Data-oriented ensemble predictor based on time series classifiers for fraud detection
Electric Power Systems Research(2023)
摘要
Non-Technical Losses (NTL) remain a challenge because electricity theft is prevalent in traditional meters and smart metering systems and structures. They cause financial losses that affect the security of supply and lead to a cooperative burden because NTL are incorporated in the expense tariff and are paid by all customers in countries such as India, China, Brazil, Tunisia, Uruguay, and others. A significant number of methods have been proposed to detect NTL, but they cannot precisely recognize this type of fraud, which enables companies to better identify the fraud’s origin and evaluate its financial impact. In addition, it is important to combine several Time-Series Classification (TSC) algorithms which consider the time-dependent nature of energy consumption data. We propose a data-oriented heterogeneous ensemble predictor based on time series classifiers for NTL detection, called DETECT. It classifies energy consumption samples either as honest or as fraudulent and further categorizes the type of fraud which might have been committed based on the analysis of the consumption patterns of each sample. DETECT is a fraud detection algorithm that has the ability to analyze and classify NTL frauds effectively regardless of the dataset. Using our proposed approach, we obtained a performance improvement with a Detection Rate (DR) value equal to 93.45% and a False Positive Rate (FPR) value equal to 1.61%. DETECT focuses on time-series data, which enables the development of a method that better interprets real-world scenarios and it is more error-resistant.
更多查看译文
关键词
Ensemble,Fraud detection,Machine learning,Smart meter
AI 理解论文
溯源树
样例
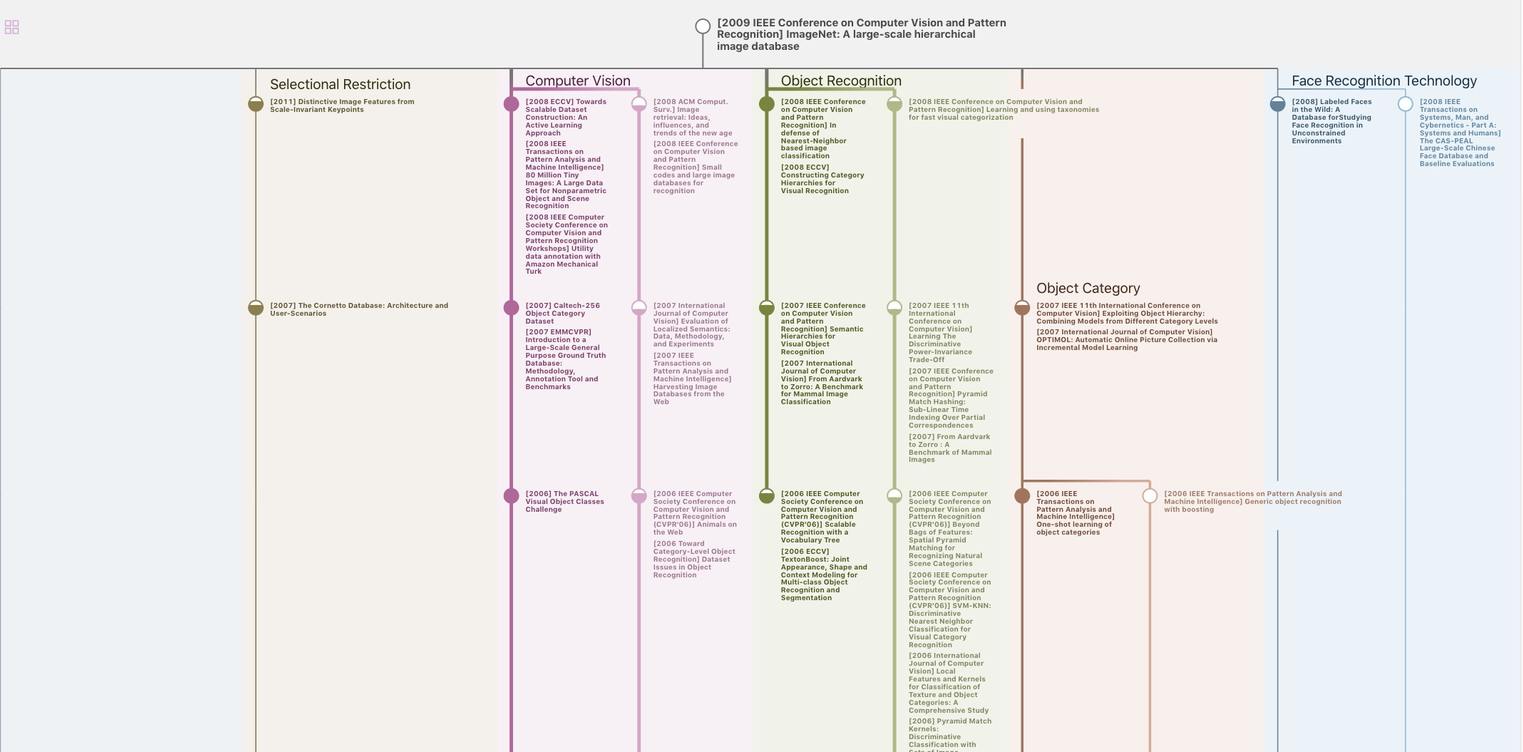
生成溯源树,研究论文发展脉络
Chat Paper
正在生成论文摘要