Bimodular continuous attractor neural networks with static and moving stimuli.
Physical review. E(2023)
摘要
We investigated the dynamical behaviors of bimodular continuous attractor neural networks, each processing a modality of sensory input and interacting with each other. We found that when bumps coexist in both modules, the position of each bump is shifted towards the other input when the intermodular couplings are excitatory and is shifted away when inhibitory. When one intermodular coupling is excitatory while another is moderately inhibitory, temporally modulated population spikes can be generated. On further increase of the inhibitory coupling, momentary spikes will emerge. In the regime of bump coexistence, bump heights are primarily strengthened by excitatory intermodular couplings, but there is a lesser weakening effect due to a bump being displaced from the direct input. When bimodular networks serve as decoders of multisensory integration, we extend the Bayesian framework to show that excitatory and inhibitory couplings encode attractive and repulsive priors, respectively. At low disparity, the bump positions decode the posterior means in the Bayesian framework, whereas at high disparity, multiple steady states exist. In the regime of multiple steady states, the less stable state can be accessed if the input causing the more stable state arrives after a sufficiently long delay. When one input is moving, the bump in the corresponding module is pinned when the moving stimulus is weak, unpinned at intermediate stimulus strength, and tracks the input at strong stimulus strength, and the stimulus strengths for these transitions increase with the velocity of the moving stimulus. These results are important to understanding multisensory integration of static and dynamic stimuli.
更多查看译文
关键词
continuous attractor,neural networks,static
AI 理解论文
溯源树
样例
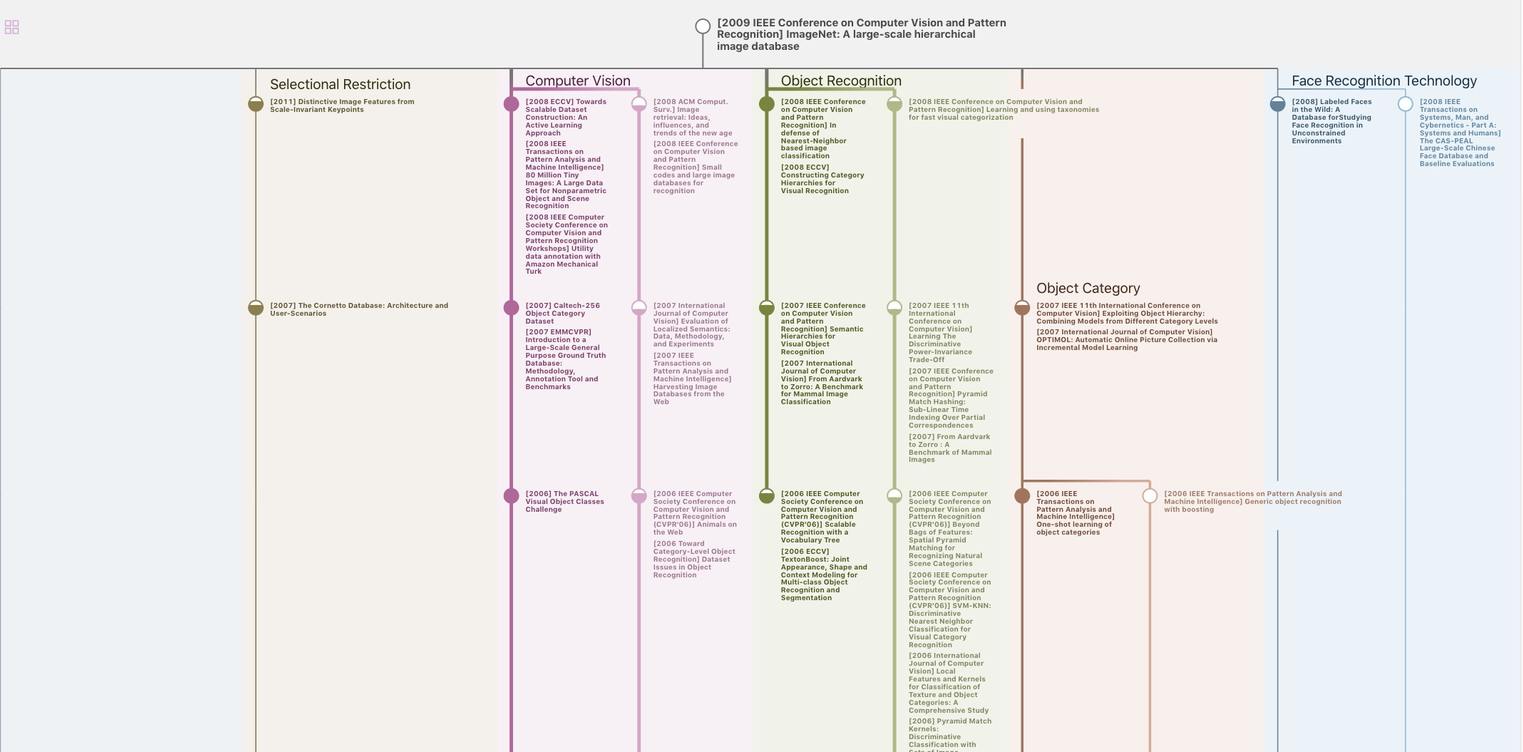
生成溯源树,研究论文发展脉络
Chat Paper
正在生成论文摘要