A deep-learning method for the end-to-end prediction of intracranial aneurysm rupture risk.
Patterns (New York, N.Y.)(2023)
摘要
It is critical to accurately predict the rupture risk of an intracranial aneurysm (IA) for timely and appropriate treatment because the fatality rate after rupture is . Existing methods relying on morphological features (e.g., height-width ratio) measured manually by neuroradiologists are labor intensive and have limited use for risk assessment. Therefore, we propose an end-to-end deep-learning method, called TransIAR net, to automatically learn the morphological features from 3D computed tomography angiography (CTA) data and accurately predict the status of IA rupture. We devise a multiscale 3D convolutional neural network (CNN) to extract the structural patterns of the IA and its neighborhood with a dual branch of shared network structures. Moreover, we learn the spatial dependence within the IA neighborhood with a transformer encoder. Our experiments demonstrated that the features learned by TransIAR are more effective and robust than handcrafted features, resulting in a improvement in the accuracy of rupture status prediction.
更多查看译文
关键词
intracranial aneurysm (IA),rupture risk,rupture status,convolutional neural network,CNN,transformer
AI 理解论文
溯源树
样例
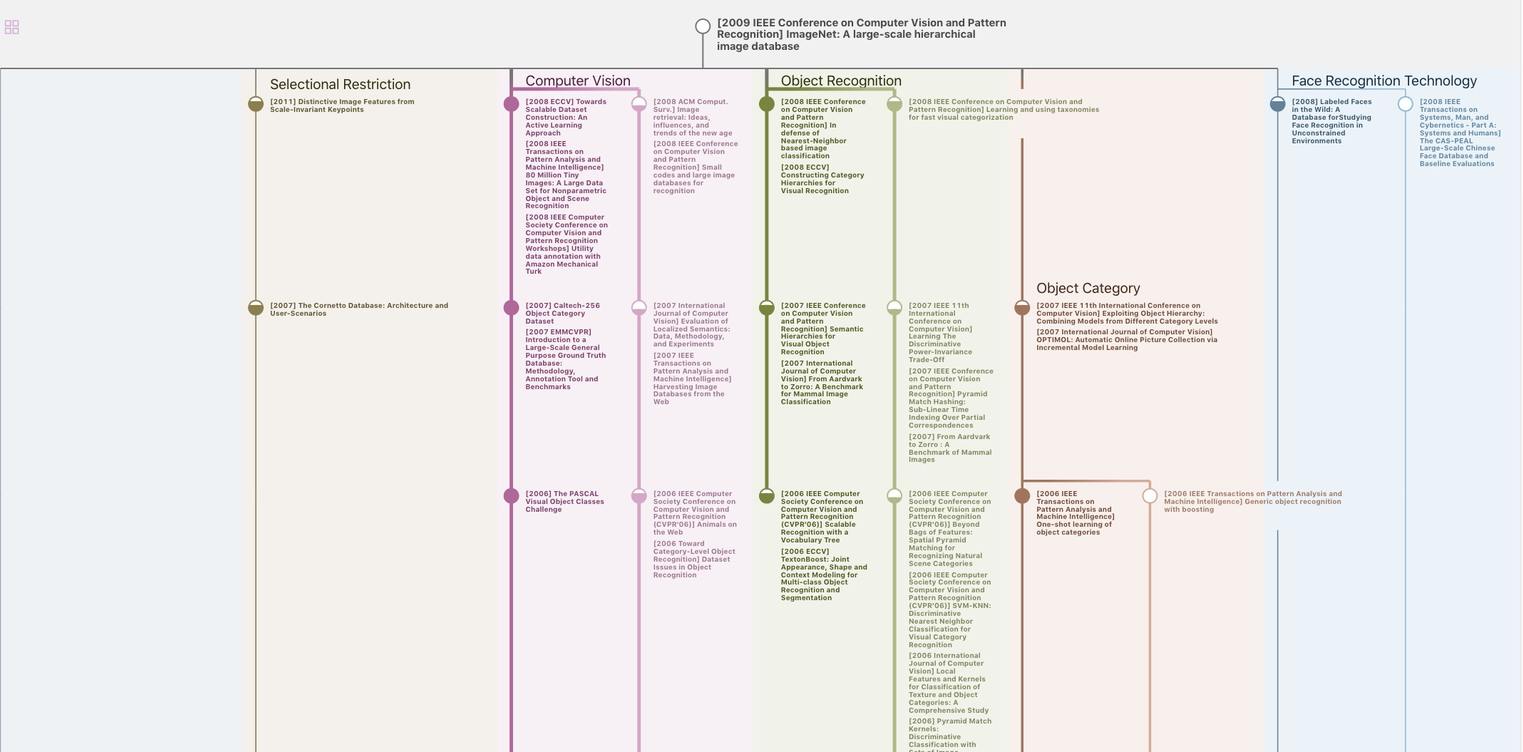
生成溯源树,研究论文发展脉络
Chat Paper
正在生成论文摘要