SubT-MRS: A Subterranean, Multi-Robot, Multi-Spectral and Multi-Degraded Dataset for Robust SLAM
CoRR(2023)
摘要
In recent years, significant progress has been made in the field of simultaneous localization and mapping (SLAM) research. However, current state-of-the-art solutions still struggle with limited accuracy and robustness in real-world applications. One major reason is the lack of datasets that fully capture the conditions faced by robots in the wild. To address this problem, we present SubT-MRS, an extremely challenging real-world dataset designed to push the limits of SLAM and perception algorithms. SubT-MRS is a multi-modal, multi-robot dataset collected mainly from subterranean environments having multi-degraded conditions including structureless corridors, varying lighting conditions, and perceptual obscurants such as smoke and dust. Furthermore, the dataset packages information from a diverse range of time-synchronized sensors, including LiDAR, visual cameras, thermal cameras, and IMUs captured using varied vehicular motions like aerial, legged, and wheeled, to support research in sensor fusion, which is essential for achieving accurate and robust robotic perception in complex environments. To evaluate the accuracy of SLAM systems, we also provide a dense 3D model with sub-centimeter-level accuracy, as well as accurate 6DoF ground truth. Our benchmarking approach includes several state-of-the-art methods to demonstrate the challenges our datasets introduce, particularly in the case of multi-degraded environments.
更多查看译文
关键词
subt-mrs,multi-robot,multi-spectral,multi-degraded
AI 理解论文
溯源树
样例
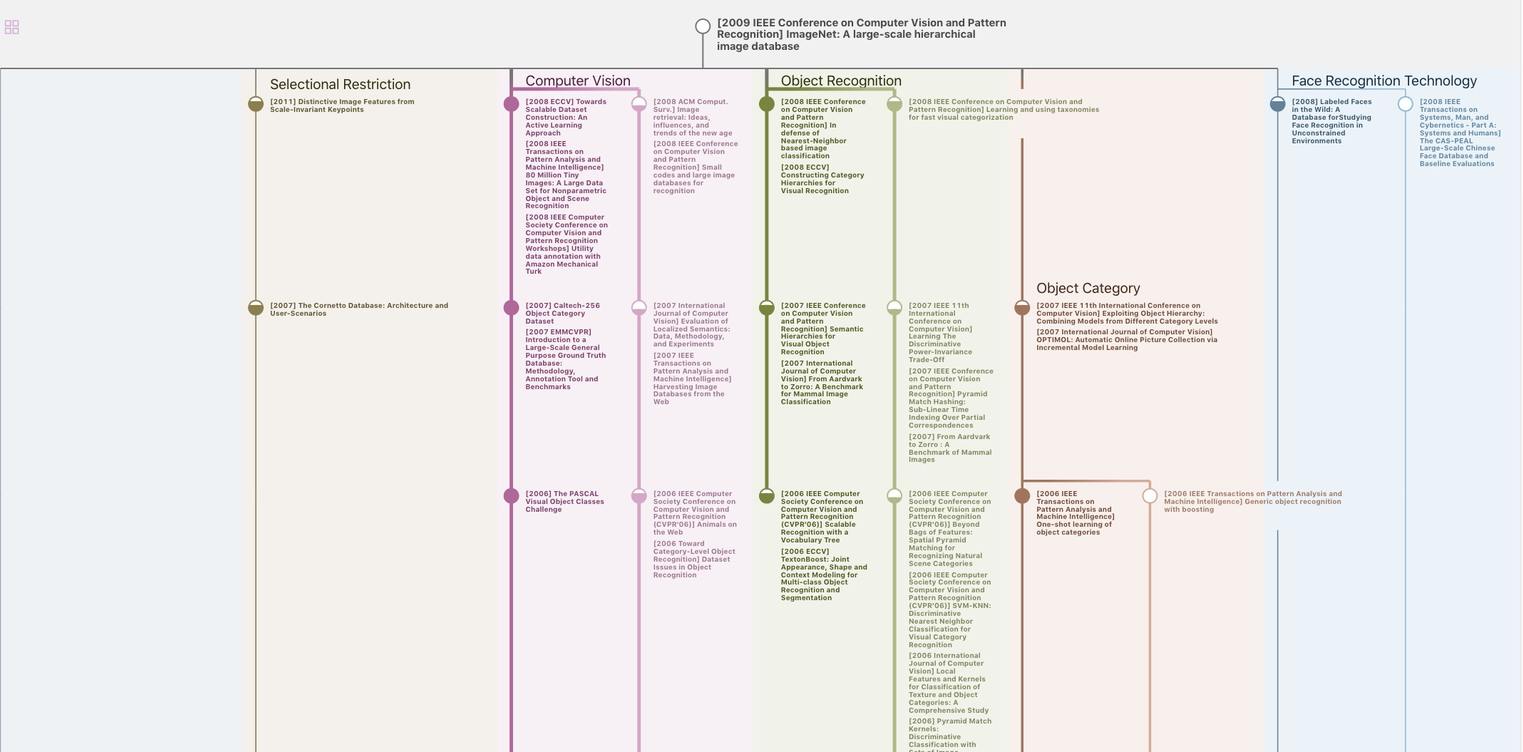
生成溯源树,研究论文发展脉络
Chat Paper
正在生成论文摘要