Attention-Enhanced Co-Interactive Fusion Network (AECIF-Net) for automated structural condition assessment in visual inspection
AUTOMATION IN CONSTRUCTION(2024)
摘要
Efficiently monitoring the condition of civil infrastructure requires automating the structural condition assessment in visual inspection. This paper proposes an Attention -Enhanced Co -Interactive Fusion Network (AECIF-Net) for automatic structural condition assessment in visual bridge inspection. AECIF-Net can simultaneously parse structural elements and segment surface defects on the elements in inspection images. It integrates two task -specific relearning subnets to extract task -specific features from an overall feature embedding. A co -interactive feature fusion module further captures the spatial correlation and facilitates information sharing between tasks. Experimental results demonstrate that the proposed AECIF-Net outperforms the current state-of-the-art approaches, achieving promising performance with 92.11% mIoU for element segmentation and 87.16% mIoU for corrosion segmentation on the test set of the new benchmark dataset Steel Bridge Condition Inspection Visual (SBCIV). An ablation study verifies the merits of the designs for AECIF-Net, and a case study demonstrates its capability to automate structural condition assessment.
更多查看译文
关键词
Infrastructure inspection,Multi-task learning,Spatial attention,Structural element segmentation,Defect segmentation
AI 理解论文
溯源树
样例
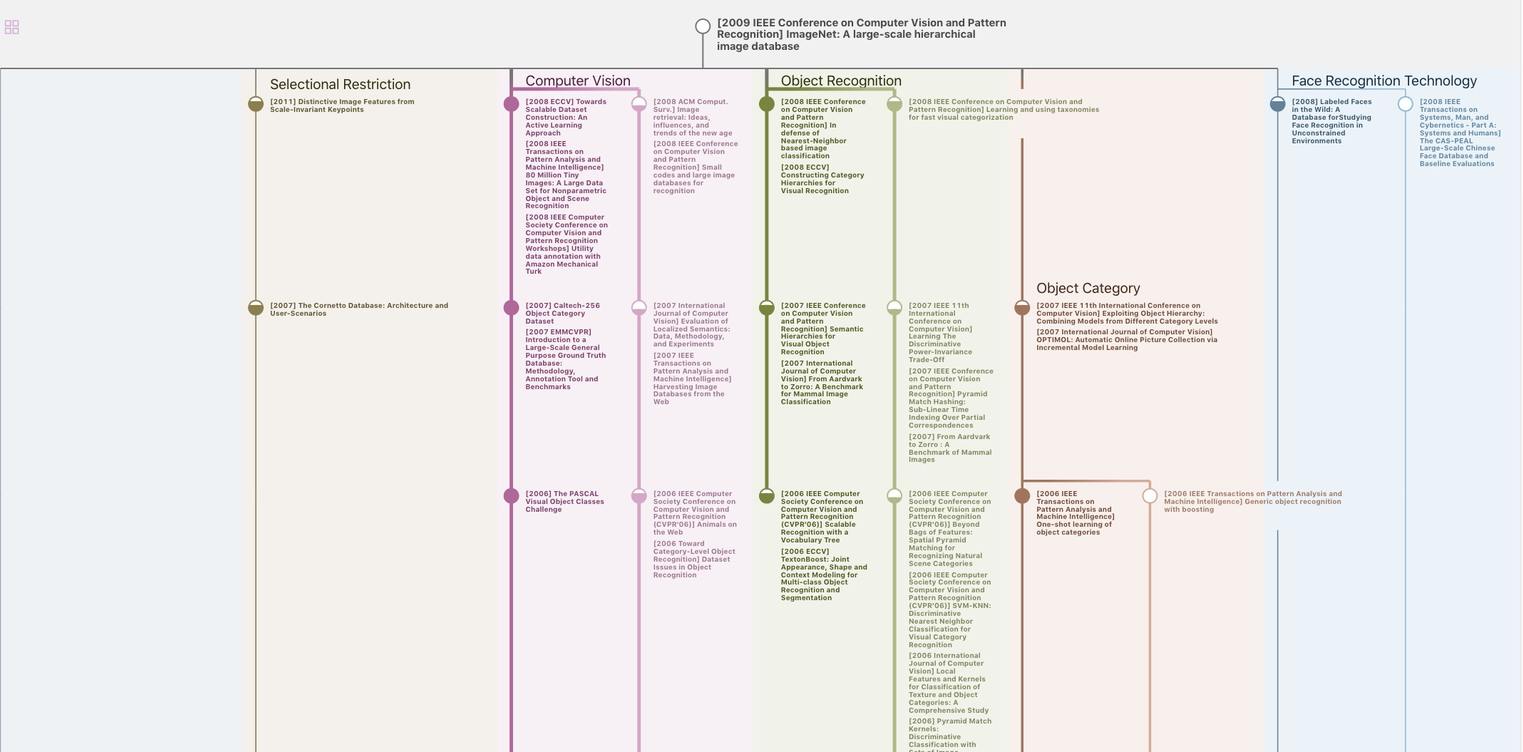
生成溯源树,研究论文发展脉络
Chat Paper
正在生成论文摘要