Short Boolean Formulas as Explanations in Practice
LOGICS IN ARTIFICIAL INTELLIGENCE, JELIA 2023(2023)
摘要
We investigate explainability via short Boolean formulas in the data model based on unary relations. As an explanation of length k, we take a Boolean formula of length k that minimizes the error with respect to the target attribute to be explained. We first provide novel quantitative bounds for the expected error in this scenario. We then also demonstrate how the setting works in practice by studying three concrete data sets. In each case, we calculate explanation formulas of different lengths using an encoding in Answer Set Programming. The most accurate formulas we obtain achieve errors similar to other methods on the same data sets. However, due to overfitting, these formulas are not necessarily ideal explanations, so we use cross validation to identify a suitable length for explanations. By limiting to shorter formulas, we obtain explanations that avoid overfitting but are still reasonably accurate and also, importantly, human interpretable.
更多查看译文
关键词
Boolean formula size,Explainability,Interpretable AI,Overfitting error,Answer Set Programming,Boolean optimization
AI 理解论文
溯源树
样例
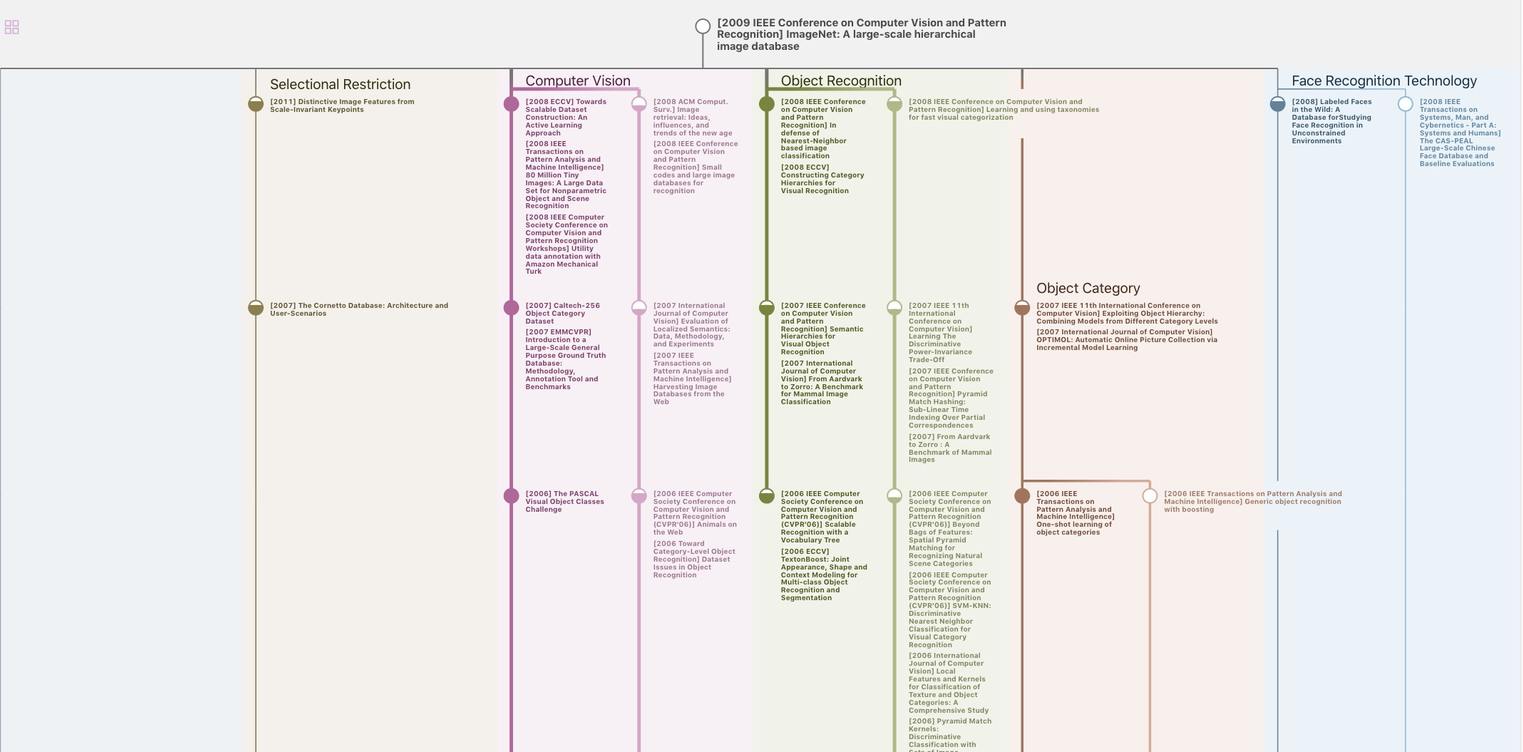
生成溯源树,研究论文发展脉络
Chat Paper
正在生成论文摘要