Bounding data reconstruction attacks with the hypothesis testing interpretation of differential privacy
CoRR(2023)
摘要
We explore Reconstruction Robustness (ReRo), which was recently proposed as an upper bound on the success of data reconstruction attacks against machine learning models. Previous research has demonstrated that differential privacy (DP) mechanisms also provide ReRo, but so far, only asymptotic Monte Carlo estimates of a tight ReRo bound have been shown. Directly computable ReRo bounds for general DP mechanisms are thus desirable. In this work, we establish a connection between hypothesis testing DP and ReRo and derive closed-form, analytic or numerical ReRo bounds for the Laplace and Gaussian mechanisms and their subsampled variants.
更多查看译文
关键词
data reconstruction attacks
AI 理解论文
溯源树
样例
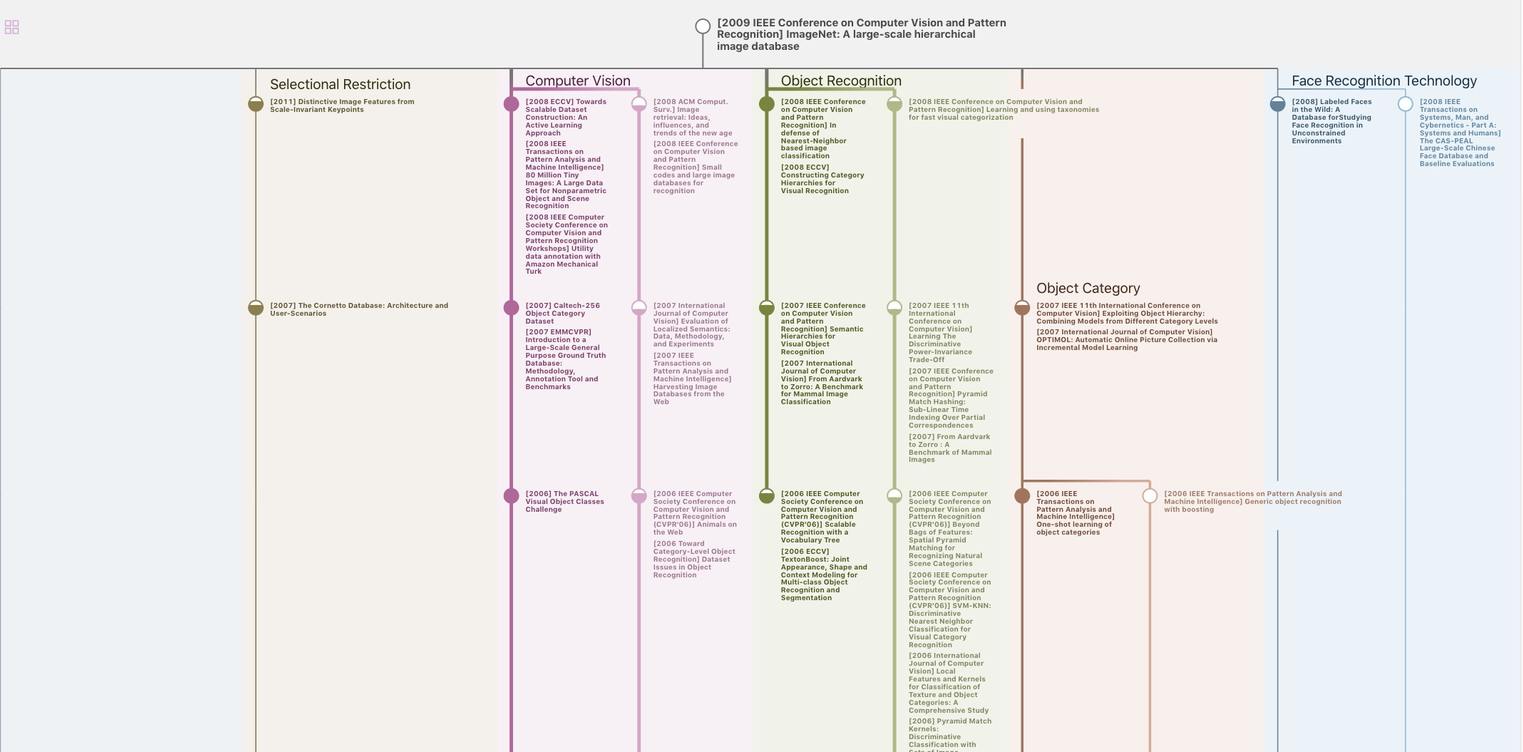
生成溯源树,研究论文发展脉络
Chat Paper
正在生成论文摘要