TDRLM: Stylometric learning for authorship verification by Topic-Debiasing
Expert Systems with Applications(2023)
摘要
Authorship verification is the task of determining if a candidate is the real author of the provided sequences of texts. Stylometric learning is the study of the writing style by analyzing the textual data through computational approaches for many linguistic tasks such as authorship verification, authorship identification, etc. In recent years, Deep learning has been used as an effective solution to stylometric learning for authorship verification. However, most of the existing studies do not consider the semantic biases captured by the model, and the learned stylometric features include a strong indicator of the topical biases. During the extraction of stylometric features, we found that specific words related to the latent topics impact the performance of machine learning algorithms and, therefore, are not generalizable toward out-of-sample authors. In this paper, we propose a latent topic score dictionary with a Topic-Debiasing Representation Learning Model (TDRLM) for stylometric representation learning on the problem of authorship verification. The model applies position-specific topic scores on a topic-debiasing attention mechanism in order to adjust the tokenized texts based on the topical bias. The experimental results show that our proposed approach achieves the best performance with the highest Area Under Curve (AUC) of 92.47% for the Twitter-Foursquare dataset and 93.11% for the (ICWSM) Twitter dataset, both better than the state-of-the-art stylometric learning models and the up-trained language models.
更多查看译文
关键词
Authorship verification,Stylometric learning,Text analysis,Topic-Debiasing Attention
AI 理解论文
溯源树
样例
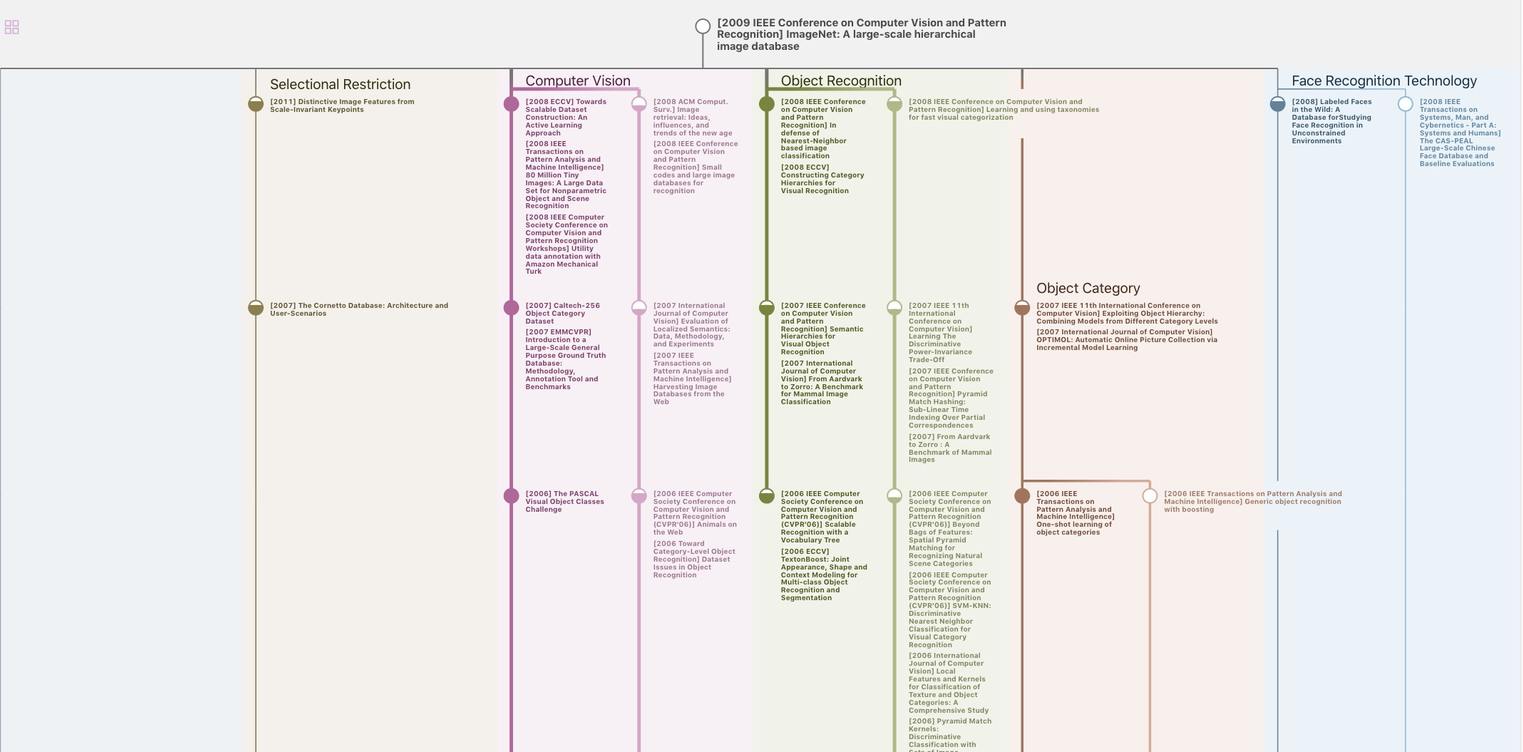
生成溯源树,研究论文发展脉络
Chat Paper
正在生成论文摘要