Unsupervised Learning of Spike-Timing-Dependent Plasticity Based on a Neuromorphic Implementation
2023 IEEE 5th International Conference on Artificial Intelligence Circuits and Systems (AICAS)(2023)
摘要
Spiking neural network is a promising endeavor to fulfill brain-like intelligence on the chip. Its learning rule, i.e., spike-timing-dependent plasticity (STDP), derived from neurobiology, is perceived as a powerful candidate to facilitate low-cost and high-performance unsupervised training. In this paper, we present a temporal coding based STDP learning method (TC-STDP) to verify the counter and look-up table based circuit design on a neuromorphic prototype chip. In order to perform on-chip STDP learning in an unsupervised manner, this paper concentrates more on detailing the experimental procedures and practical evaluations, where we introduce the matching score as a quantitative index to carry out label assignment and accuracy confirmation. Evaluation experiments demonstrate that the unsupervised STDP learning achieves best on-chip recognition accuracies of 93.51%, 80.33% on MNIST and EMNIST datasets, respectively. Moreover, experiments conducted on ModelNet40 3D dataset also validate the effectiveness of unsupervised STDP rule to perform possible incremental learning.
更多查看译文
关键词
unsupervised learning,spike-timing-dependent plasticity (STDP),spiking neural network (SNN),neuromorphic computing,on-chip inference & learning
AI 理解论文
溯源树
样例
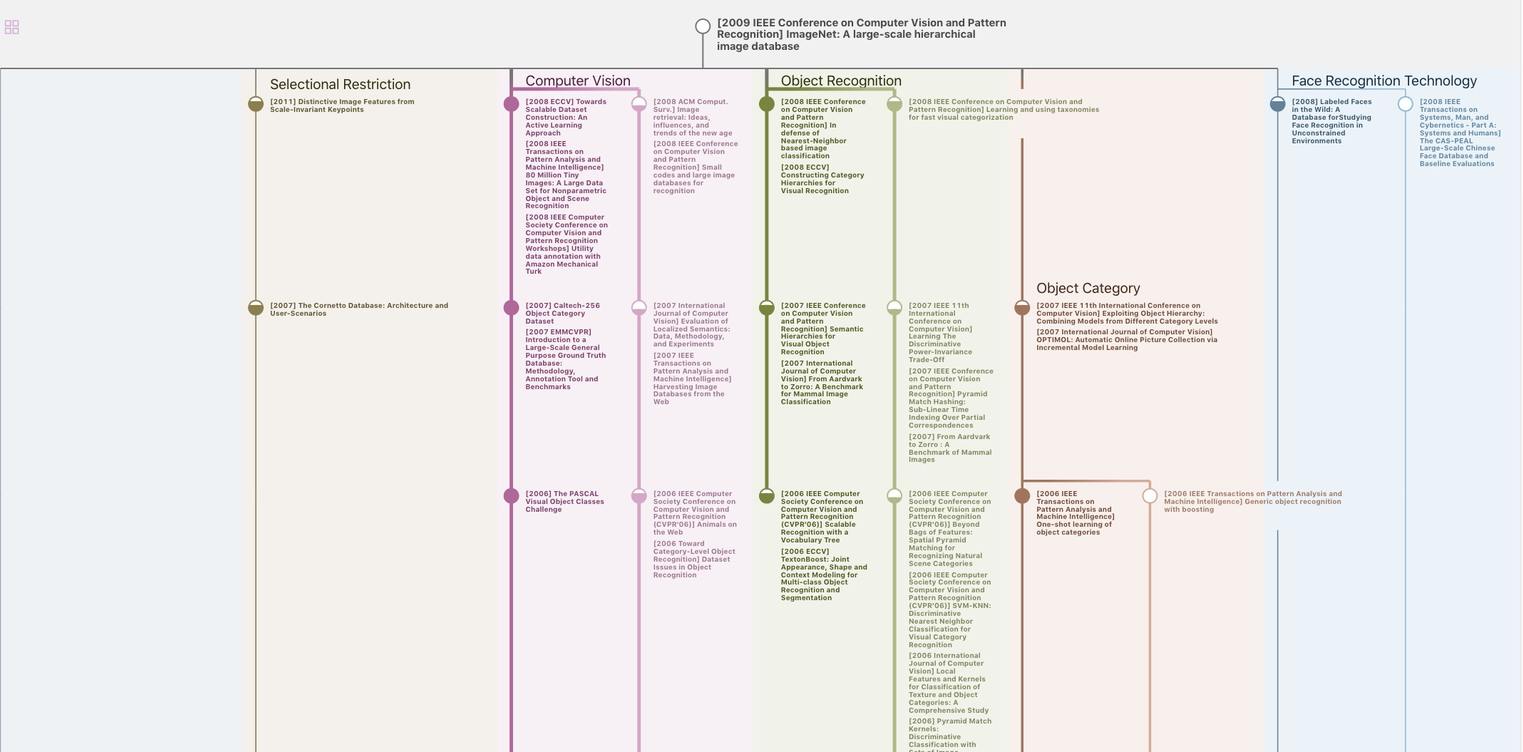
生成溯源树,研究论文发展脉络
Chat Paper
正在生成论文摘要