Use of Machine Learning for Dosage Individualization of Vancomycin in Neonates
Clinical pharmacokinetics(2023)
摘要
Background and Objective High variability in vancomycin exposure in neonates requires advanced individualized dosing regimens. Achieving steady-state trough concentration ( C 0 ) and steady-state area-under-curve (AUC 0–24 ) targets is important to optimize treatment. The objective was to evaluate whether machine learning (ML) can be used to predict these treatment targets to calculate optimal individual dosing regimens under intermittent administration conditions. Methods C 0 were retrieved from a large neonatal vancomycin dataset. Individual estimates of AUC 0–24 were obtained from Bayesian post hoc estimation. Various ML algorithms were used for model building to C 0 and AUC 0–24 . An external dataset was used for predictive performance evaluation. Results Before starting treatment, C 0 can be predicted a priori using the Catboost-based C 0 -ML model combined with dosing regimen and nine covariates. External validation results showed a 42.5% improvement in prediction accuracy by using the ML model compared with the population pharmacokinetic model. The virtual trial showed that using the ML optimized dose; 80.3% of the virtual neonates achieved the pharmacodynamic target ( C 0 in the range of 10–20 mg/L), much higher than the international standard dose (37.7–61.5%). Once therapeutic drug monitoring (TDM) measurements ( C 0 ) in patients have been obtained, AUC 0–24 can be further predicted using the Catboost-based AUC-ML model combined with C 0 and nine covariates. External validation results showed that the AUC-ML model can achieve an prediction accuracy of 80.3%. Conclusion C 0 -based and AUC 0–24 -based ML models were developed accurately and precisely. These can be used for individual dose recommendations of vancomycin in neonates before treatment and dose revision after the first TDM result is obtained, respectively.
更多查看译文
关键词
dosage individualization,vancomycin,machine learning
AI 理解论文
溯源树
样例
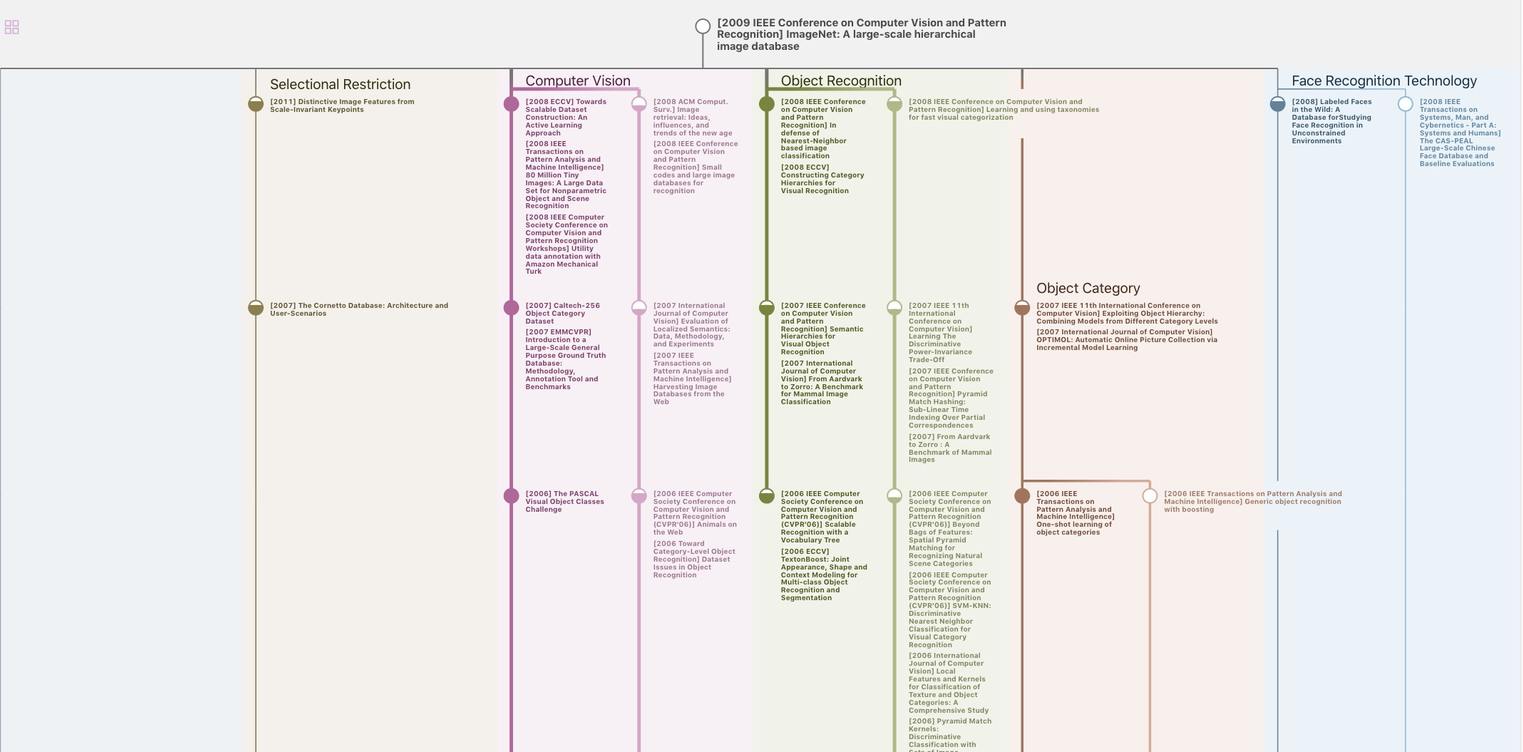
生成溯源树,研究论文发展脉络
Chat Paper
正在生成论文摘要