Distilling Large Language Models for Biomedical Knowledge Extraction: A Case Study on Adverse Drug Events
CoRR(2023)
摘要
Large language models (LLMs), such as GPT-4, have demonstrated remarkable capabilities across a wide range of tasks, including health applications. In this paper, we study how LLMs can be used to scale biomedical knowledge curation. We find that while LLMs already possess decent competency in structuring biomedical text, by distillation into a task-specific student model through self-supervised learning, substantial gains can be attained over out-of-box LLMs, with additional advantages such as cost, efficiency, and white-box model access. We conduct a case study on adverse drug event (ADE) extraction, which is an important area for improving care. On standard ADE extraction evaluation, a GPT-3.5 distilled PubMedBERT model attained comparable accuracy as supervised state-of-the-art models without using any labeled data. Despite being over 1,000 times smaller, the distilled model outperformed its teacher GPT-3.5 by over 6 absolute points in F1 and GPT-4 by over 5 absolute points. Ablation studies on distillation model choice (e.g., PubMedBERT vs BioGPT) and ADE extraction architecture shed light on best practice for biomedical knowledge extraction. Similar gains were attained by distillation for other standard biomedical knowledge extraction tasks such as gene-disease associations and protected health information, further illustrating the promise of this approach.
更多查看译文
关键词
biomedical knowledge extraction,large language models,drug
AI 理解论文
溯源树
样例
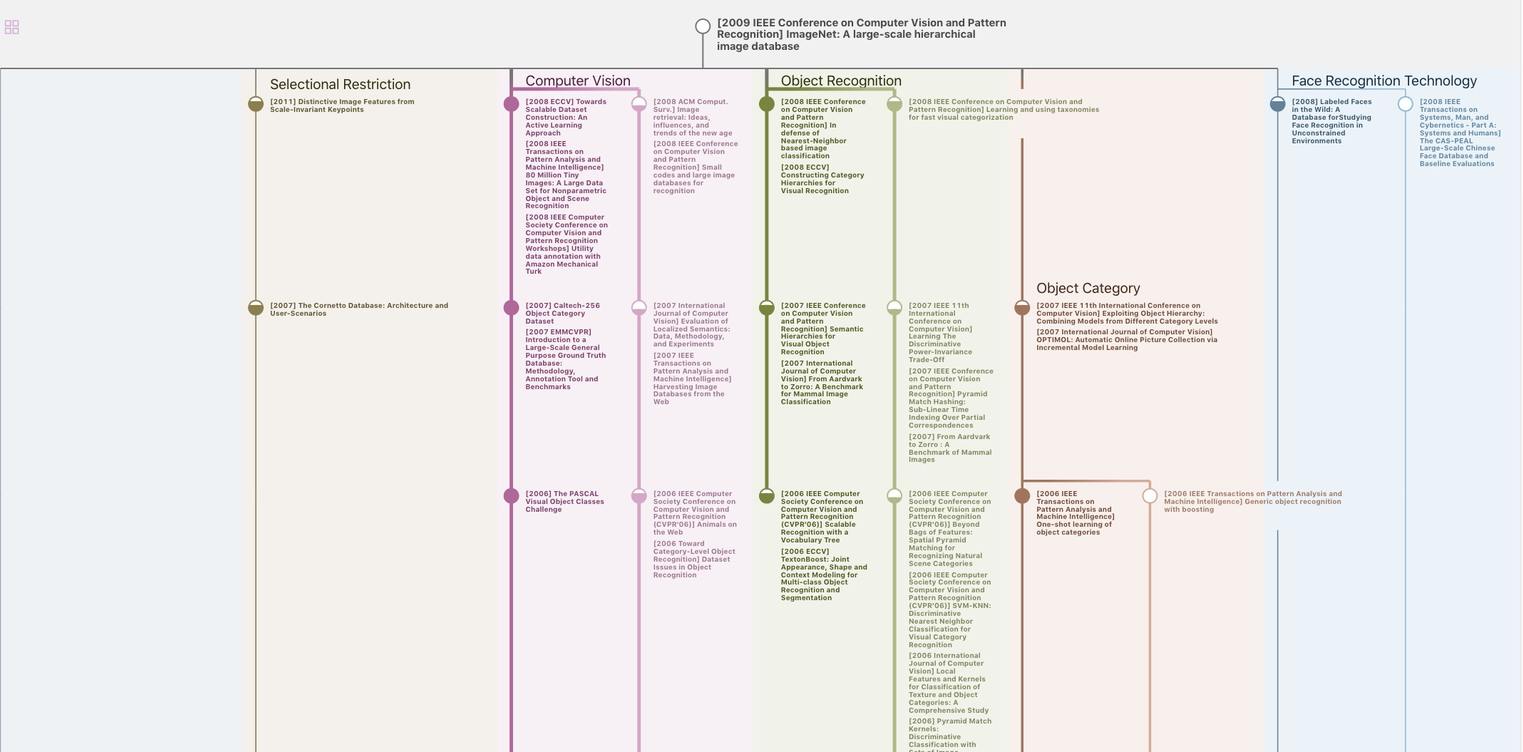
生成溯源树,研究论文发展脉络
Chat Paper
正在生成论文摘要