Performance comparison between multi-center histopathology datasets of a weakly-supervised deep learning model for pancreatic ductal adenocarcinoma detection
Cancer imaging : the official publication of the International Cancer Imaging Society(2023)
摘要
Background Pancreatic ductal carcinoma patients have a really poor prognosis given its difficult early detection and the lack of early symptoms. Digital pathology is routinely used by pathologists to diagnose the disease. However, visually inspecting the tissue is a time-consuming task, which slows down the diagnostic procedure. With the advances occurred in the area of artificial intelligence, specifically with deep learning models, and the growing availability of public histology data, clinical decision support systems are being created. However, the generalization capabilities of these systems are not always tested, nor the integration of publicly available datasets for pancreatic ductal carcinoma detection (PDAC). Methods In this work, we explored the performace of two weakly-supervised deep learning models using the two more widely available datasets with pancreatic ductal carcinoma histology images, The Cancer Genome Atlas Project (TCGA) and the Clinical Proteomic Tumor Analysis Consortium (CPTAC). In order to have sufficient training data, the TCGA dataset was integrated with the Genotype-Tissue Expression (GTEx) project dataset, which contains healthy pancreatic samples. Results We showed how the model trained on CPTAC generalizes better than the one trained on the integrated dataset, obtaining an inter-dataset accuracy of 90 . 62% ± 2 . 32 and an outer-dataset accuracy of 92 . 17% when evaluated on TCGA + GTEx. Furthermore, we tested the performance on another dataset formed by tissue micro-arrays, obtaining an accuracy of 98 . 59%. We showed how the features learned in an integrated dataset do not differentiate between the classes, but between the datasets, noticing that a stronger normalization might be needed when creating clinical decision support systems with datasets obtained from different sources. To mitigate this effect, we proposed to train on the three available datasets, improving the detection performance and generalization capabilities of a model trained only on TCGA + GTEx and achieving a similar performance to the model trained only on CPTAC. Conclusions The integration of datasets where both classes are present can mitigate the batch effect present when integrating datasets, improving the classification performance, and accurately detecting PDAC across different datasets.
更多查看译文
关键词
Digital pathology,Deep learning,Weakly-supervised classification
AI 理解论文
溯源树
样例
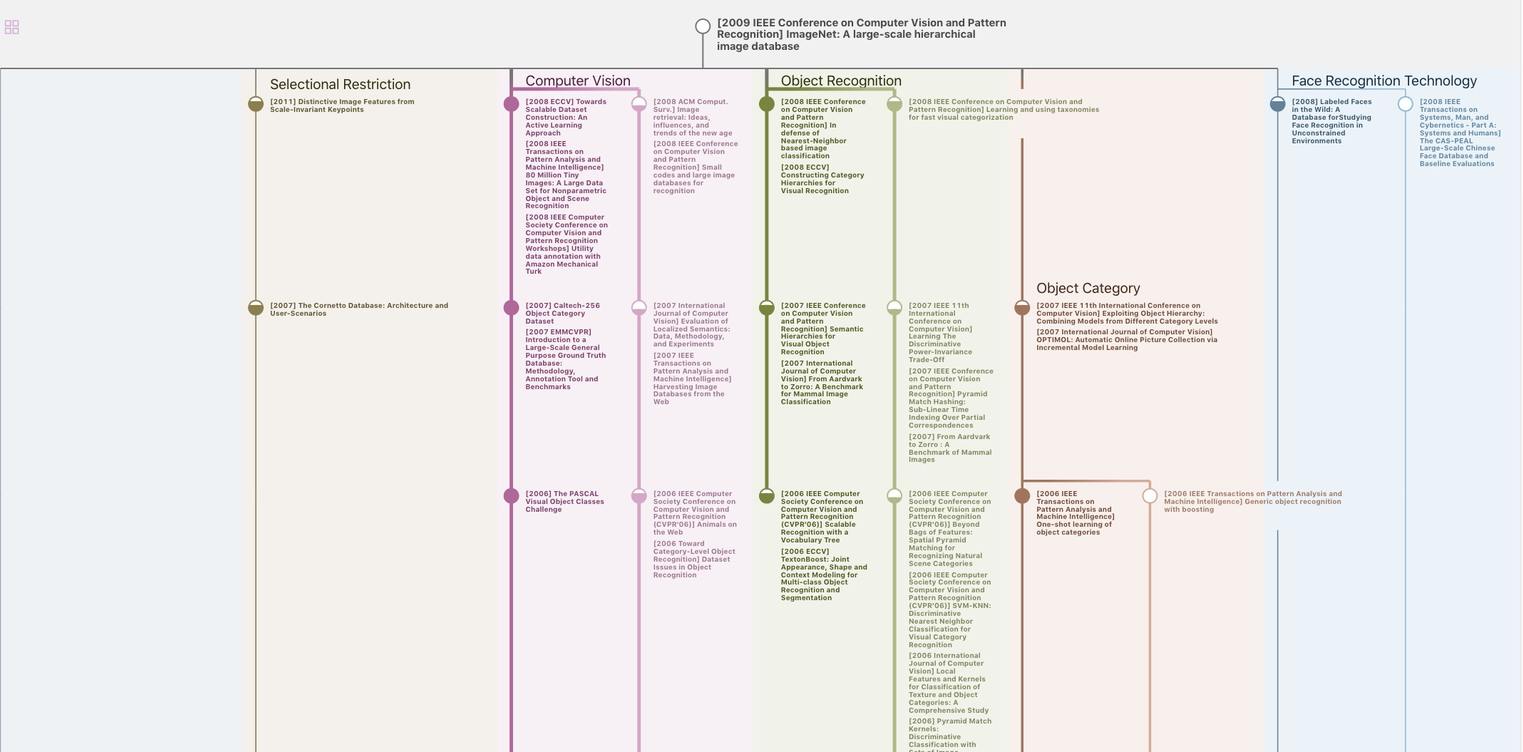
生成溯源树,研究论文发展脉络
Chat Paper
正在生成论文摘要