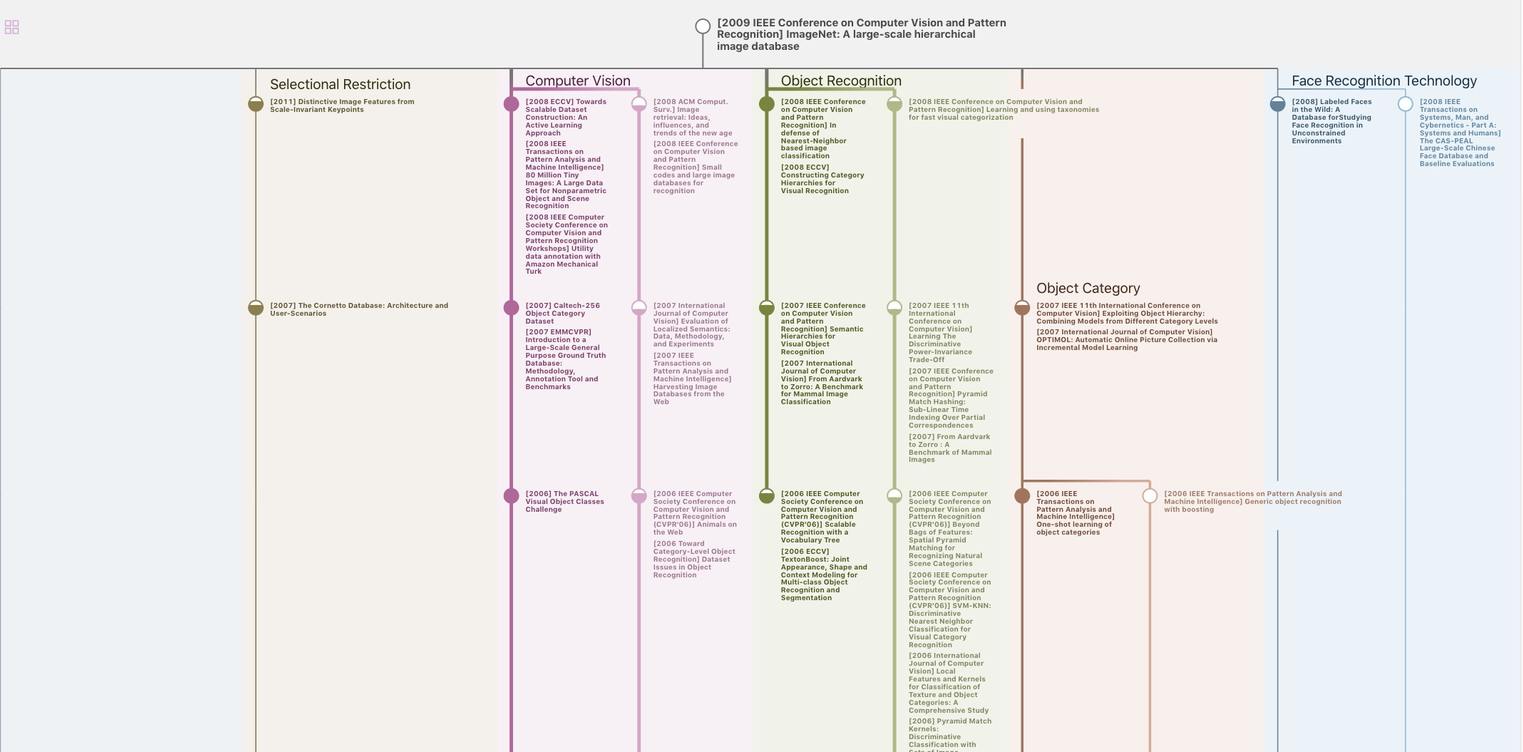
生成溯源树,研究论文发展脉络
Chat Paper
正在生成论文摘要