Comparing methods for drug–gene interaction prediction on the biomedical literature knowledge graph: performance versus explainability
BMC bioinformatics(2023)
摘要
This paper applies different link prediction methods on a knowledge graph generated from biomedical literature, with the aim to compare their ability to identify unknown drug-gene interactions and explain their predictions. Identifying novel drug–target interactions is a crucial step in drug discovery and repurposing. One approach to this problem is to predict missing links between drug and gene nodes, in a graph that contains relevant biomedical knowledge. Such a knowledge graph can be extracted from biomedical literature, using text mining tools. In this work, we compare state-of-the-art graph embedding approaches and contextual path analysis on the interaction prediction task. The comparison reveals a trade-off between predictive accuracy and explainability of predictions. Focusing on explainability, we train a decision tree on model predictions and show how it can aid the understanding of the prediction process. We further test the methods on a drug repurposing task and validate the predicted interactions against external databases, with very encouraging results.
更多查看译文
关键词
biomedical literature knowledge graph,prediction,drug-gene
AI 理解论文
溯源树
样例
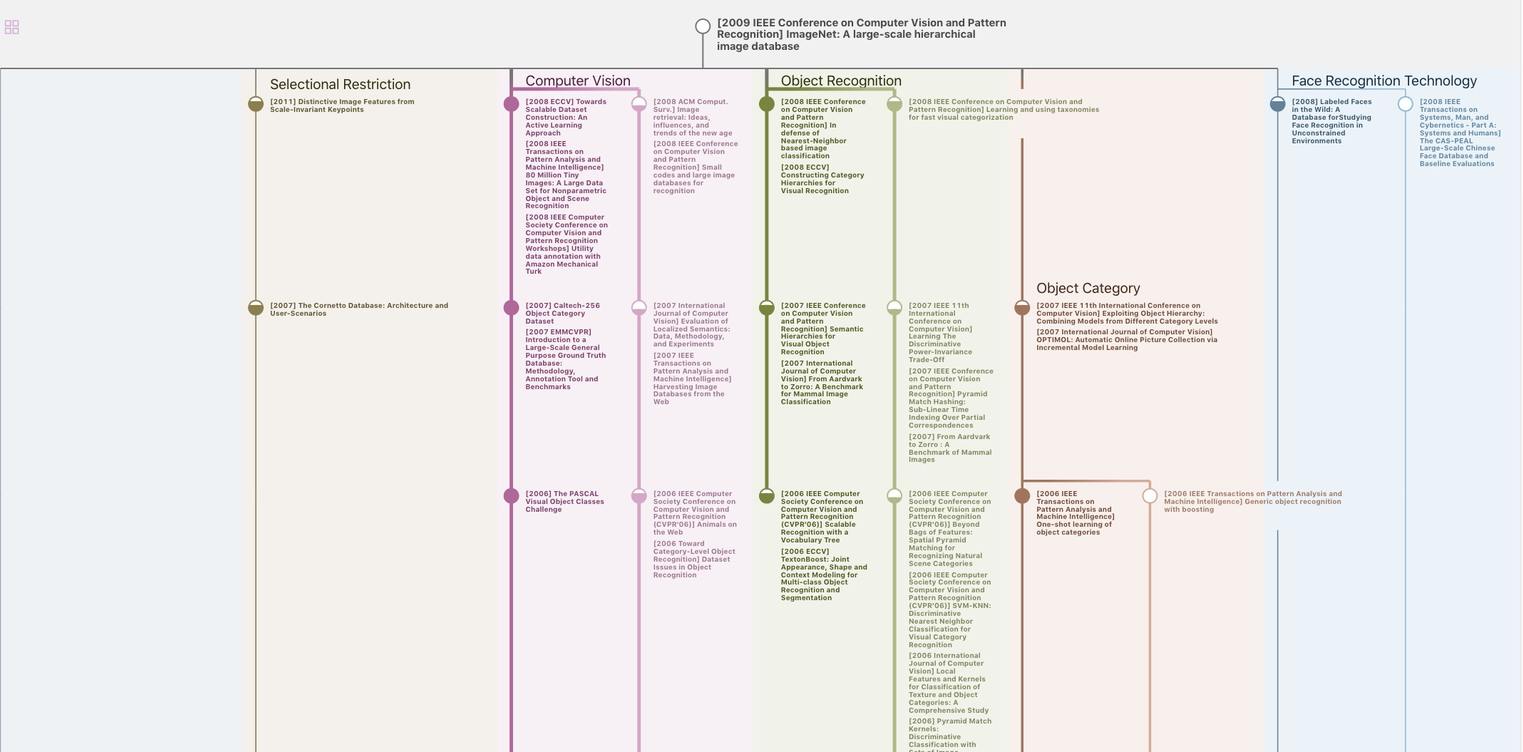
生成溯源树,研究论文发展脉络
Chat Paper
正在生成论文摘要