Compositional Generalization from First Principles
arXiv (Cornell University)(2023)
摘要
Leveraging the compositional nature of our world to expedite learning and facilitate generalization is a hallmark of human perception. In machine learning, on the other hand, achieving compositional generalization has proven to be an elusive goal, even for models with explicit compositional priors. To get a better handle on compositional generalization, we here approach it from the bottom up: Inspired by identifiable representation learning, we investigate compositionality as a property of the data-generating process rather than the data itself. This reformulation enables us to derive mild conditions on only the support of the training distribution and the model architecture, which are sufficient for compositional generalization. We further demonstrate how our theoretical framework applies to real-world scenarios and validate our findings empirically. Our results set the stage for a principled theoretical study of compositional generalization.
更多查看译文
关键词
generalization,principles
AI 理解论文
溯源树
样例
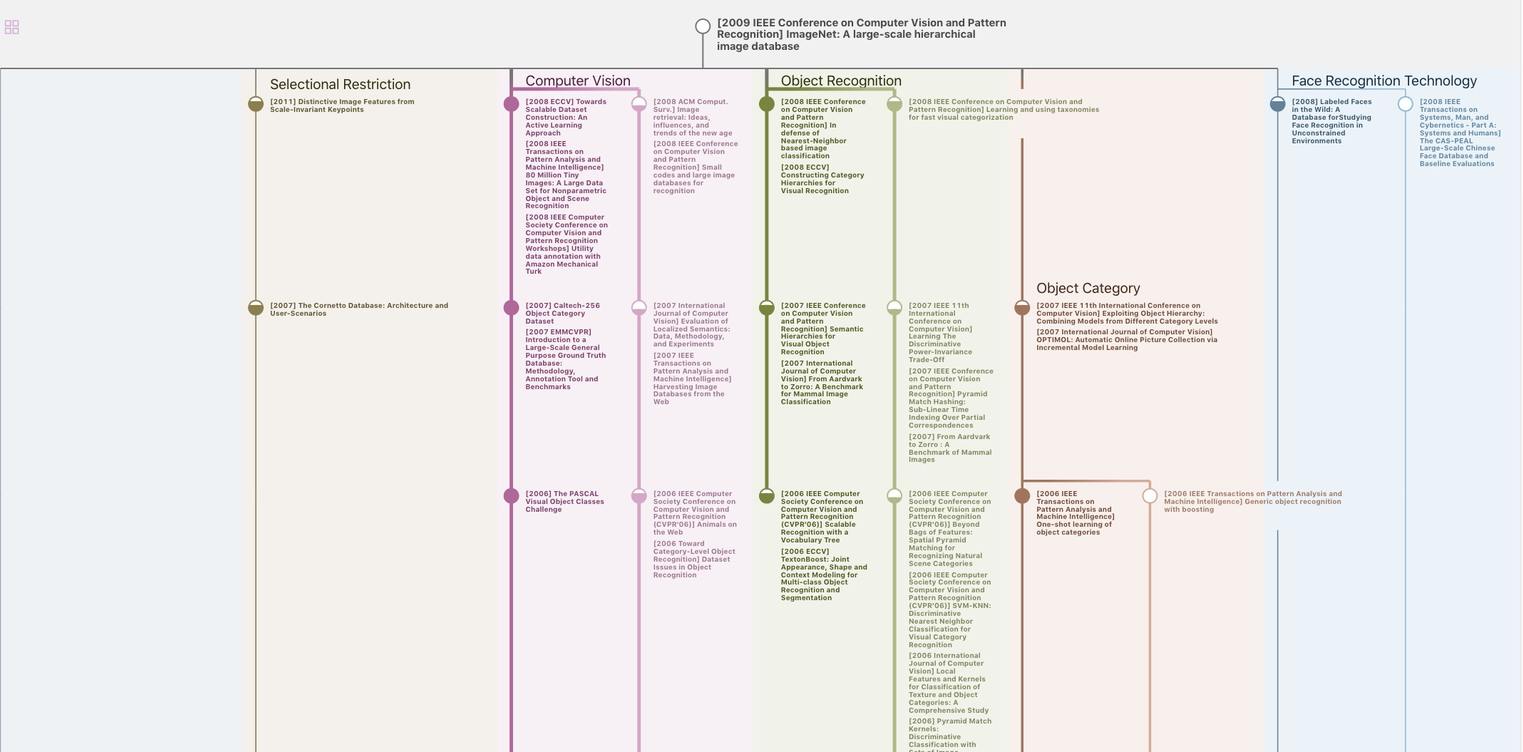
生成溯源树,研究论文发展脉络
Chat Paper
正在生成论文摘要