Lightweight image de-snowing: A better trade-off between network capacity and performance.
Neural networks : the official journal of the International Neural Network Society(2023)
摘要
The single image de-snowing task is an essential topic in computer vision, as images captured on snowy days degrade the performance of current vision-based intelligent systems. Existing methods build complex network structures with numerous parameters to pursue continuous performance improvement. Nonetheless, they generally ignore the negative impact of large memory consumption in real applications. This paper aims to address the above problem by making a trade-off between network capacity and performance. We propose two novel networks suitable for different application scenarios. For devices with small memory and requiring fast inference speed, we propose an extremely lightweight recursive network (XLRNet). XLRNet is constructed by a single recursive strategy and two novel lightweight modules. For devices with large memory and pursuing better de-snowing performance, we propose a coupled lightweight dual recursive network (CLDRNet). CLDRNet cascades two XLRNets by a novel dual recursive strategy and a novel dual coupled LSTM module (DC-LSTM). Extensive experiments demonstrate the effectiveness and superiority of our two models on three synthetic datasets and real-world datasets.
更多查看译文
关键词
network capacity,de-snowing,trade-off
AI 理解论文
溯源树
样例
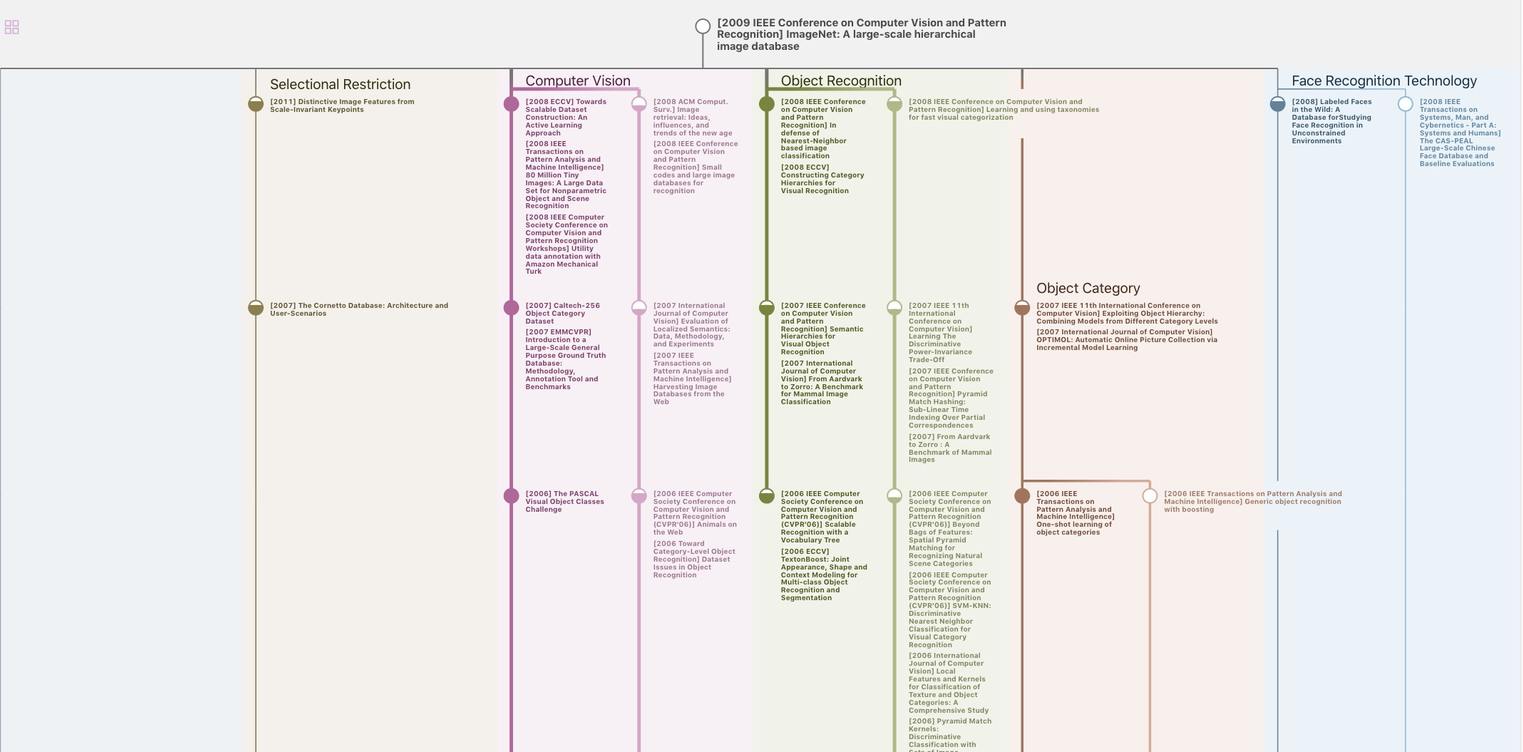
生成溯源树,研究论文发展脉络
Chat Paper
正在生成论文摘要