Deep Learning Integration of Chest Computed Tomography Imaging and Gene Expression Identifies Novel Aspects of COPD
CHRONIC OBSTRUCTIVE PULMONARY DISEASES-JOURNAL OF THE COPD FOUNDATION(2023)
摘要
Rationale: Chronic obstructive pulmonary disease (COPD) is characterized by pathologic changes in the airways, lung parenchyma, and persistent inflammation, but the links between lung structural changes and blood transcriptome patterns have not been fully described. Objectives: The objective of this study was to identify novel relationships between lung structural changes measured by chest computed tomography (CT) and blood transcriptome patterns measured by blood RNA sequencing (RNA-seq). Methods: CT scan images and blood RNA-seq gene expression from 1223 participants in the COPD Genetic Epidemiology (COPDGene (R)) study were jointly analyzed using deep learning to identify shared aspects of inflammation and lung structural changes that we labeled image-expression axes (IEAs). We related IEAs to COPD-related measurements and prospective health outcomes through regression and Cox proportional hazards models and tested them for biological pathway enrichment. Results: We identified 2 distinct IEAs: IEAemph which captures an emphysema-predominant process with a strong positive correlation to CT emphysema and a negative correlation to forced expiratory volume in 1 second and body mass index (BMI); and IEAairway which captures an airway-predominant process with a positive correlation to BMI and airway wall thickness and a negative correlation to emphysema. Pathway enrichment analysis identified 29 and 13 pathways significantly associated with IEAemph and IEAairway, respectively (adjusted p<0.001). Conclusions: Integration of CT scans and blood RNA-seq data identified 2 IEAs that capture distinct inflammatory processes associated with emphysema and airway-predominant COPD.
更多查看译文
关键词
machine learning,emphysema,genomics
AI 理解论文
溯源树
样例
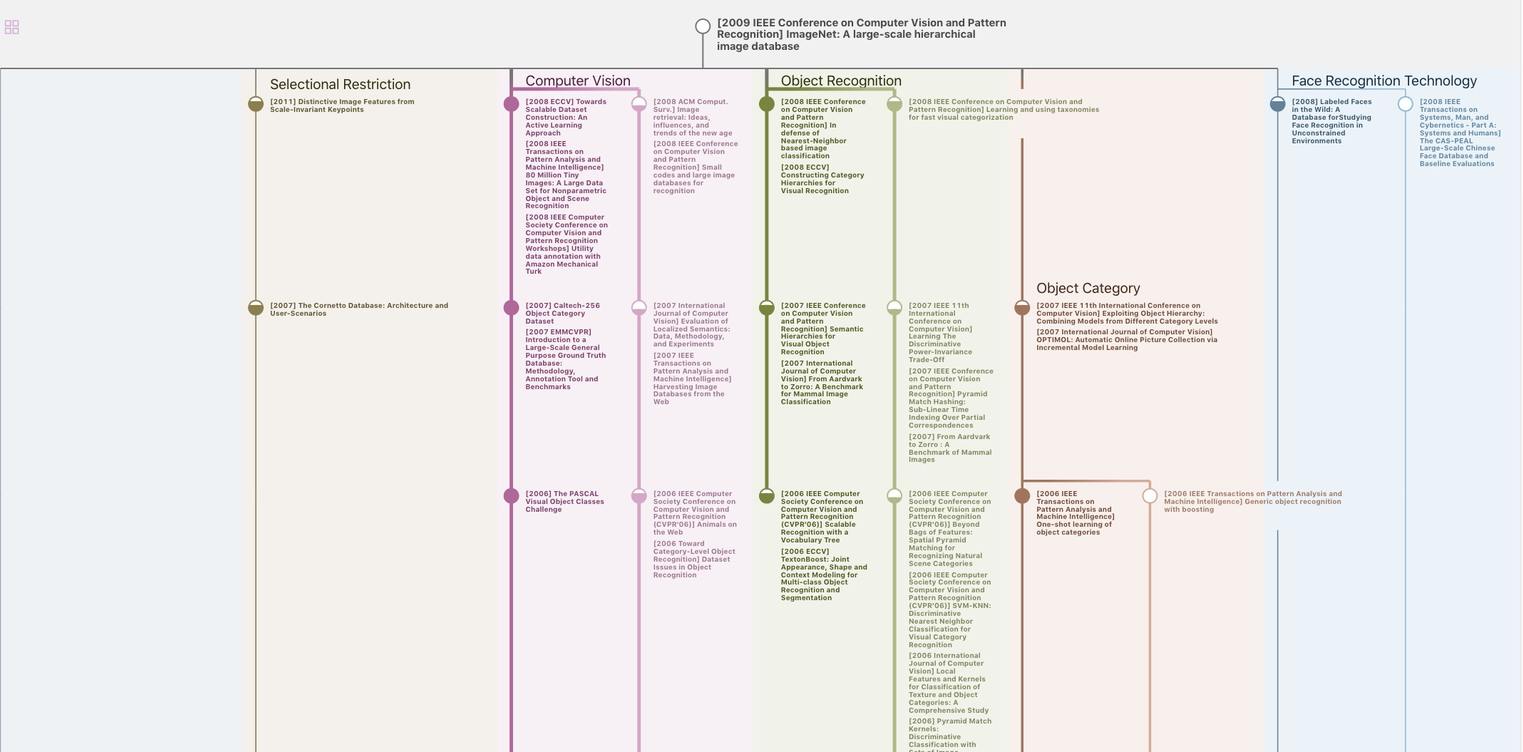
生成溯源树,研究论文发展脉络
Chat Paper
正在生成论文摘要