Advanced characterization-informed machine learning framework and quantitative insight to irradiated annular U-10Zr metallic fuels
Scientific reports(2023)
摘要
U-10Zr Metal fuel is a promising nuclear fuel candidate for next-generation sodium-cooled fast spectrum reactors. Since the Experimental Breeder Reactor-II in the late 1960s, researchers accumulated a considerable amount of experience and knowledge on fuel performance at the engineering scale. However, a mechanistic understanding of fuel microstructure evolution and property degradation during in-reactor irradiation is still missing due to a lack of appropriate tools for rapid fuel microstructure assessment and property prediction based on post irradiation examination. This paper proposed a machine learning enabled workflow, coupled with domain knowledge and large dataset collected from advanced post-irradiation examination microscopies, to provide rapid and quantified assessments of the microstructure in two reactor irradiated prototypical annular metal fuels. Specifically, this paper revealed the distribution of Zr-bearing secondary phases and constitutional redistribution across different radial locations. Additionally, the ratios of seven different microstructures at various locations along the temperature gradient were quantified. Moreover, the distributions of fission gas pores on two types of U-10Zr annular fuels were quantitatively compared.
更多查看译文
关键词
metallic fuels,machine learning,characterization-informed
AI 理解论文
溯源树
样例
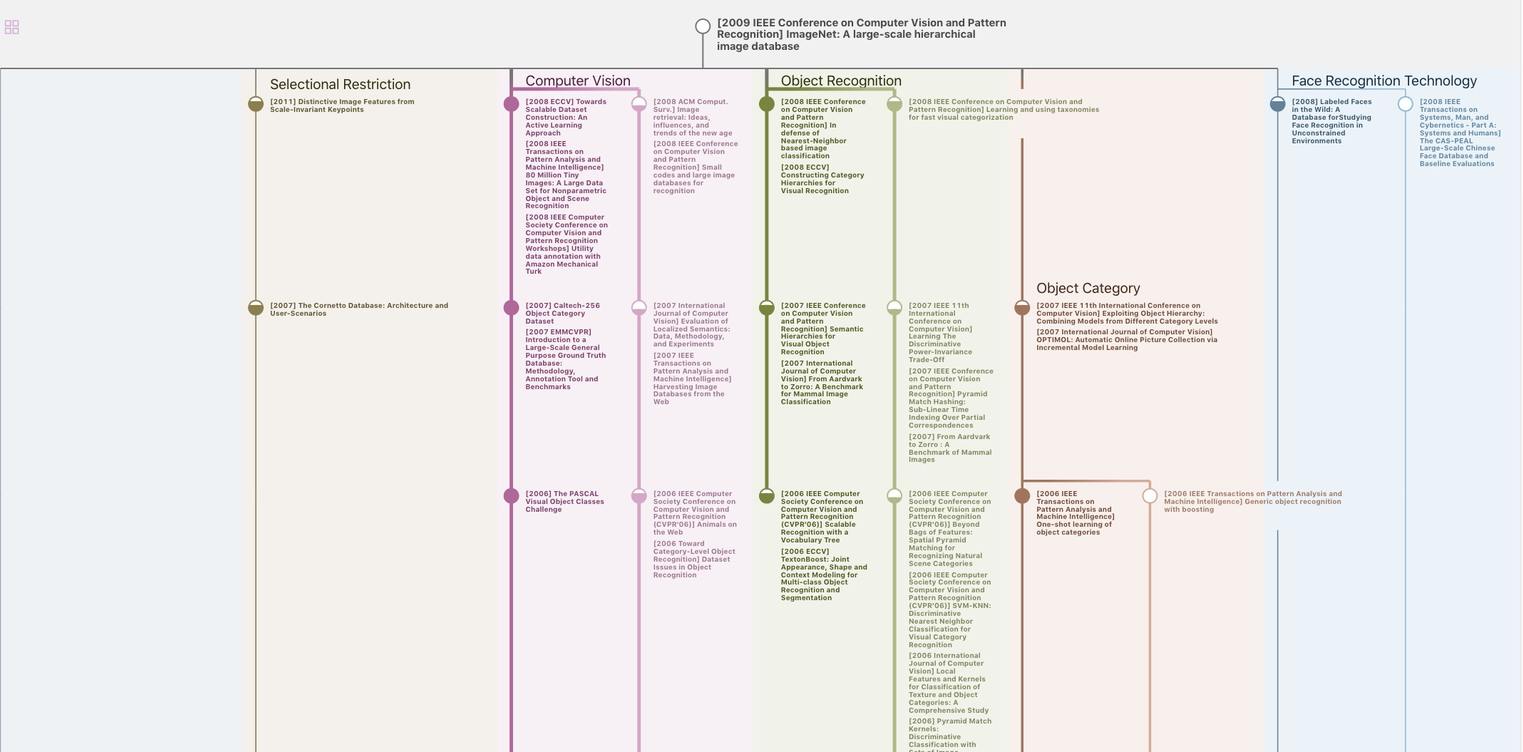
生成溯源树,研究论文发展脉络
Chat Paper
正在生成论文摘要