Towards Causality-Aware Inferring: A Sequential Discriminative Approach for Medical Diagnosis.
IEEE transactions on pattern analysis and machine intelligence(2023)
摘要
Medical diagnosis assistant (MDA) aims to build an interactive diagnostic agent to sequentially inquire about symptoms for discriminating diseases. However, since the dialogue records for building a patient simulator are collected passively, the collected records might be deteriorated by some task-unrelated biases, such as the preference of the collectors. These biases might hinder the diagnostic agent to capture transportable knowledge from the simulator. This work identifies and resolves two representative non-causal biases, i.e., (i) default-answer bias and (ii) distributional inquiry bias. Specifically, Bias (i) originates from the patient simulator which tries to answer the unrecorded inquiries with some biased default answers. To eliminate this bias and improve upon a well-known causal inference technique, i.e., propensity score matching, we propose a novel propensity latent matching in building a patient simulator to effectively answer unrecorded inquiries; Bias (ii) inherently comes along with the passively collected data that the agent might learn by remembering what to inquire within the training data while not able to generalize to the out-of-distribution cases. To this end, we propose a progressive assurance agent, which includes the dual processes accounting for symptom inquiry and disease diagnosis respectively. The diagnosis process pictures the patient mentally and probabilistically by intervention to eliminate the effect of the inquiry behavior. And the inquiry process is driven by the diagnosis process to inquire about symptoms to enhance the diagnostic confidence which alters as the patient distribution changes. In this cooperative manner, our proposed agent can improve upon the out-of-distribution generalization significantly. Extensive experiments demonstrate that our framework achieves new state-of-the-art performance and possesses the advantage of transportability. The source code is available at https://github.com/junfanlin/CAMAD.
更多查看译文
关键词
Diseases, Training, Medical diagnosis, Computational modeling, Training data, Estimation, Buildings, Causal inference, reinforcement learning, decision making, medical diagnosis assistant
AI 理解论文
溯源树
样例
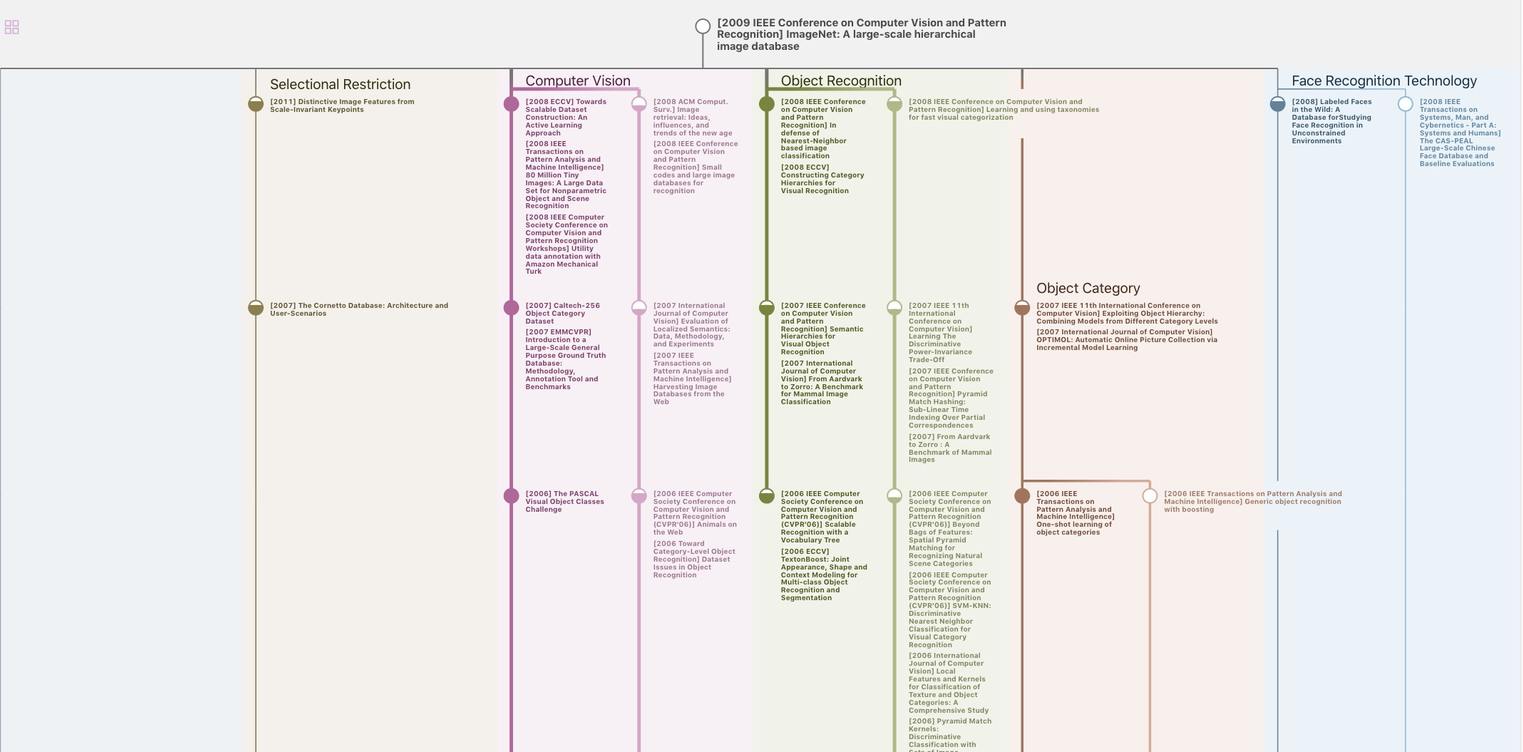
生成溯源树,研究论文发展脉络
Chat Paper
正在生成论文摘要