A Novel Feature Engineering Method Based on Latent Representation Learning for Radiomics: Application in NSCLC Subtype Classification
IEEE JOURNAL OF BIOMEDICAL AND HEALTH INFORMATICS(2024)
摘要
Radiomics refers to the high-throughput extraction of quantitative features from medical images, and is widely used to construct machine learning models for the prediction of clinical outcomes, while feature engineering is the most important work in radiomics. However, current feature engineering methods fail to fully and effectively utilize the heterogeneity of features when dealing with different kinds of radiomics features. In this work, latent representation learning is first presented as a novel feature engineering approach to reconstruct a set of latent space features from original shape, intensity and texture features. This proposed method projects features into a subspace called latent space, in which the latent space features are obtained by minimizing a unique hybrid loss function including a clustering-like loss and a reconstruction loss. The former one ensures the separability among each class while the latter one narrows the gap between the original features and latent space features. Experiments were performed on a multi-center non-small cell lung cancer (NSCLC) subtype classification dataset from 8 international open databases. Results showed that compared with four traditional feature engineering methods (baseline, PCA, Lasso and L2,1-norm minimization), latent representation learning could significantly improve the classification performance of various machine learning classifiers on the independent test set (all p < 0.001). Further on two additional test sets, latent representation learning also showed a significant improvement in generalization performance. Our research shows that latent representation learning is a more effective feature engineering method, which has the potential to be used as a general technology in a wide range of radiomics researches.
更多查看译文
关键词
Latent representation learning,radiomics,feature engineering,NSCLC subtype classification
AI 理解论文
溯源树
样例
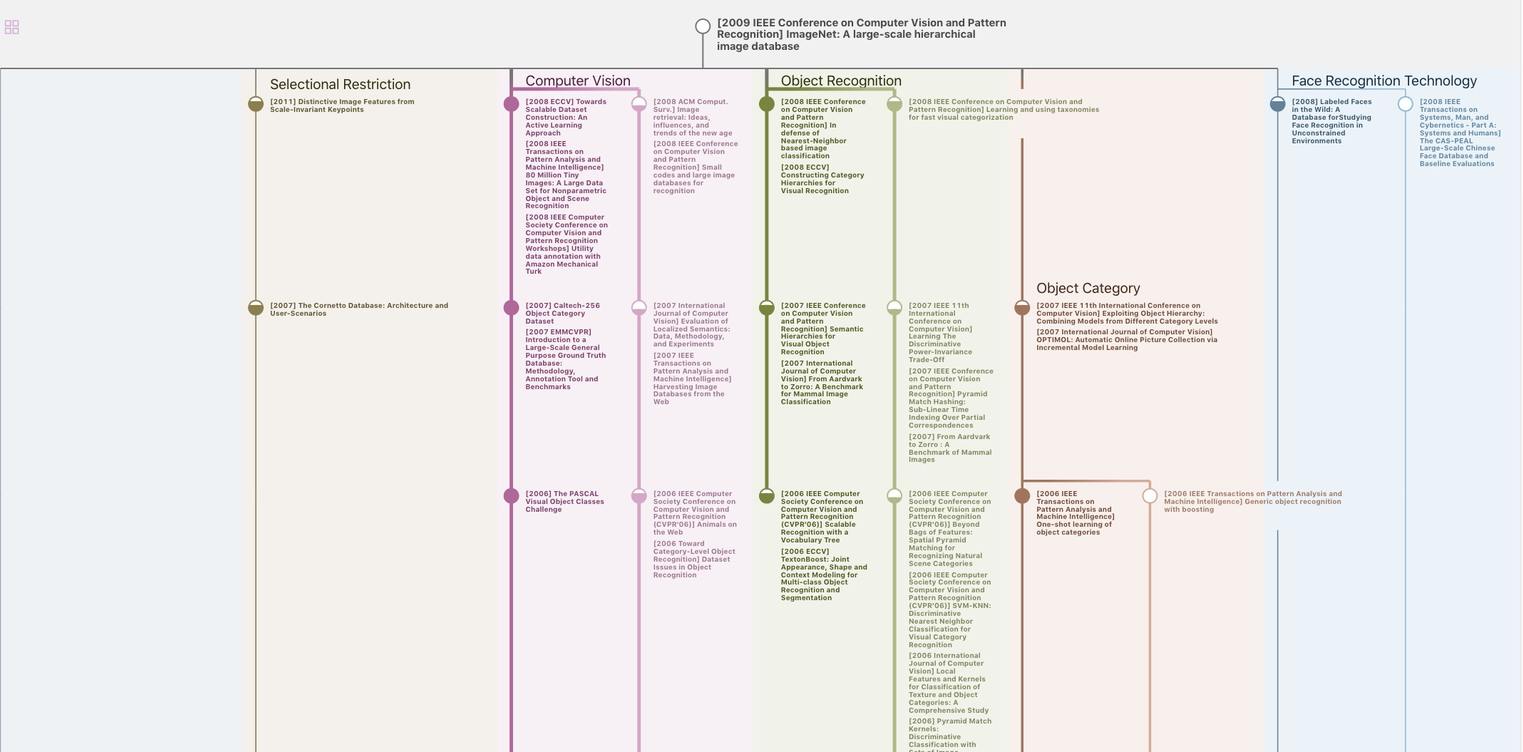
生成溯源树,研究论文发展脉络
Chat Paper
正在生成论文摘要