Hypothesis Scoring for Confidence-Aware Blood Pressure Estimation with Particle Filters.
IEEE journal of biomedical and health informatics(2023)
摘要
We propose our Confidence-Aware Particle Filter (CAPF) framework that analyzes a series of estimated changes in blood pressure (BP) to provide several true state hypotheses for a given instance. Particularly, our novel confidence-awareness mechanism assigns likelihood scores to each hypothesis in an effort to discard potentially erroneous measurements - based on the agreement amongst a series of estimated changes and the physiological plausibility when considering DBP/SBP pairs. The particle filter formulation (or sequential Monte Carlo method) can jointly consider the hypotheses and their probabilities over time to provide a stable trend of estimated BP measurements. In this study, we evaluate BP trend estimation from an emerging bio-impedance (Bio-Z) prototype wearable modality although it is applicable to all types of physiological modalities. Each subject in the evaluation cohort underwent a hand-gripper exercise, a cold pressor test, and a recovery state to increase the variation to the captured BP ranges. Experiments show that CAPF yields superior continuous pulse pressure (PP), diastolic blood pressure (DBP), and systolic blood pressure (SBP) estimation performance compared to ten baseline approaches. Furthermore, CAPF performs on track to comply with AAMI and BHS standards for achieving a performance classification of Grade A, with mean error accuracies of -0.16 ± 3.75 mmHg for PP (r = 0.81), 0.42 ± 4.39 mmHg for DBP (r = 0.92), and -0.09 ± 6.51 mmHg for SBP (r = 0.92) from more than test 3500 data points.
更多查看译文
关键词
Blood pressure,blood pressure variability,particle filtering,confidence-aware,ensemble modeling
AI 理解论文
溯源树
样例
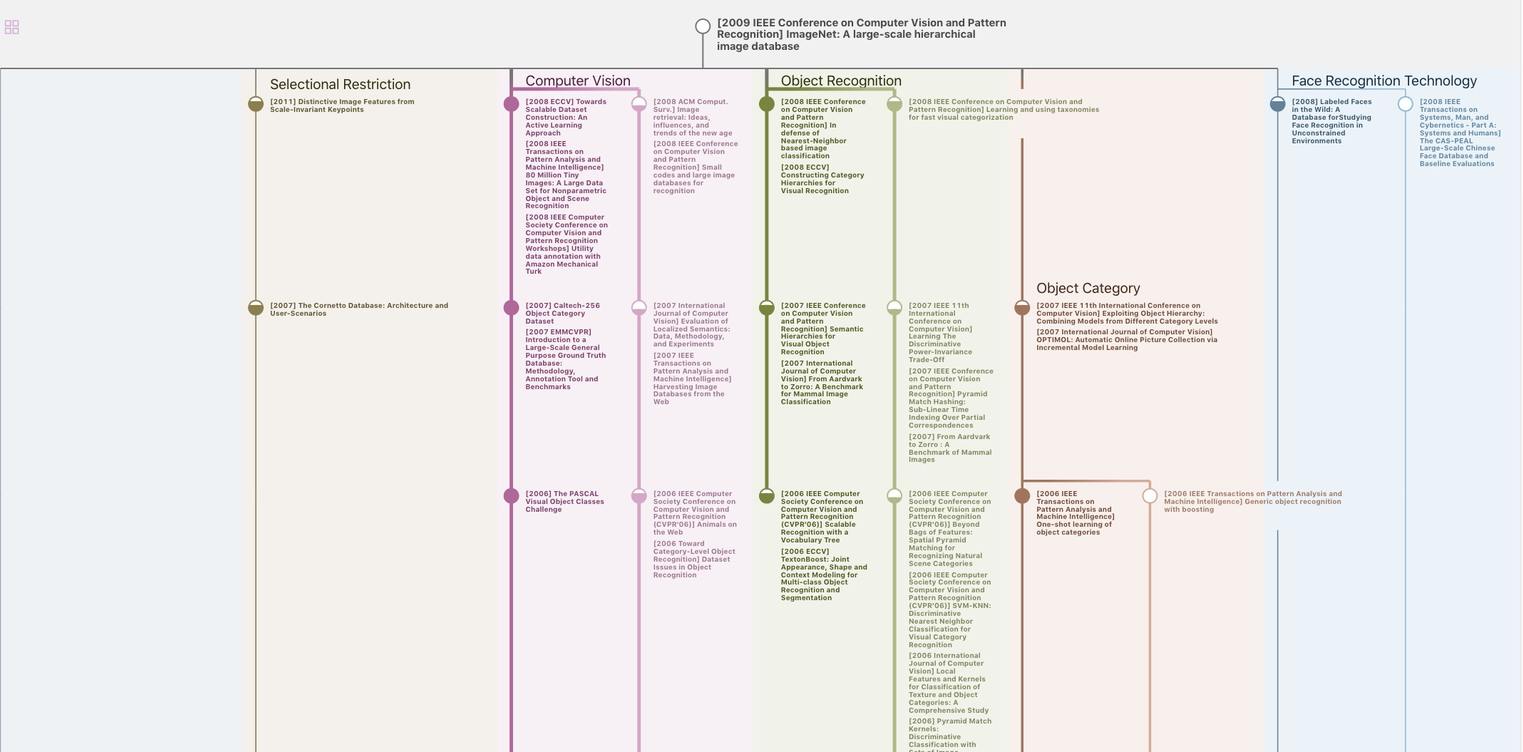
生成溯源树,研究论文发展脉络
Chat Paper
正在生成论文摘要