Federated Semi-Supervised Learning for Medical Image Segmentation via Pseudo-Label Denoising.
IEEE journal of biomedical and health informatics(2023)
摘要
Distributed big data and digital healthcare technologies have great potential to promote medical services, but challenges arise when it comes to learning predictive model from diverse and complex e-health datasets. Federated Learning (FL), as a collaborative machine learning technique, aims to address the challenges by learning a joint predictive model across multi-site clients, especially for distributed medical institutions or hospitals. However, most existing FL methods assume that clients possess fully labeled data for training, which is often not the case in e-health datasets due to high labeling costs or expertise requirement. Therefore, this work proposes a novel and feasible approach to learn a Federated Semi-Supervised Learning (FSSL) model from distributed medical image domains, where a federated pseudo-labeling strategy for unlabeled clients is developed based on the embedded knowledge learned from labeled clients. This greatly mitigates the annotation deficiency at unlabeled clients and leads to a cost-effective and efficient medical image analysis tool. We demonstrated the effectiveness of our method by achieving significant improvements compared to the state-of-the-art in both fundus image and prostate MRI segmentation tasks, resulting in the highest Dice scores of 89.23 and 91.95 respectively even with only a few labeled clients participating in model training. This reveals the superiority of our method for practical deployment, ultimately facilitating the wider use of FL in healthcare and leading to better patient outcomes.
更多查看译文
关键词
Federated learning,federated pseudo-labeling strategy,medical image segmentation
AI 理解论文
溯源树
样例
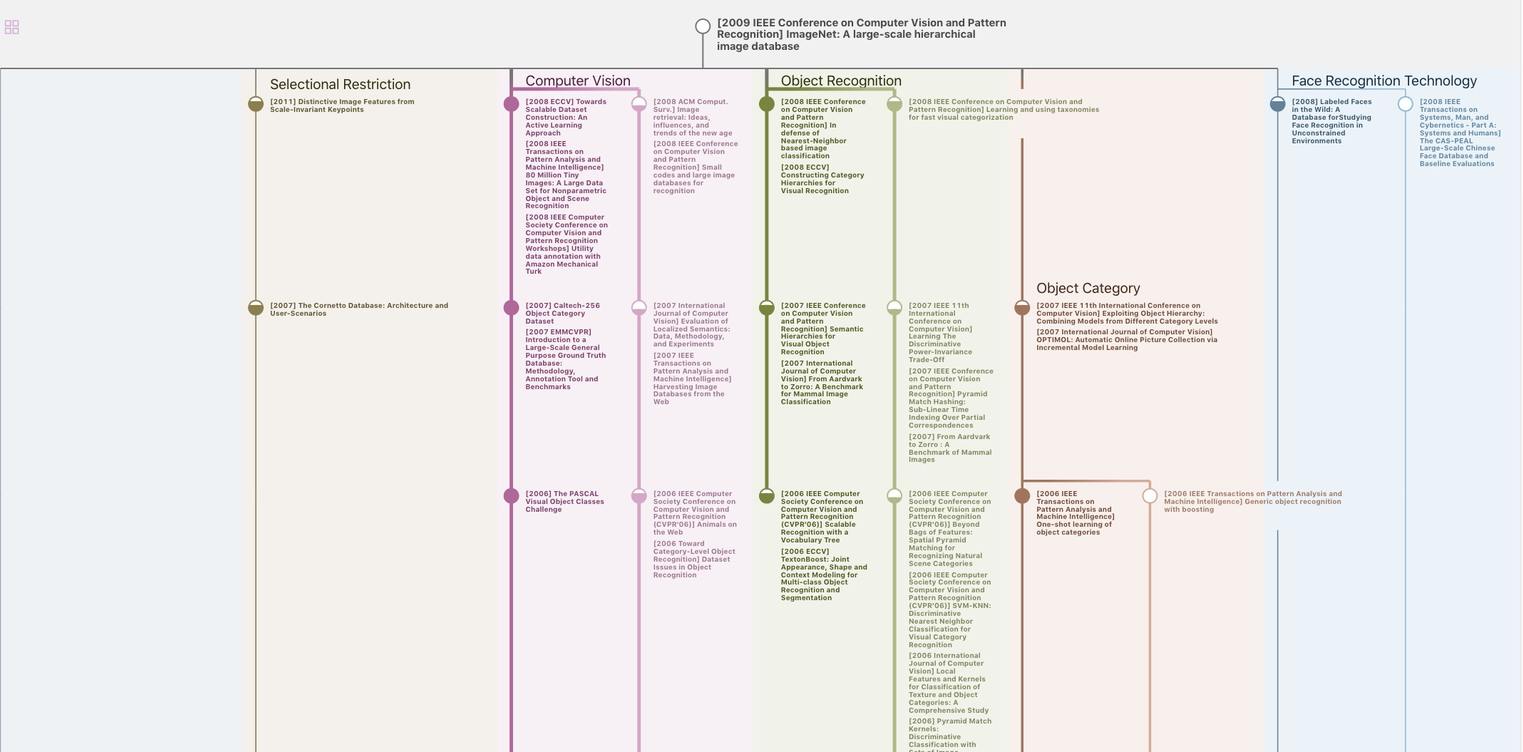
生成溯源树,研究论文发展脉络
Chat Paper
正在生成论文摘要