Robust feature learning against noisy labels
2023 IEEE INTERNATIONAL CONFERENCE ON IMAGE PROCESSING, ICIP(2023)
摘要
Supervised learning of deep neural networks heavily relies on large-scale datasets annotated by high-quality labels. In contrast, mislabeled samples can significantly degrade the generalization of models and result in memorizing samples, further learning erroneous associations of data contents to incorrect annotations. To this end, this paper proposes an efficient approach to tackle noisy labels by learning robust feature representation based on unsupervised augmentation restoration and cluster regularization. In addition, progressive self-bootstrapping is introduced to minimize the negative impact of supervision from noisy labels. Our proposed design is generic and flexible in applying to existing classification architectures with minimal overheads. Experimental results show that our proposed method can efficiently and effectively enhance model robustness under severely noisy labels.
更多查看译文
关键词
Image classification,noisy labels,robust feature learning
AI 理解论文
溯源树
样例
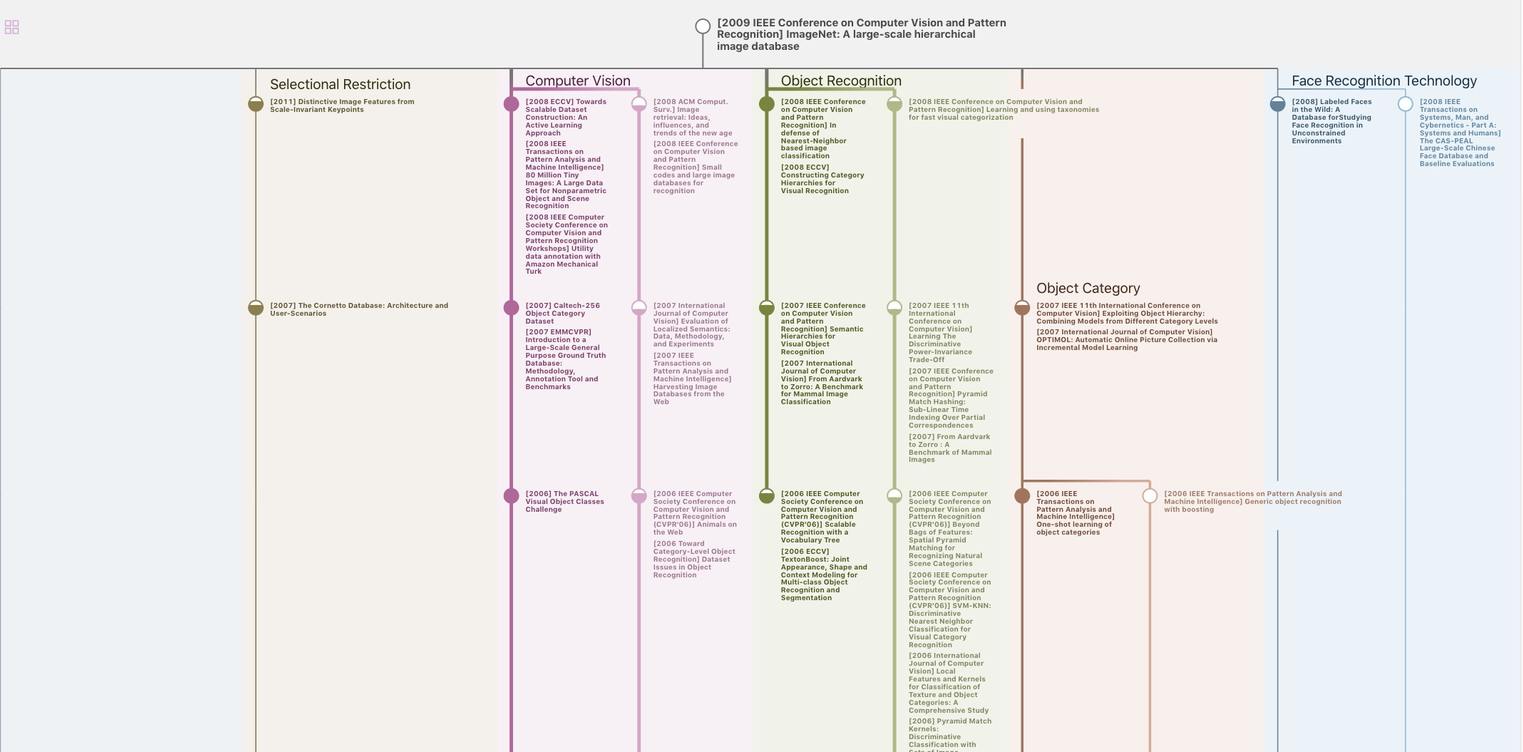
生成溯源树,研究论文发展脉络
Chat Paper
正在生成论文摘要