Diga: Guided Diffusion Model for Graph Recovery in Anti-Money Laundering.
KDD(2023)
摘要
With the upsurge of online banking, mobile payment, and virtual currency, new money-laundering crimes easily conceal in the enormous transaction volume. The traditional rule-based methods with large amounts of alerting thresholds are already incapable of handling the fast-changing transaction networks. Recently, the DL models represented by the graph neural networks (GNNs) show the potential to capture money-laundering modes with high accuracy. However, most related works are still far from practical deployment in the industry. Based on our practice at WeBank, there are three major challenges: Firstly, supervised learning is infeasible facing the extraordinarily large-scale but imbalanced data, with hundreds of millions of active accounts but only thousands of anomalies. Secondly, the real-world transactions form a sparse network with millions of isolated user groups, which overflows the expressive ability of current node-level GNNs. Thirdly, the explanation for each suspicious account is mandatory by the government for double check, which conflicts with the black-box nature of most DL models. Therefore, we proposed Diga, the first work to apply the diffusion probabilistic model to a graph anomaly detection problem with three novel techniques: the biased..-hop PageRank, the semi-supervised guided diffusion and the novel weight-sharing GNN layer. The effectiveness and efficiency of Diga are verified via intensive experiments on both industrial and public datasets.
更多查看译文
关键词
Anti-Money Laundering,Graph Anomaly Detection,Diffusion Model
AI 理解论文
溯源树
样例
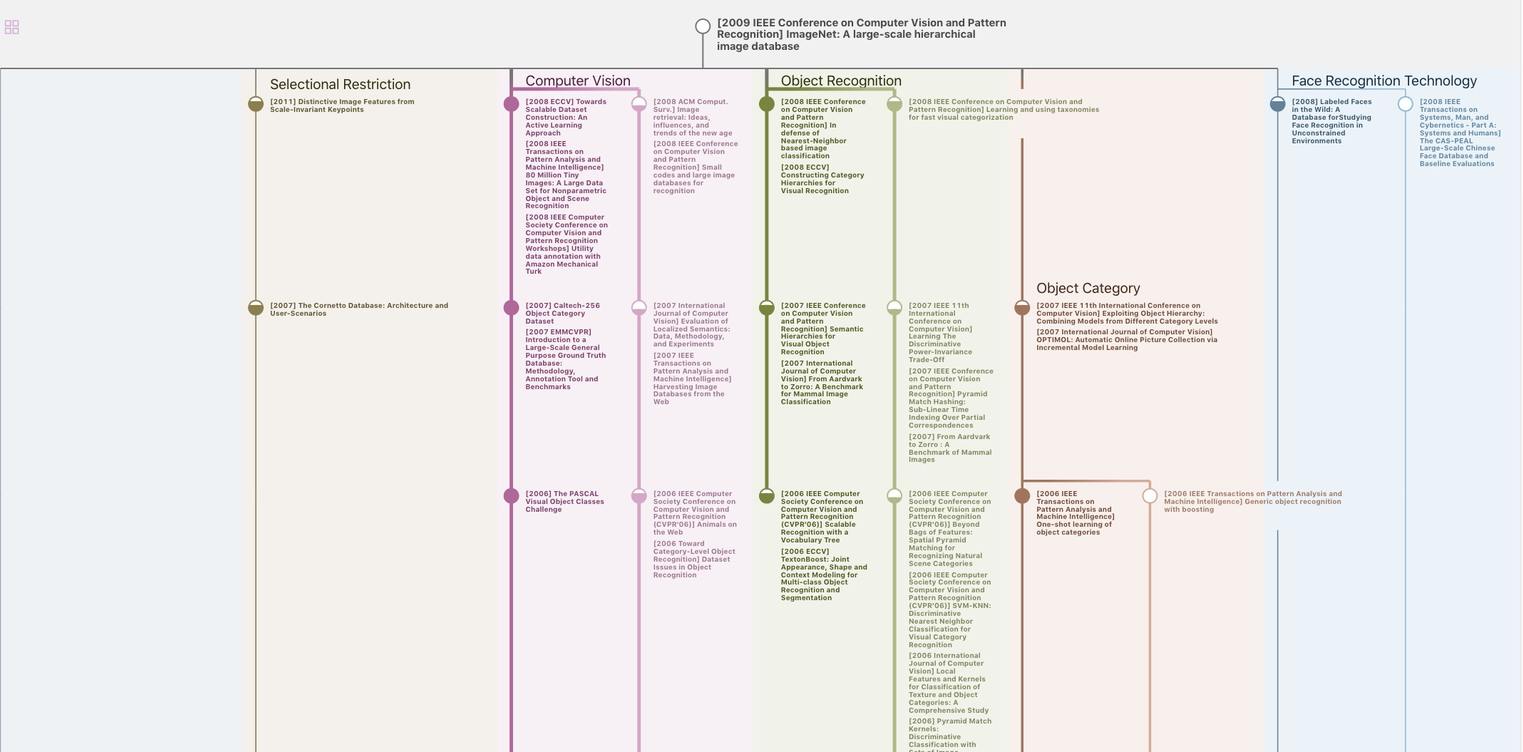
生成溯源树,研究论文发展脉络
Chat Paper
正在生成论文摘要