A practical study of methods for deriving insightful attribute importance rankings using decision bireducts
Information Sciences(2023)
摘要
Subject matter experts (SMEs) often rely on attribute importance rankings to verify machine learning models, acquire insights into their outcomes, and gain a deeper understanding of the investigated phenomena. To further increase their usefulness, we introduce a new approach to the evaluation of attribute rankings produced by any machine learning method. As a real-world case study, we investigate the attribute importance scores produced using XGBoost and decision bireducts on the data gathered by an HR company, where the goal is to predict the willingness of candidates to change their job. For this task, XGBoost delivers accurate models but fails to identify many attributes that are important to SMEs. In comparison, decision bireducts lead to models that are easier to interpret and explore the data with a higher focus on the diversity of attributes. The ensembles of decision bireducts deliver comparable accuracy and their associated attribute rankings are more insightful than those of XGBoost.
更多查看译文
关键词
insightful attribute importance rankings,decision
AI 理解论文
溯源树
样例
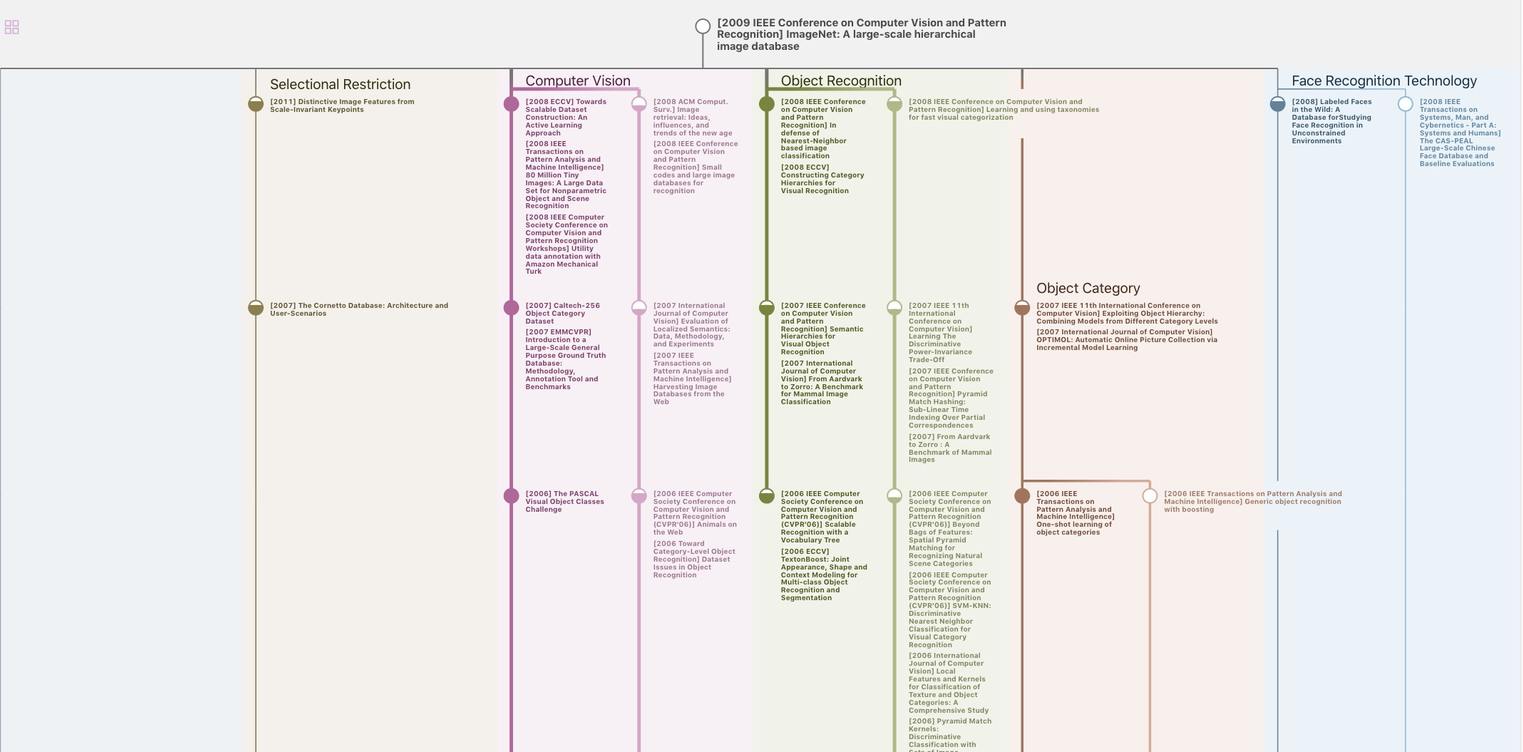
生成溯源树,研究论文发展脉络
Chat Paper
正在生成论文摘要