Unbiased Locally Private Estimator for Polynomials of Laplacian Variables.
KDD(2023)
摘要
This work presents a mechanism to debias polynomial functions computed from locally differentially private data. Local differential privacy is a widely used privacy notion where users add Laplacian noise to their information before submitting it to a central server. That, however, causes bias when we calculate non-linear functions based on those noisy information. Our proposed recursive algorithm debiases these functions, with a calculation time of O (rn log n), where r is the polynomial degree and n is the number of users. We evaluate our method on the problems of k-star counting and variance estimation, comparing results with state-of-the-art algorithms. The results show that our method not only eliminates bias, but also provides at least 100 times more accuracy than previous works.
更多查看译文
关键词
differential privacy,post-processing,unbiased estimator,k-stars,variance
AI 理解论文
溯源树
样例
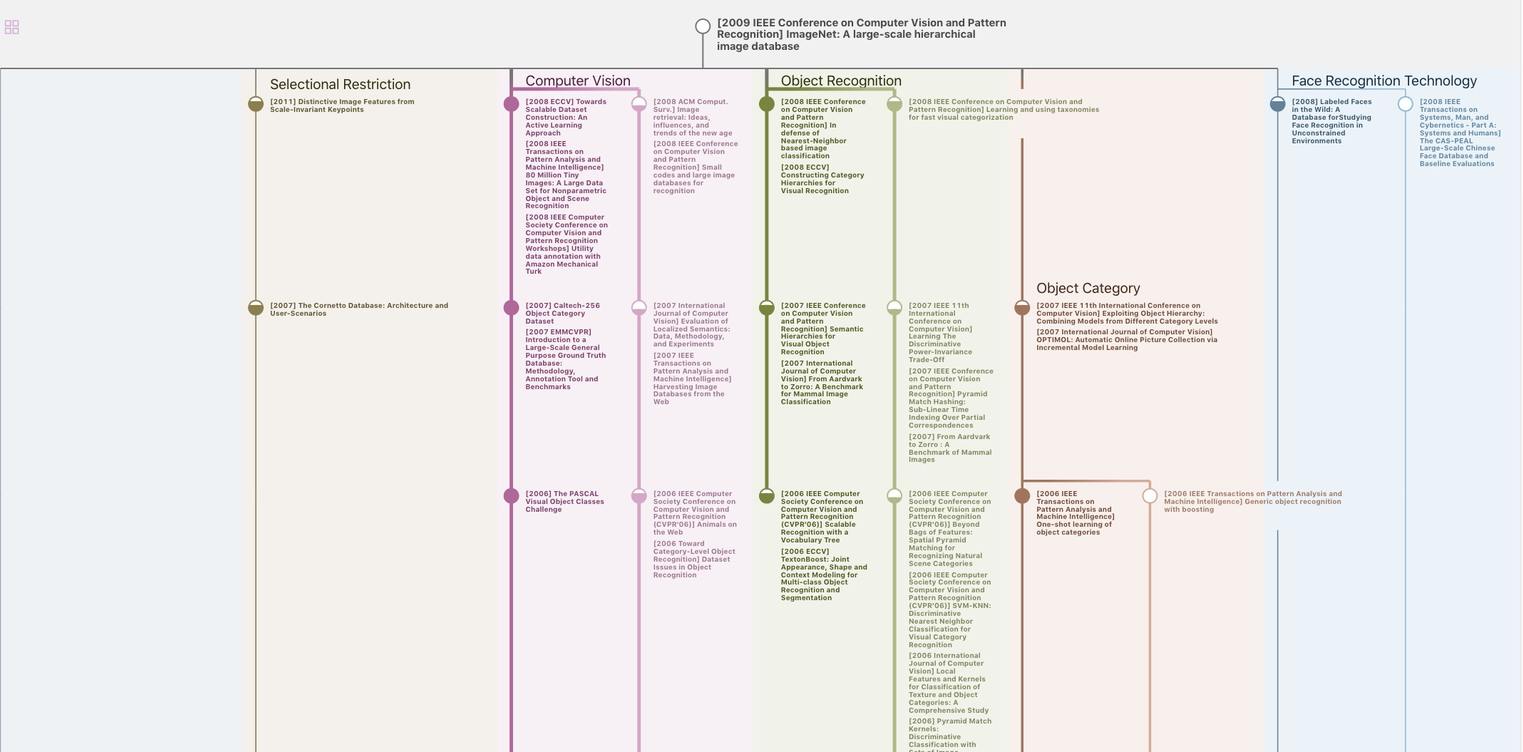
生成溯源树,研究论文发展脉络
Chat Paper
正在生成论文摘要