Empower Post-hoc Graph Explanations with Information Bottleneck: A Pre-training and Fine-tuning Perspective.
KDD(2023)
摘要
Researchers recently investigated to explain Graph Neural Networks (GNNs) on the access to a task-specific GNN, which may hinder their wide applications in practice. Specifically, task-specific explanation methods are incapable of explaining pretrained GNNs whose downstream tasks are usually inaccessible, not to mention giving explanations for the transferable knowledge in pretrained GNNs. Additionally, task-specific methods only consider target models' output in the label space, which are coarse-grained and insufficient to reflect the model's internal logic. To address these limitations, we consider a two-stage explanation strategy, i.e., explainers are first pretrained in a task-agnostic fashion in the representation space and then further fine-tuned in the task-specific label space and representation space jointly if downstream tasks are accessible. The two-stage explanation strategy endows post-hoc graph explanations with the applicability to pretrained GNNs where downstream tasks are inaccessible and the capacity to explain the transferable knowledge in the pretrained GNNs. Moreover, as the two-stage explanation strategy explains the GNNs in the representation space, the fine-grained information in the representation space also empowers the explanations. Furthermore, to achieve a trade-off between the fidelity and intelligibility of explanations, we propose an explanation framework based on the Information Bottleneck principle, named Explainable Graph Information Bottleneck (EGIB). EGIB subsumes the task-specific explanation and task-agnostic explanation into a unified framework. To optimize EGIB objective, we derive a tractable bound and adopt a simple yet effective explanation generation architecture. Based on the unified framework, we further theoretically prove that task-agnostic explanation is a relaxed sufficient condition of task-specific explanation, which indicates the transferability of task-agnostic explanations. Extensive experimental results demonstrate the effectiveness of our proposed explanation method.
更多查看译文
关键词
Graph Neural Networks,Explanation,Information Bottleneck
AI 理解论文
溯源树
样例
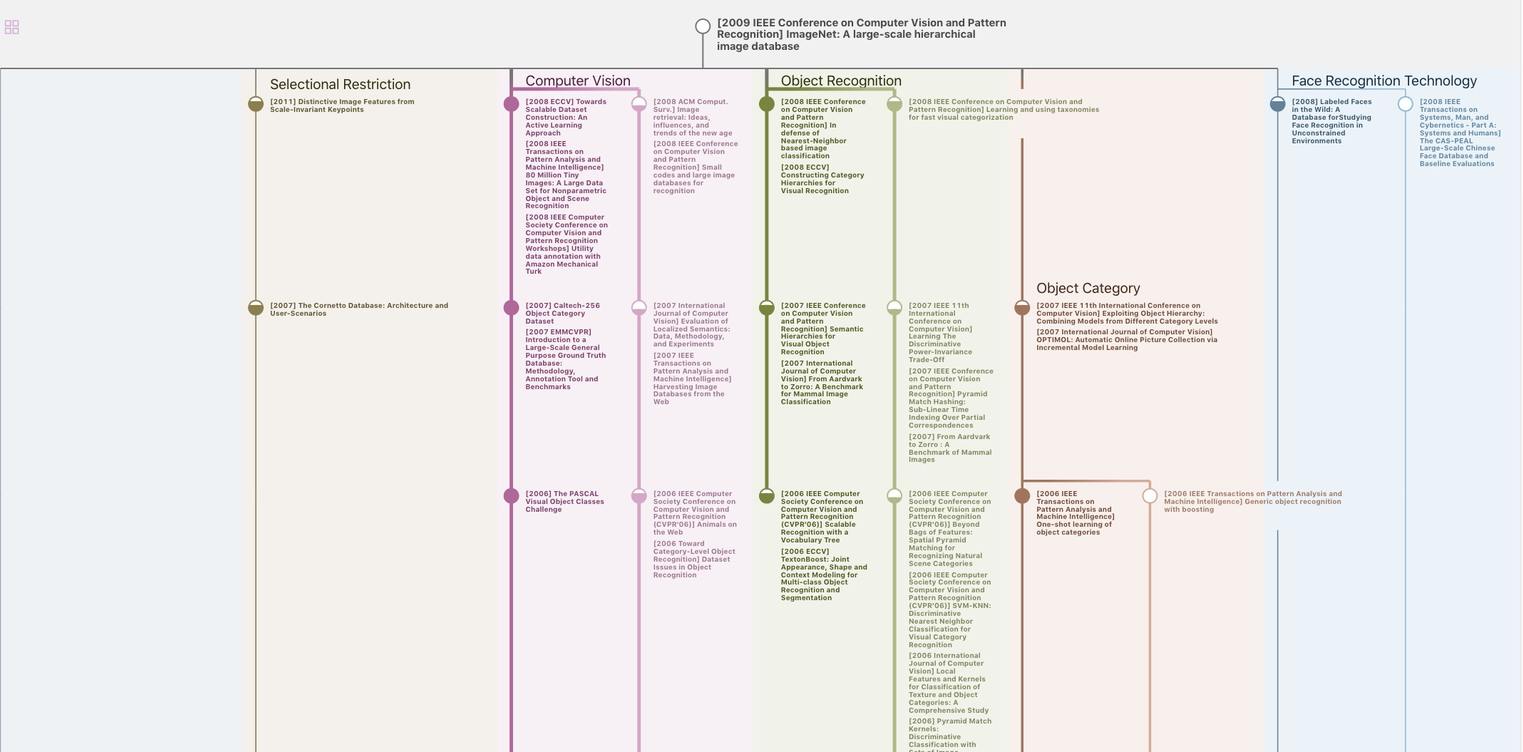
生成溯源树,研究论文发展脉络
Chat Paper
正在生成论文摘要