A comprehensive gas recognition algorithm with label-free drift compensation based on domain adversarial network
Sensors and Actuators B: Chemical(2023)
摘要
For most applications, the sensor system needs to identify/measure target gases in mixture conditions simultaneously, while countering the performance degradation caused by the drift deviation and batch to batch discrepancy. To solve these problems, we propose a comprehensive algorithm, hybrid attention-based transformer network with domain adversarial learning (HATN-DA). Inside it, multi-head transformer and channel-wise attention mechanism serving as main blocks of the feature extractor effectively capture temporal dependencies and lays emphasis on the diversities of sensors. Then, the capsule vector is applied in the predictor to encode gas species into the concentration information. Besides, the domain discriminator calculates Wasserstein distance of gas features between source and target domains, which is optimized through the back propagation by the feature extractor during the training process. Based on this, label-free drift compensation and transfer calibration effects are achieved: for public dataset, HATN-DA achieves 97.50∼100% accuracies for drift compensation experiment; for dataset of mixture gases, in the batch transfer tasks, HATN-DA achieves an accuracy of 98.79%, significantly improved compared to 90.16% (before transfer). Besides, in the cross-domain real-time concentration prediction tasks, HATN-DA achieves a normalized root mean squared error of 3.32%, 3.25%, 6.62% and 3.01% for ethanol, methane, carbon monoxide and ethylene.
更多查看译文
关键词
Transfer calibration, Drift compensation, Electronic nose, Mixture gases, Concentration measurement
AI 理解论文
溯源树
样例
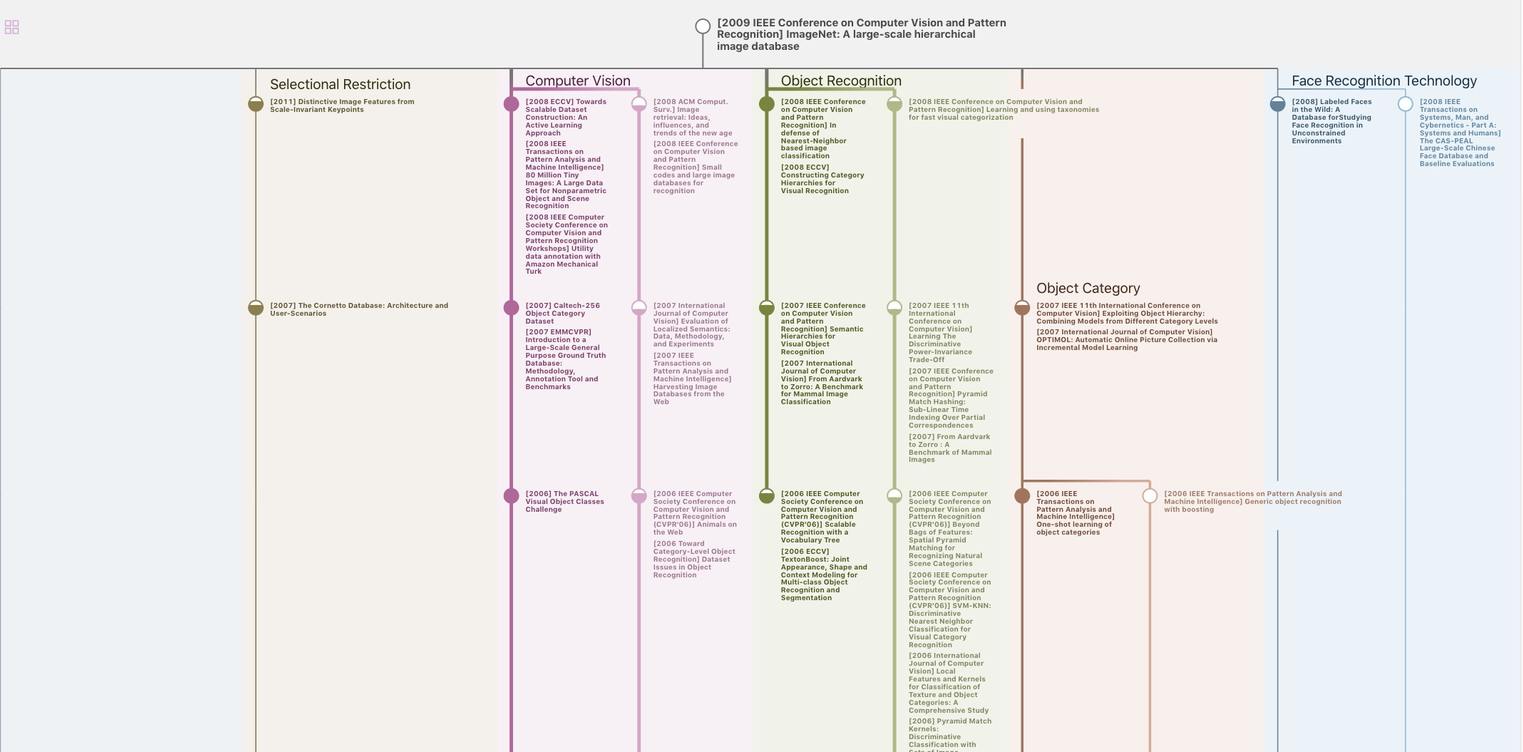
生成溯源树,研究论文发展脉络
Chat Paper
正在生成论文摘要