Self-Organizing a Latent Hierarchy of Sketch Patterns for Controllable Sketch Synthesis.
IEEE transactions on neural networks and learning systems(2023)
摘要
Encoding sketches as Gaussian mixture model (GMM)-distributed latent codes is an effective way to control sketch synthesis. Each Gaussian component represents a specific sketch pattern, and a code randomly sampled from the Gaussian can be decoded to synthesize a sketch with the target pattern. However, existing methods treat the Gaussians as individual clusters, which neglects the relationships between them. For example, the giraffe and horse sketches heading left are related to each other by their face orientation. The relationships between sketch patterns are important messages to reveal cognitive knowledge in sketch data. Thus, it is promising to learn accurate sketch representations by modeling the pattern relationships into a latent structure. In this article, we construct a tree-structured taxonomic hierarchy over the clusters of sketch codes. The clusters with the more specific descriptions of sketch patterns are placed at the lower levels, while the ones with the more general patterns are ranked at the higher levels. The clusters at the same rank relate to each other through the inheritance of features from common ancestors. We propose a hierarchical expectation-maximization (EM)-like algorithm to explicitly learn the hierarchy, jointly with the training of encoder-decoder network. Moreover, the learned latent hierarchy is utilized to regularize sketch codes with structural constraints. Experimental results show that our method significantly improves controllable synthesis performance and obtains effective sketch analogy results.
更多查看译文
关键词
Controllable sketch synthesis,hierarchical latent representation,latent code self-organization,sketch analogy,sketch representation learning
AI 理解论文
溯源树
样例
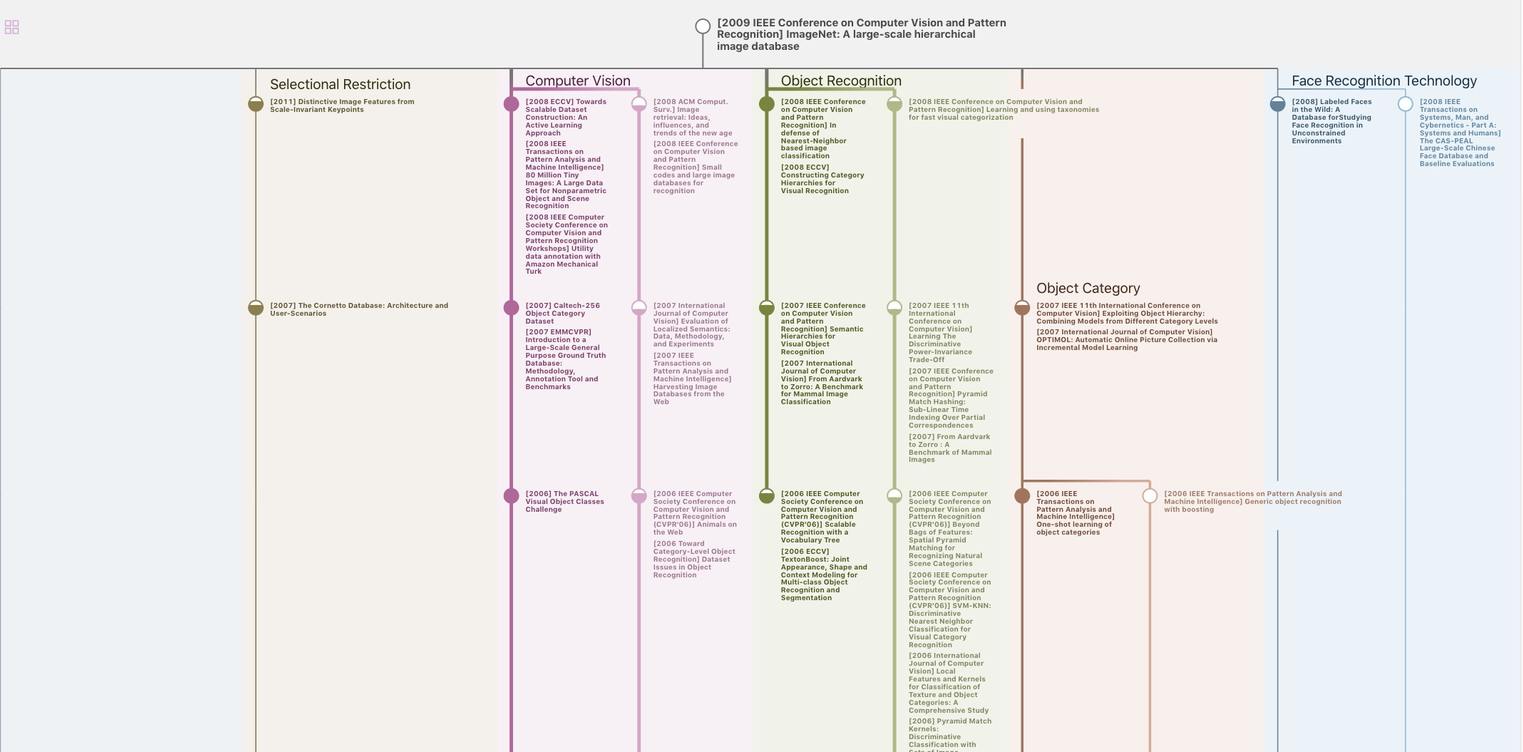
生成溯源树,研究论文发展脉络
Chat Paper
正在生成论文摘要