A Review of Convex Clustering From Multiple Perspectives: Models, Optimizations, Statistical Properties, Applications, and Connections.
IEEE transactions on neural networks and learning systems(2023)
摘要
Traditional partition-based clustering is very sensitive to the initialized centroids, which are easily stuck in the local minimum due to their nonconvex objectives. To this end, convex clustering is proposed by relaxing K -means clustering or hierarchical clustering. As an emerging and excellent clustering technology, convex clustering can solve the instability problems of partition-based clustering methods. Generally, convex clustering objective consists of the fidelity and the shrinkage terms. The fidelity term encourages the cluster centroids to estimate the observations and the shrinkage term shrinks the cluster centroids matrix so that their observations share the same cluster centroid in the same category. Regularized by the l -norm ( p ∈ {1,2,+∞} ), the convex objective guarantees the global optimal solution of the cluster centroids. This survey conducts a comprehensive review of convex clustering. It starts with the convex clustering as well as its nonconvex variants and then concentrates on the optimization algorithms and the hyperparameters setting. In particular, the statistical properties, the applications, and the connections of convex clustering with other methods are reviewed and discussed thoroughly for a better understanding the convex clustering. Finally, we briefly summarize the development of convex clustering and present some potential directions for future research.
更多查看译文
关键词
Convex clustering,first-order optimization algorithm,nonconvex regularization,second-order optimization algorithm,statistical property
AI 理解论文
溯源树
样例
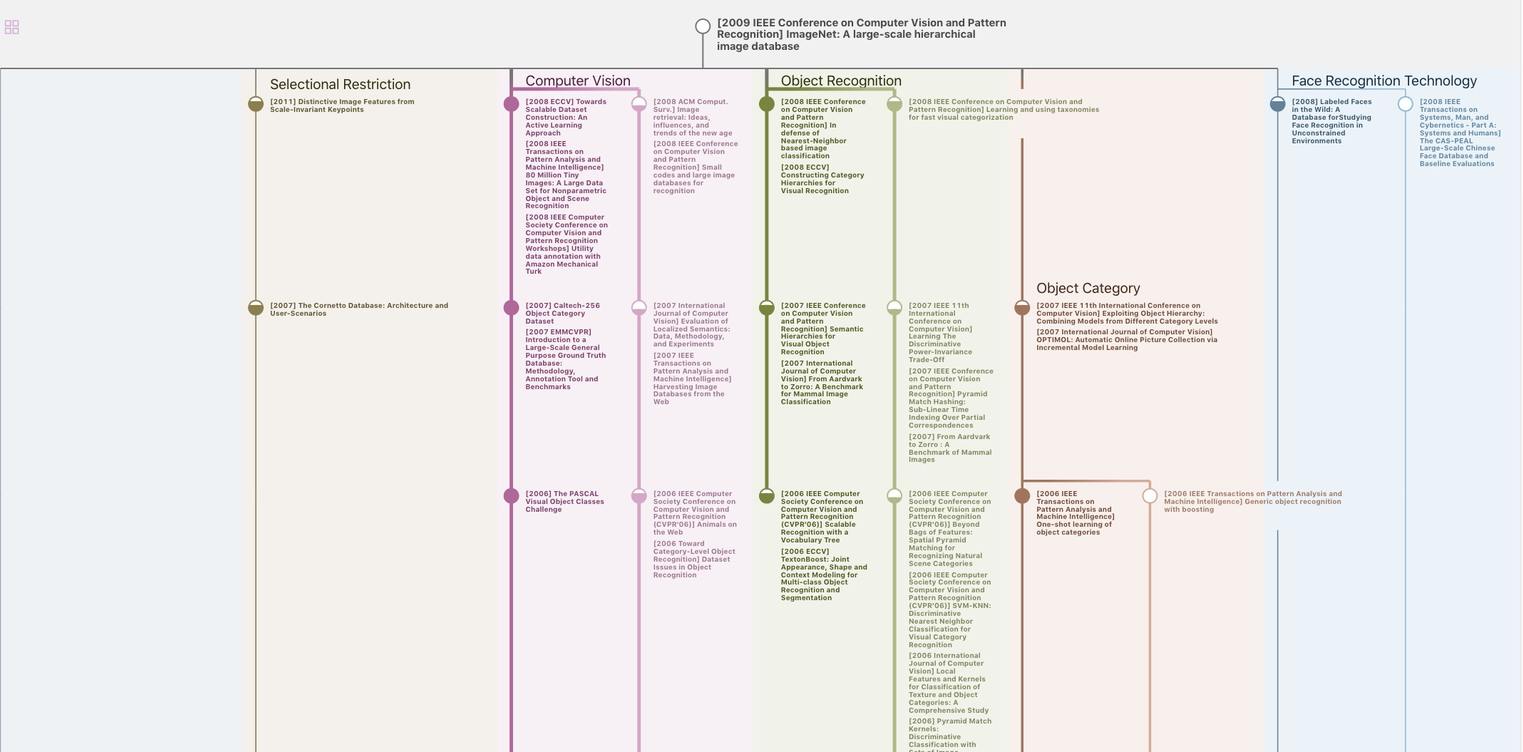
生成溯源树,研究论文发展脉络
Chat Paper
正在生成论文摘要