Autonomous perception and adaptive standardization for few-shot learning
KNOWLEDGE-BASED SYSTEMS(2023)
摘要
Identifying unseen classes with limited labeled data for reference is a challenging task, which is also known as few-shot learning. Generally, a knowledge-rich model is more robust than a knowledge-poor model when facing novel situations, and an intuitive way to enrich knowledge is to find additional training data, but this is not compatible with the principle of few-shot learning which aims to reduce reliance on big data. In contrast, improving the utilization of existing data is a more attractive option. In this paper, we propose a batch perception distillation approach, which improves the utilization of existing data by guiding individual classification with the intermixed information across a batch. In addition to data utilization, obtaining robust feature representation is also a concern. Specifically, the widely adopted metric-based few-shot classification approach classifies unseen testing classes by comparing the extracted features of different novel samples, which requires that the extracted features can accurately represent the class-related clues of the input images. In this paper, we propose a salience perception attention that enables the model to focus more easily on key clues in images, which helps to reduce the interference of irrelevant factors during classification. To overcome the distribution gap between the training classes and the unseen testing classes, we propose a weighted centering post-processing that standardizes the testing data according to the similarity between the training and testing classes. By combining the three proposed components, our method achieves superior performance on four widely used few-shot image classification datasets.(c) 2023 Elsevier B.V. All rights reserved.
更多查看译文
关键词
Few-shot learning,Image classification,Deep learning,Feature extraction
AI 理解论文
溯源树
样例
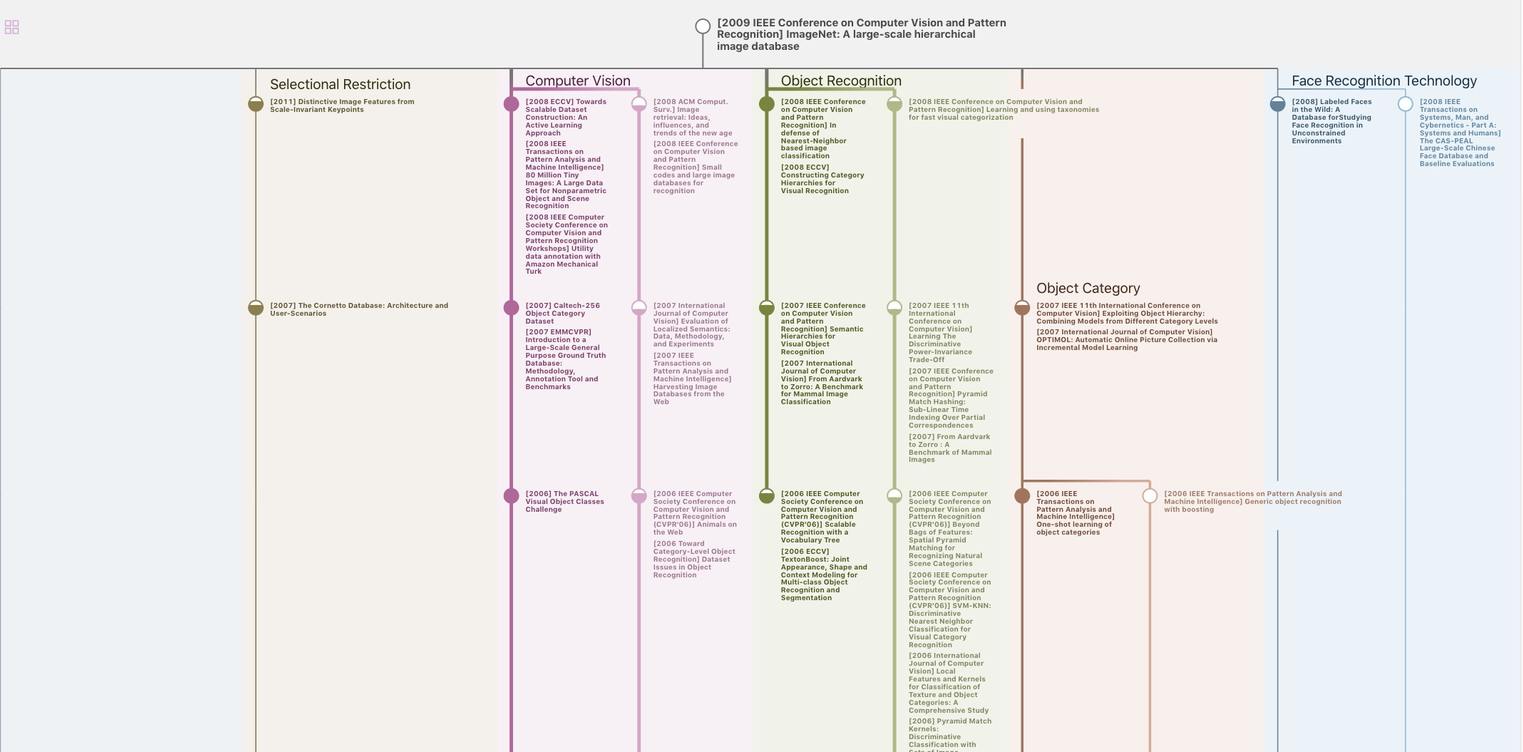
生成溯源树,研究论文发展脉络
Chat Paper
正在生成论文摘要