scASGC: An adaptive simplified graph convolution model for clustering single-cell RNA-seq data.
Computers in biology and medicine(2023)
摘要
Single-cell RNA sequencing (scRNA-seq) is now a successful technique for identifying cellular heterogeneity, revealing novel cell subpopulations, and forecasting developmental trajectories. A crucial component of the processing of scRNA-seq data is the precise identification of cell subpopulations. Although many unsupervised clustering methods have been developed to cluster cell subpopulations, the performance of these methods is vulnerable to dropouts and high dimensionality. In addition, most existing methods are time-consuming and fail to adequately account for potential associations between cells. In the manuscript, we present an unsupervised clustering method based on an adaptive simplified graph convolution model called scASGC. The proposed method builds plausible cell graphs, aggregates neighbor information using a simplified graph convolution model, and adaptively determines the most optimal number of convolution layers for various graphs. Experiments on 12 public datasets show that scASGC outperforms both classical and state-of-the-art clustering methods. In addition, in a study of mouse intestinal muscle containing 15,983 cells, we identified distinct marker genes based on the clustering results of scASGC. The source code of scASGC is available at https://github.com/ZzzOctopus/scASGC.
更多查看译文
关键词
ScRNA-seq,Clustering,Bioinformatics,Graph convolution,Computational biology,Machine learning
AI 理解论文
溯源树
样例
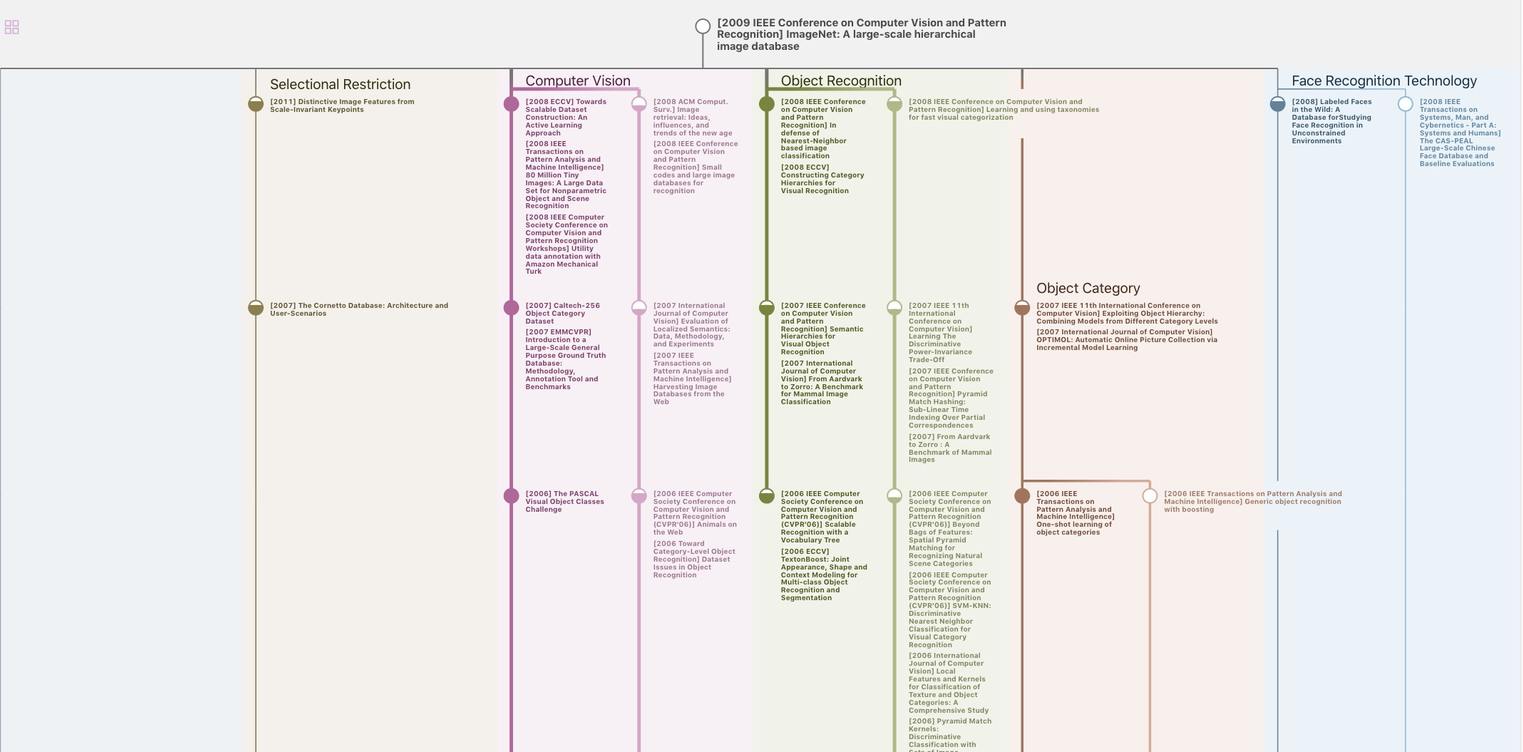
生成溯源树,研究论文发展脉络
Chat Paper
正在生成论文摘要