Radar Operation Mode Recognition via Multifeature Residual-and-Shrinkage ConvNet
IEEE Journal of Selected Topics in Applied Earth Observations and Remote Sensing(2023)
摘要
Radar operation mode recognition holds an increasingly critical place in electronic countermeasure as well as in remote sensing. However, the overlapped waveform parameters pose huge challenges to performing the radar operation mode recognition task in severe electromagnetic environments, particularly with large measurement errors or small sample lengths. By analyzing the timing patterns of a single radar pulse parameter and the correlation characteristics of multiple radar pulse parameters, this article first provides a revolutionary representation of the radar operating state by integrating interpulse parameter characteristics. Subsequently, a multifeature fused stream-level recognition framework with an attention mechanism, named residual-and-shrinkage ConvNet, is proposed to identify typical radar operating states. This tailored-made deep learning framework can effectively extract the timing and correlative features, which are conducive to pattern classification. The results of numerical experiments suggest that the proposed approach affords superior performance for the operation mode recognition task, even when the measurement error is large and the sample length is small, signifying the proposed method is strongly robust and time-efficient.
更多查看译文
关键词
Electronic countermeasure,multifeature correlation,neural networks,radar operation mode
AI 理解论文
溯源树
样例
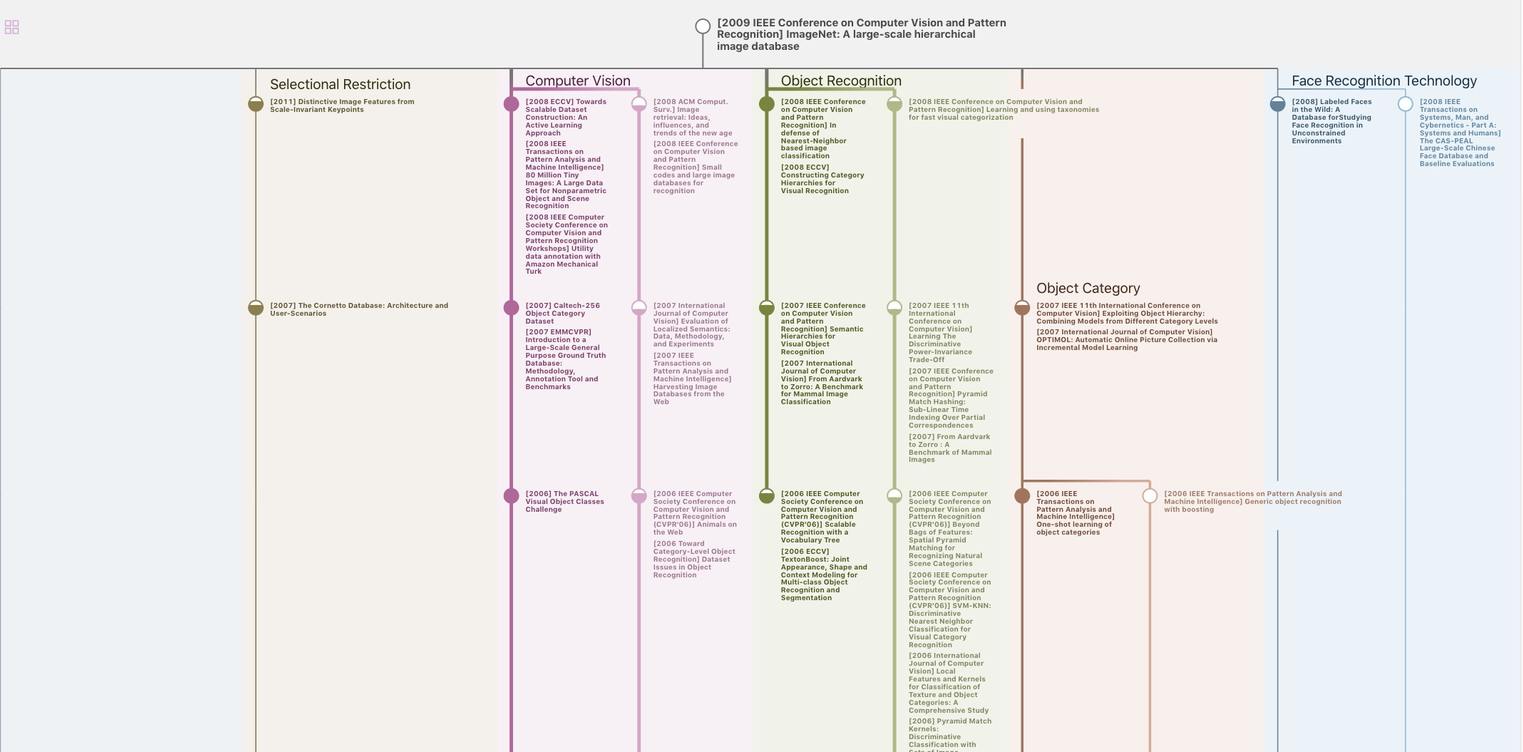
生成溯源树,研究论文发展脉络
Chat Paper
正在生成论文摘要