Attention guided domain alignment for conditional face image generation
Computer Vision and Image Understanding(2023)
摘要
Despite the great success of Generative Adversarial Network (GAN) in face image generation, it is still a challenge to generate a faithful yet high-fidelity face image given an exemplar image and a conditional input from a distinct domain (e.g., a semantic segmentation mask or an edge map). Existing methods learn image level features to align distinct domains in an intermediate domain, which ignore the spatial relationship of the facial semantic parts and therefore lead to semantic mismatching problems. In addition, it is computationally expensive to establish dense correspondences in the intermediate domain, especially for high-resolution face images. To address these problems, we propose a novel attention guided domain alignment method for conditional face image generation, which aligns two domains directly under the guidance of the local attention learned from semantically similar face parts. In particular, we assign a dedicated index for each feature block and adopt a top-k ranking operation to correspond block-wise features in two distinct domains, which exploits the spatial relationship of facial parts and preserves the texture structure during alignment. The local attention is then learned from the retrieved blocks, which reduces computation complexity substantially and therefore enables to build alignment in a high resolution. The aligned features are finally fused with adaptive weights learned from their long-range correlation coefficients, which capture the semantic coherence of the style features between the two domains. Extensive experimental results on the CelebAMask-HQ dataset demonstrate that the proposed method is superior to the state-of-the-art methods.
更多查看译文
关键词
Conditional face image generation,Top-k ranking,Domain alignment,Optimal Transport
AI 理解论文
溯源树
样例
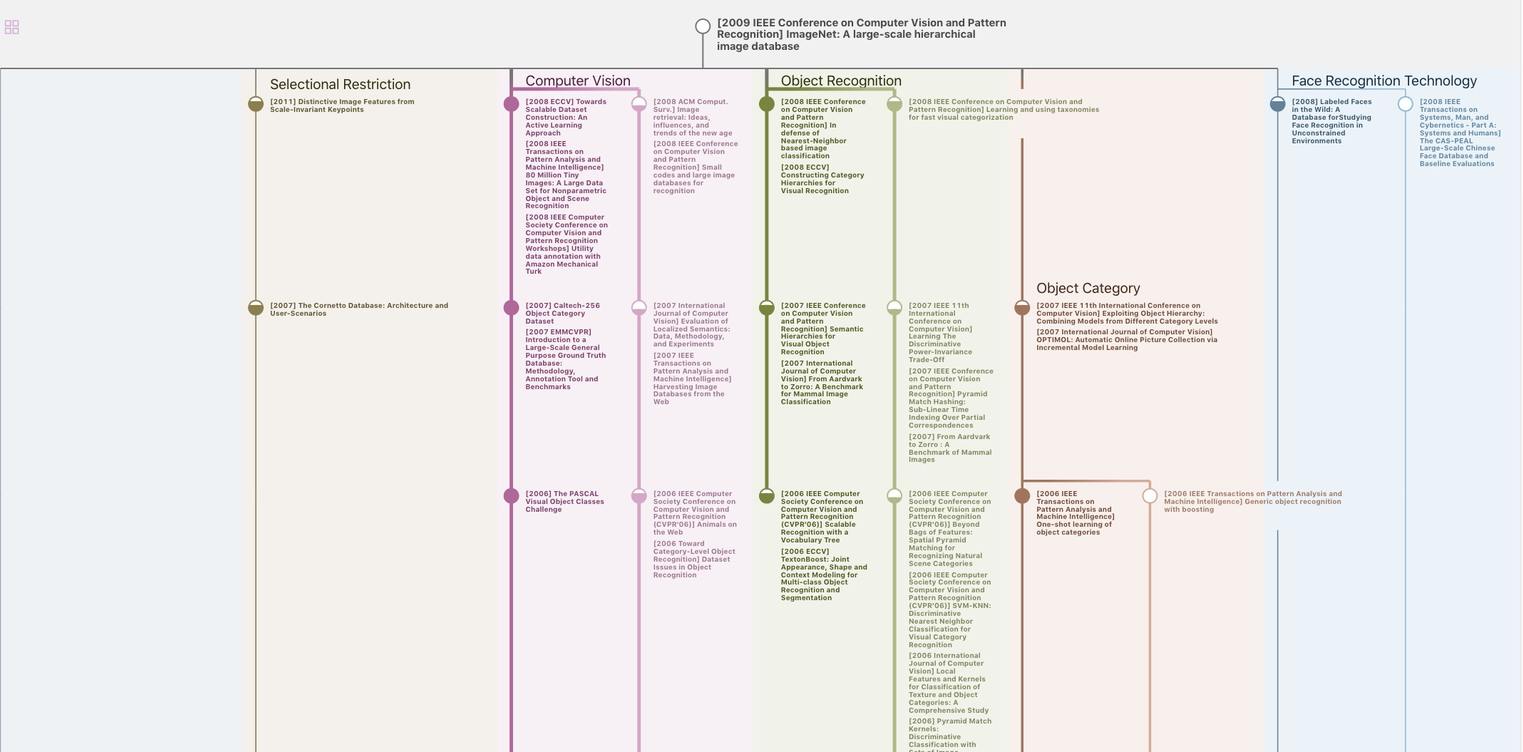
生成溯源树,研究论文发展脉络
Chat Paper
正在生成论文摘要