Permeability Prediction of Nanoscale Porous Materials Using Discrete Cosine Transform-Based Artificial Neural Networks
Materials (Basel, Switzerland)(2023)
摘要
The permeability of porous materials determines the fluid flow rate and aids in the prediction of their mechanical properties. This study developed a novel approach that combines the discrete cosine transform (DCT) and artificial neural networks (ANN) for permeability analysis and prediction in digital rock images, focusing on nanoscale porous materials in shale formations. The DCT effectively captured the morphology and spatial distribution of material structure at the nanoscale and enhanced the computational efficiency, which was crucial for handling the complexity and high dimensionality of the digital rock images. The ANN model, trained using the Levenberg-Marquardt algorithm, preserved essential features and demonstrated exceptional accuracy for permeability prediction from the DCT-processed rock images. Our approach offers versatility and efficiency in handling diverse rock samples, from nanoscale shale to microscale sandstone. This work contributes to the comprehension and exploitation of unconventional resources, especially those preserved in nanoscale pore structures.
更多查看译文
关键词
permeability,nanoscale,porous material,discrete cosine transform,artificial neural network
AI 理解论文
溯源树
样例
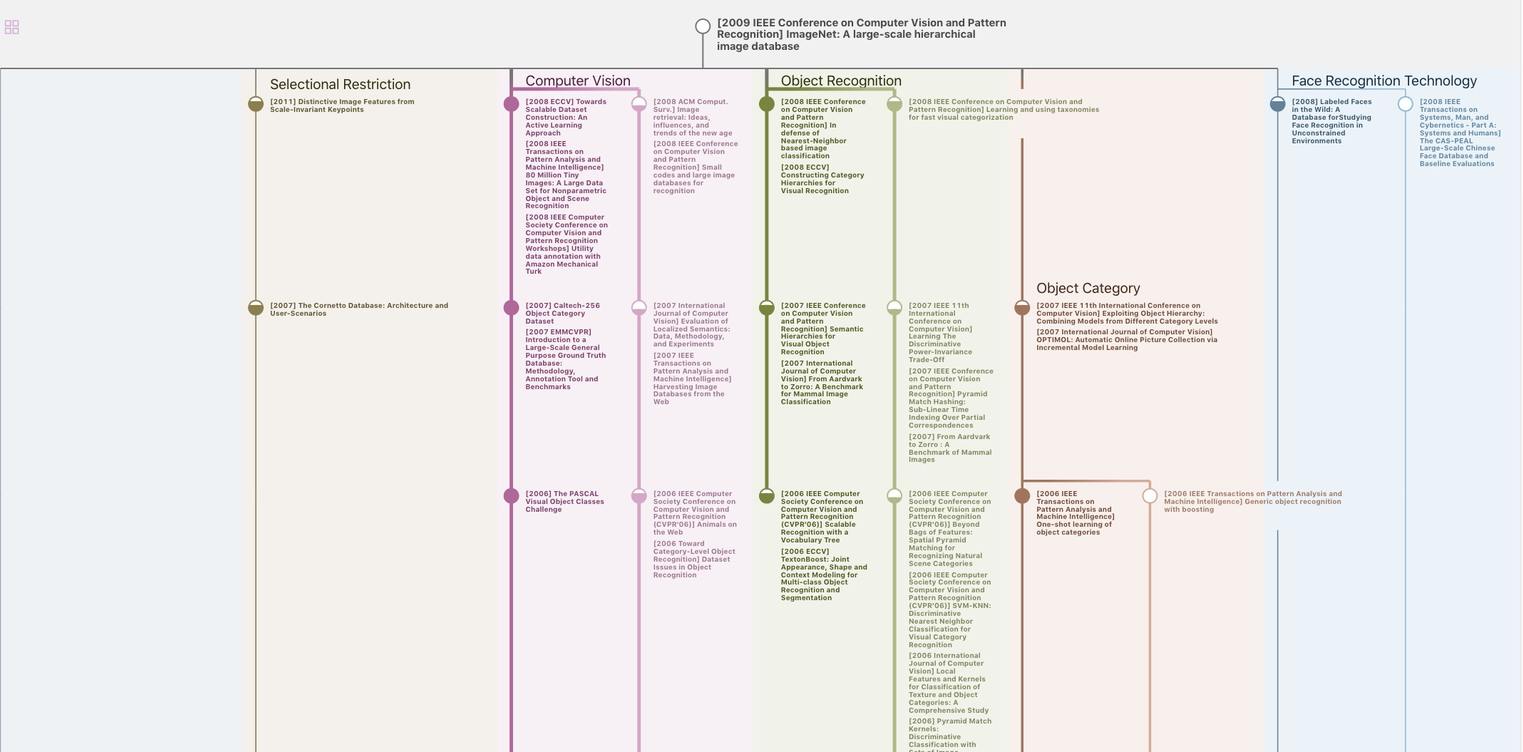
生成溯源树,研究论文发展脉络
Chat Paper
正在生成论文摘要