ADis-QSAR: a machine learning model based on biological activity differences of compounds
Journal of computer-aided molecular design(2023)
摘要
Drug candidates identified by the pharmaceutical industry typically have unique structural characteristics to ensure they interact strongly and specifically with their biological targets. Identifying these characteristics is a key challenge for developing new drugs, and quantitative structure-activity relationship (QSAR) analysis has generally been used to perform this task. QSAR models with good predictive power improve the cost and time efficiencies invested in compound development. Generating these good models depends on how well differences between “active” and “inactive” compound groups can be conveyed to the model to be learned. Efforts to solve this difference issue have been made, including generating a “molecular descriptor” that compressively expresses the structural characteristics of compounds. From the same perspective, we succeeded in developing the Activity Differences-Quantitative Structure-Activity Relationship (ADis-QSAR) model by generating molecular descriptors that more explicitly convey features of the group through a pair system that performs direct connections between active and inactive groups. We used popular machine learning algorithms, such as Support Vector Machine, Random Forest, XGBoost and Multi-Layer Perceptron for model learning and evaluated the model using scores such as accuracy, area under curve, precision and specificity. The results showed that the Support Vector Machine performed better than the others. Notably, the ADis-QSAR model showed significant improvements in meaningful scores such as precision and specificity compared to the baseline model, even in datasets with dissimilar chemical spaces. This model reduces the risk of selecting false positive compounds, improving the efficiency of drug development.
更多查看译文
关键词
compounds,biological activity differences,machine learning model,machine learning,adis-qsar
AI 理解论文
溯源树
样例
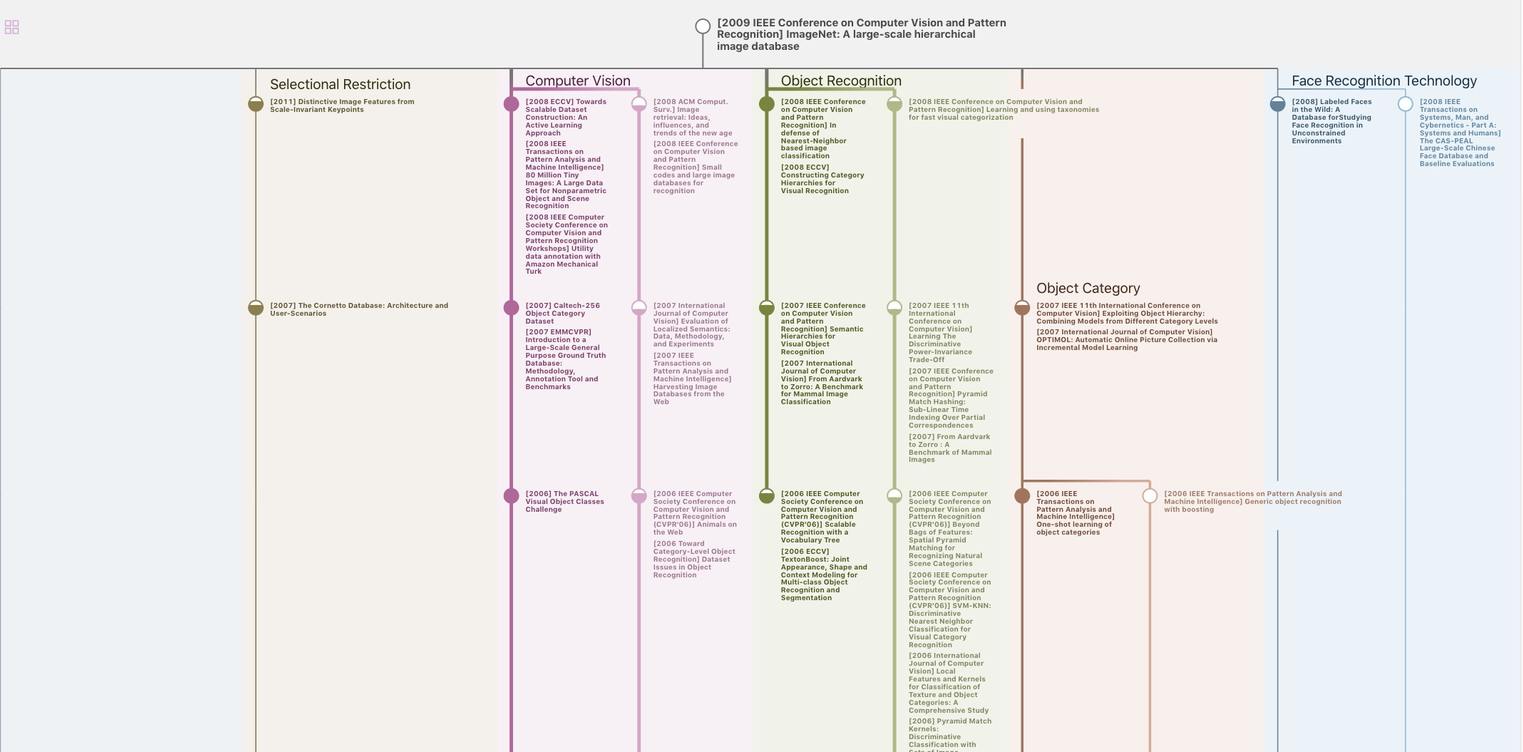
生成溯源树,研究论文发展脉络
Chat Paper
正在生成论文摘要