Adaptive time series representation for out-of-round railway wheels fault diagnosis in wayside monitoring
Engineering Failure Analysis(2023)
摘要
•A novel method of signal segmentation, leveraging the sensitivity of each type of sensor. The stable temporal structure of the strain gauge signal was used to segment both signals, while feature extraction was focused on the most damage-sensitive accelerometer, less impacted by non-linear operational and environmental factors;•Fault localization method proposed in this study offers a wheel-based approach with implicit indexing and axle count, thereby providing infrastructure managers and maintenance personnel with more comprehensive and valuable information;•The methodology doesn’t require the installment of a series of sensors on the rail, reducing the cost of installation and maintenance. By only using one accelerometer and strain gauge data quality wasn’t compromised;•The final anomaly detection stage not only successfully distinguished a healthy wheel from defective ones, but also provided a damage indicator for severity identification, regardless of different wheel profiles, track irregularities, train speeds, position of the sensors and artificial noise, associated to other environmental and operational variations.
更多查看译文
关键词
Machine Learning,Fault diagnosis,Wheel Out-of-roundess,Wayside monitoring,Artificial Intelligence
AI 理解论文
溯源树
样例
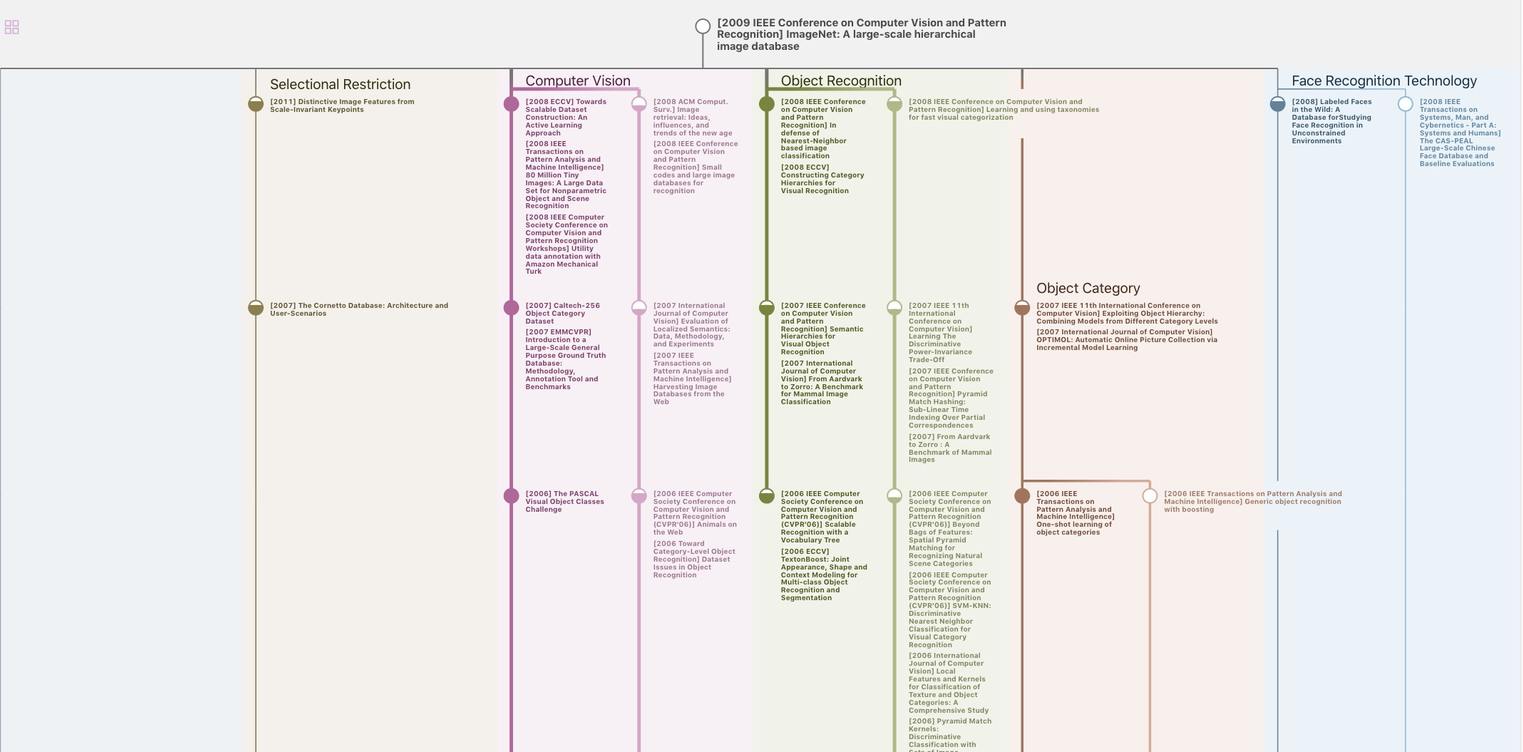
生成溯源树,研究论文发展脉络
Chat Paper
正在生成论文摘要