Health-Aware Food Recommendation Based on Knowledge Graph and Multi-Task Learning.
Foods (Basel, Switzerland)(2023)
摘要
Current food recommender systems tend to prioritize either the user's dietary preferences or the healthiness of the food, without considering the importance of personalized health requirements. To address this issue, we propose a novel approach to healthy food recommendations that takes into account the user's personalized health requirements, in addition to their dietary preferences. Our work comprises three perspectives. Firstly, we propose a collaborative recipe knowledge graph (CRKG) with millions of triplets, containing user-recipe interactions, recipe-ingredient associations, and other food-related information. Secondly, we define a score-based method for evaluating the healthiness match between recipes and user preferences. Based on these two prior perspectives, we develop a novel health-aware food recommendation model (FKGM) using knowledge graph embedding and multi-task learning. FKGM employs a knowledge-aware attention graph convolutional neural network to capture the semantic associations between users and recipes on the collaborative knowledge graph and learns the user's requirements in both preference and health by fusing the losses of these two learning tasks. We conducted experiments to demonstrate that FKGM outperformed four competing baseline models in integrating users' dietary preferences and personalized health requirements in food recommendations and performed best on the health task.
更多查看译文
关键词
knowledge graph,food,health-aware,multi-task
AI 理解论文
溯源树
样例
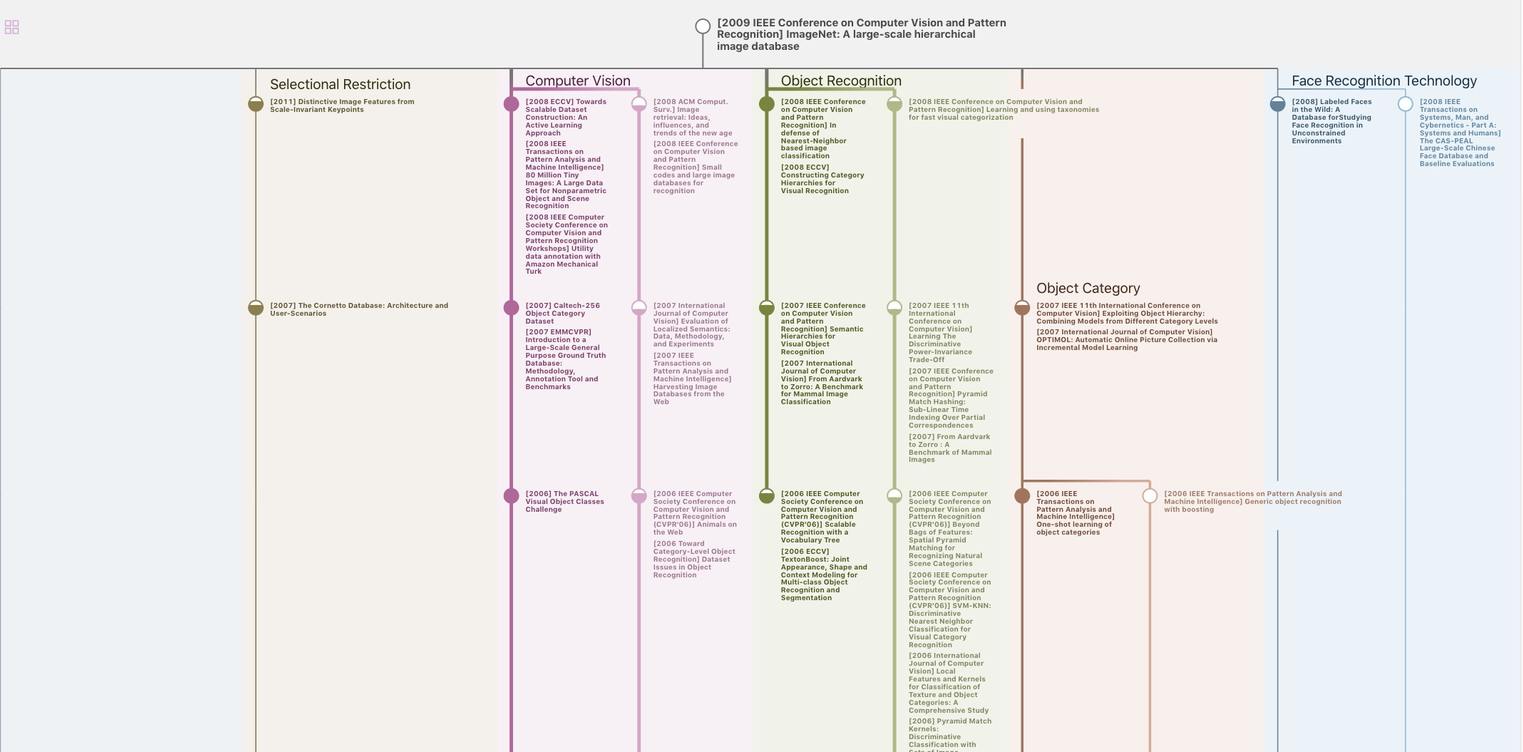
生成溯源树,研究论文发展脉络
Chat Paper
正在生成论文摘要