A Lightweight Convolutional Neural Network for Atrial Fibrillation Detection Using Dual-Channel Binary Features from Single-Lead Short ECG
2023 IEEE 5th International Conference on Artificial Intelligence Circuits and Systems (AICAS)(2023)
摘要
Atrial fibrillation (AF) is a prevalent cardiovascular disease in the elderly, significantly increasing the risk of stroke and heart failure, etc. While the artificial neural network (ANN) has recently demonstrated high accuracy in ECG-based AF detection, its high computation complexity makes it challenging for real-time and long-term monitoring on low-power wearable devices, which is critical for detecting paroxysmal AF. Therefore, in this work, a lightweight convolutional neural network for AF detection is proposed using a dual-channel binary features extraction technique from single-lead short ECG to achieve both high classification accuracy and low computation complexity, and evaluated on the 2017 PhysioNet/CinC Challenge dataset, the proposed method achieves 93.6% sensitivity and 0.81 F1 score for AF detection. Moreover, this design consumes only 1.83M parameters, achieving up to 27x reductions compared with prior works, and only needs 57M MACs for calculation. As a result, it is suitable for deployment in low-power wearable devices for long-term AF monitoring.
更多查看译文
关键词
Atrial fibrillation (AF),neural networks,Lightweight,single-lead ECG
AI 理解论文
溯源树
样例
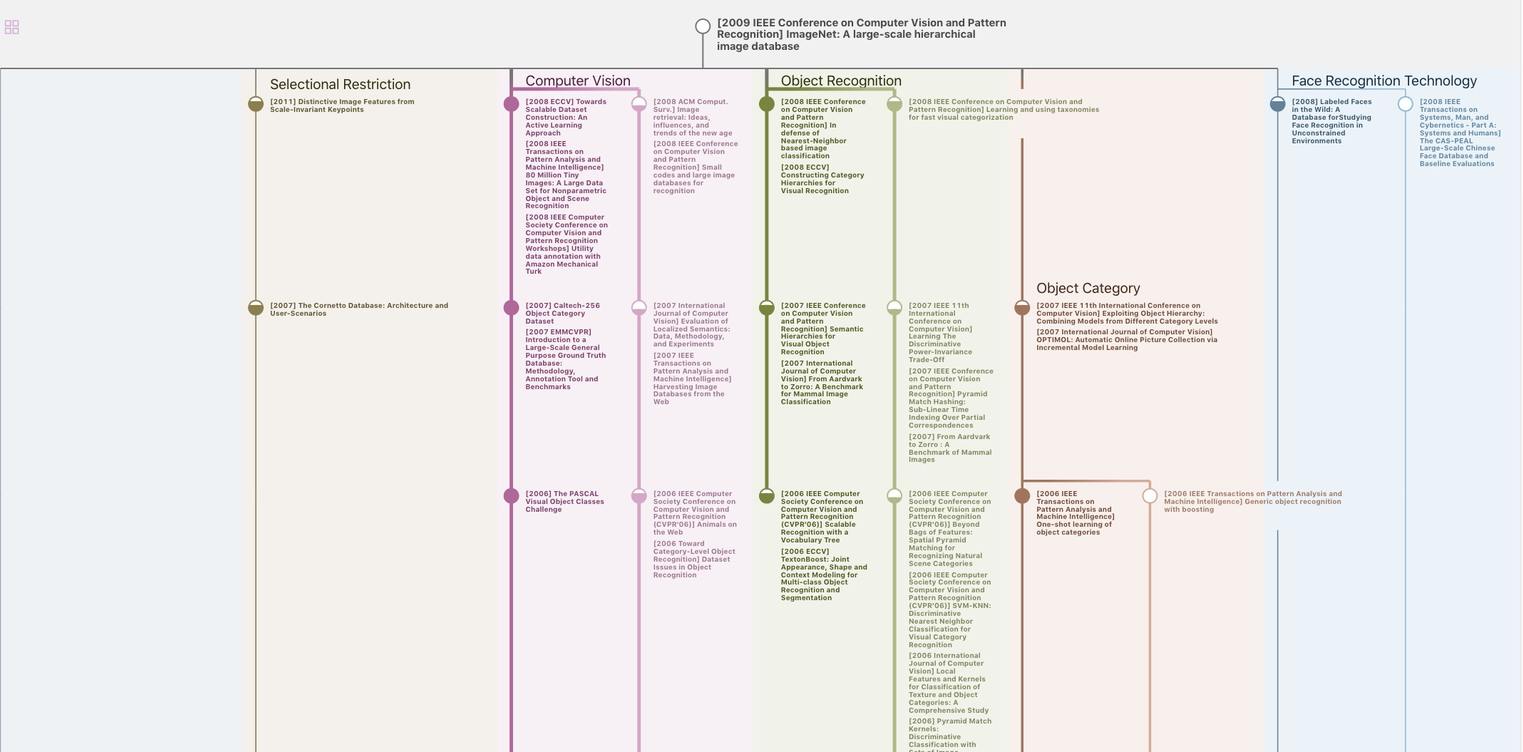
生成溯源树,研究论文发展脉络
Chat Paper
正在生成论文摘要