Merging multiple input descriptors and supervisors in a deep neural network for tractogram filtering
CoRR(2023)
摘要
One of the main issues of the current tractography methods is their high false-positive rate. Tractogram filtering is an option to remove false-positive streamlines from tractography data in a post-processing step. In this paper, we train a deep neural network for filtering tractography data in which every streamline of a tractogram is classified as {\em plausible, implausible}, or {\em inconclusive}. For this, we use four different tractogram filtering strategies as supervisors: TractQuerier, RecobundlesX, TractSeg, and an anatomy-inspired filter. Their outputs are combined to obtain the classification labels for the streamlines. We assessed the importance of different types of information along the streamlines for performing this classification task, including the coordinates of the streamlines, diffusion data, landmarks, T1-weighted information, and a brain parcellation. We found that the streamline coordinates are the most relevant followed by the diffusion data in this particular classification task.
更多查看译文
关键词
tractogram filtering,multiple input descriptors,supervisors
AI 理解论文
溯源树
样例
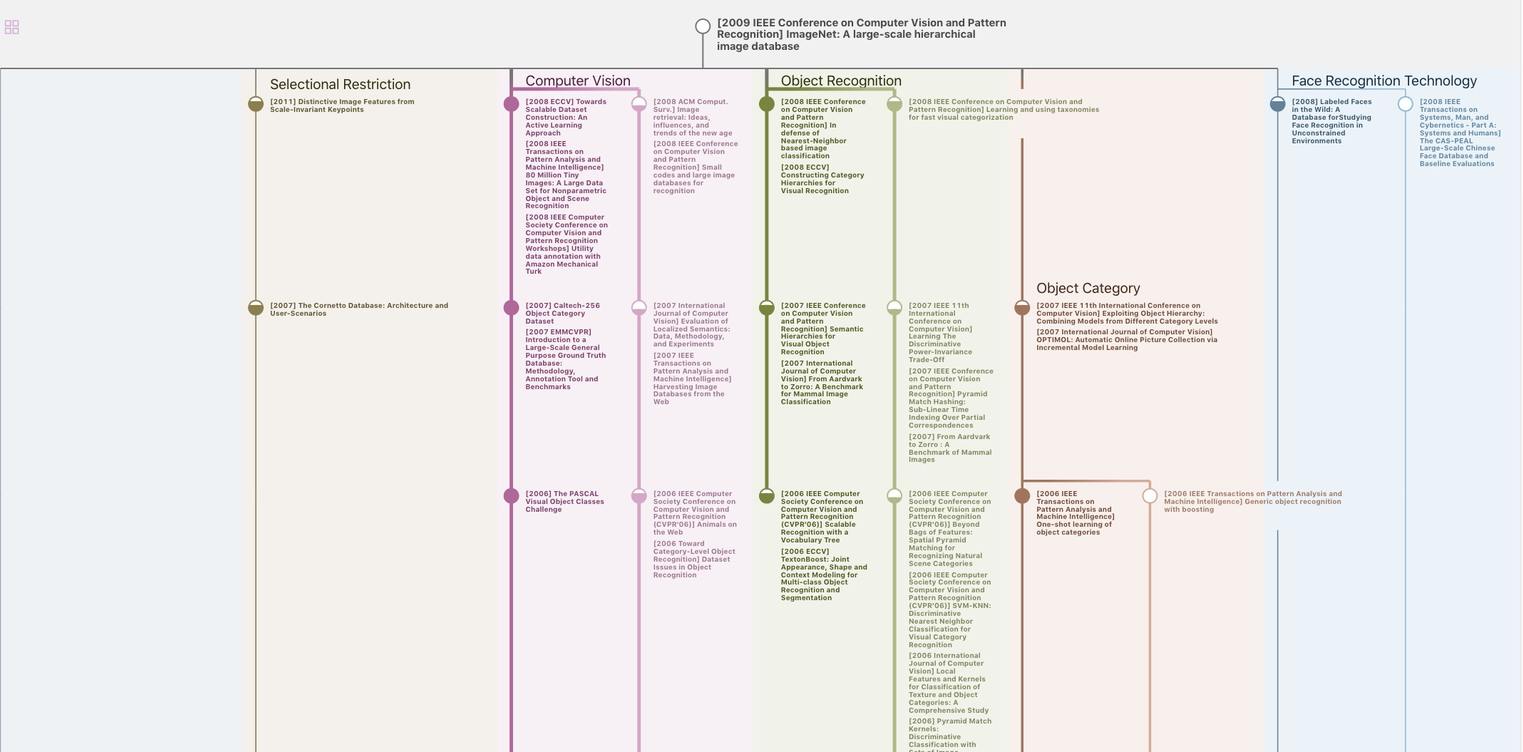
生成溯源树,研究论文发展脉络
Chat Paper
正在生成论文摘要