MedOptNet: Meta-Learning Framework for Few-shot Medical Image Classification.
IEEE/ACM transactions on computational biology and bioinformatics(2023)
摘要
In the medical research domain, limited data and high annotation costs have made efficient classification under few-shot conditions a popular research area. This paper proposes a meta-learning framework, termed MedOptNet, for few-shot medical image classification. The framework enables the use of various high-performance convex optimization models as classifiers, such as multi-class kernel support vector machines, ridge regression, and other models. End-to-end training is then implemented using dual problems and differentiation in the paper. Additionally, various regularization techniques are employed to enhance the model's generalization capabilities. Experiments on the BreakHis, ISIC2018, and Pap smear medical few-shot datasets demonstrate that the MedOptNet framework outperforms benchmark models. Moreover, the model training time is also compared to prove its effectiveness in the paper, and an ablation study is conducted to validate the effectiveness of each module.
更多查看译文
关键词
few-shot,meta learning,convex optimization,medical image classification
AI 理解论文
溯源树
样例
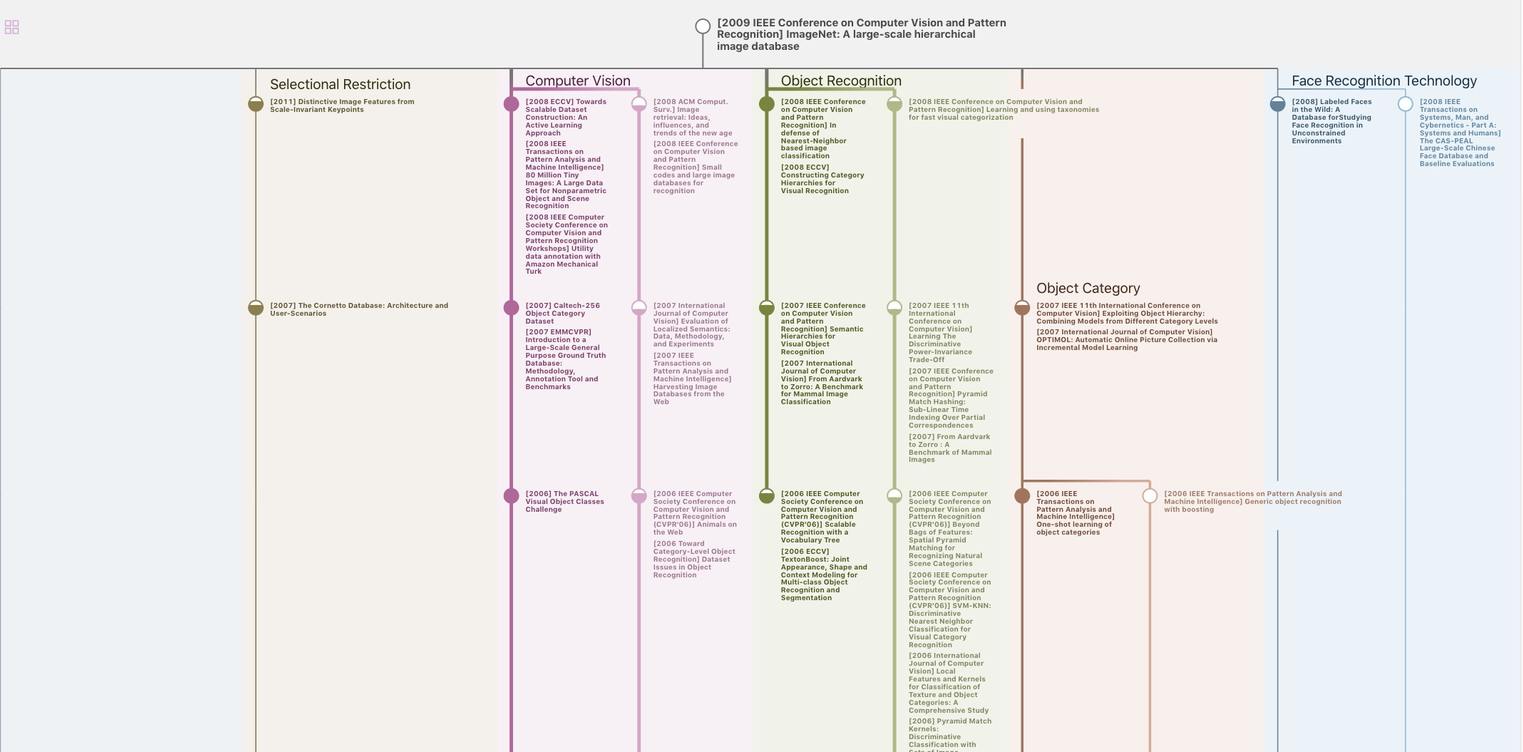
生成溯源树,研究论文发展脉络
Chat Paper
正在生成论文摘要