Smart contracts vulnerability detection model based on adversarial multi-task learning
Journal of Information Security and Applications(2023)
摘要
Vulnerability detection is important for smart contracts because of their immutable and irreversible features. In this work, a new detection method based on adversarial multi-task learning is proposed to improve the accuracy of existing vulnerability detection methods, which is based on the multi-task learning framework, including a shared part and a task-specific part. We optimize the multi-task learning frameworks and propose the mixed parameter sharing method to make each task not only maintain its uniqueness, but also share features with other tasks, which helps solve the problem that the hard parameter sharing method cannot constrain the underlying shared layer and improve the quality of extracted features. In addition, we introduce adversarial transfer learning to reduce noise pollution caused by the private feature and interference between the general feature and the private feature. We experimented on datasets obtained from our previous work, and the experimental results prove that our proposed model can judge whether there are vulnerabilities in smart contracts and then identify their types. Additionally, the results also show that our model effectively improves detection accuracy and has an advantage in performance over representative methods.
更多查看译文
关键词
vulnerability,learning,multi-task
AI 理解论文
溯源树
样例
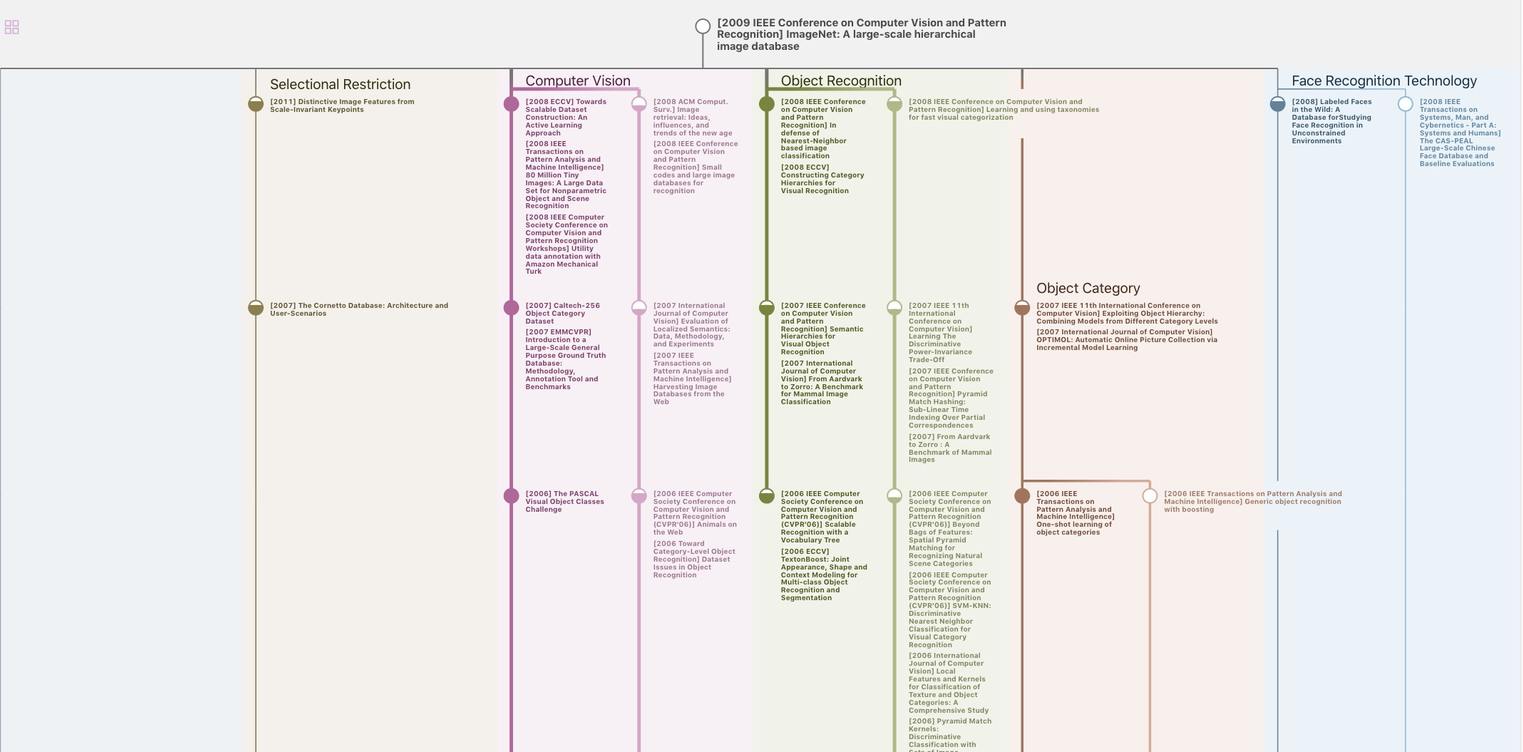
生成溯源树,研究论文发展脉络
Chat Paper
正在生成论文摘要