An integrated control strategy for simultaneous robot assignment, tool change and preventive maintenance scheduling using Heterogeneous Graph Neural Network
Robotics and Computer-Integrated Manufacturing(2023)
摘要
There has been a leap in the field of smart manufacturing with the advancement of automation systems, robotic technology, big data analytics, and state-of-the-art Artificial Intelligence (AI) and Machine Learning (ML) algorithms. Three very important aspects of smart manufacturing systems are system productivity, product quality, and maintenance of machines and equipment. These three issues are strongly interrelated and collectively determine the performance of a smart manufacturing system. Although there has been significant studies in production control, quality control and maintenance scheduling to address each of these aspects individually, there has been a lack of sufficient studies taking all of them into consideration in one control scheme. In this paper, a mobile multi-skilled robot operated Flexible Manufacturing System (FMS) is considered and a model that integrates robots, individual workstation processes and product quality is developed using a Heterogeneous Graph Structure. Heterogeneous Graph Neural Network (HGNN) is used to aggregate local information from different nodes of the graph model to create node embeddings that represent global information. A control problem is then formulated in the Decentralized Partially Observable Markov Decision Process (Dec-POMDP) framework to simultaneously consider robot assignment, product quality and maintenance scheduling. The problem is solved using Multi-Agent Reinforcement Learning (MARL). A case study is presented to demonstrate the effectiveness of the HGNN-MARL control strategy by comparing it to three baselines and the naive MARL policy without HGNN.
更多查看译文
关键词
simultaneous robot assignment,preventive maintenance scheduling,integrated control strategy,tool change
AI 理解论文
溯源树
样例
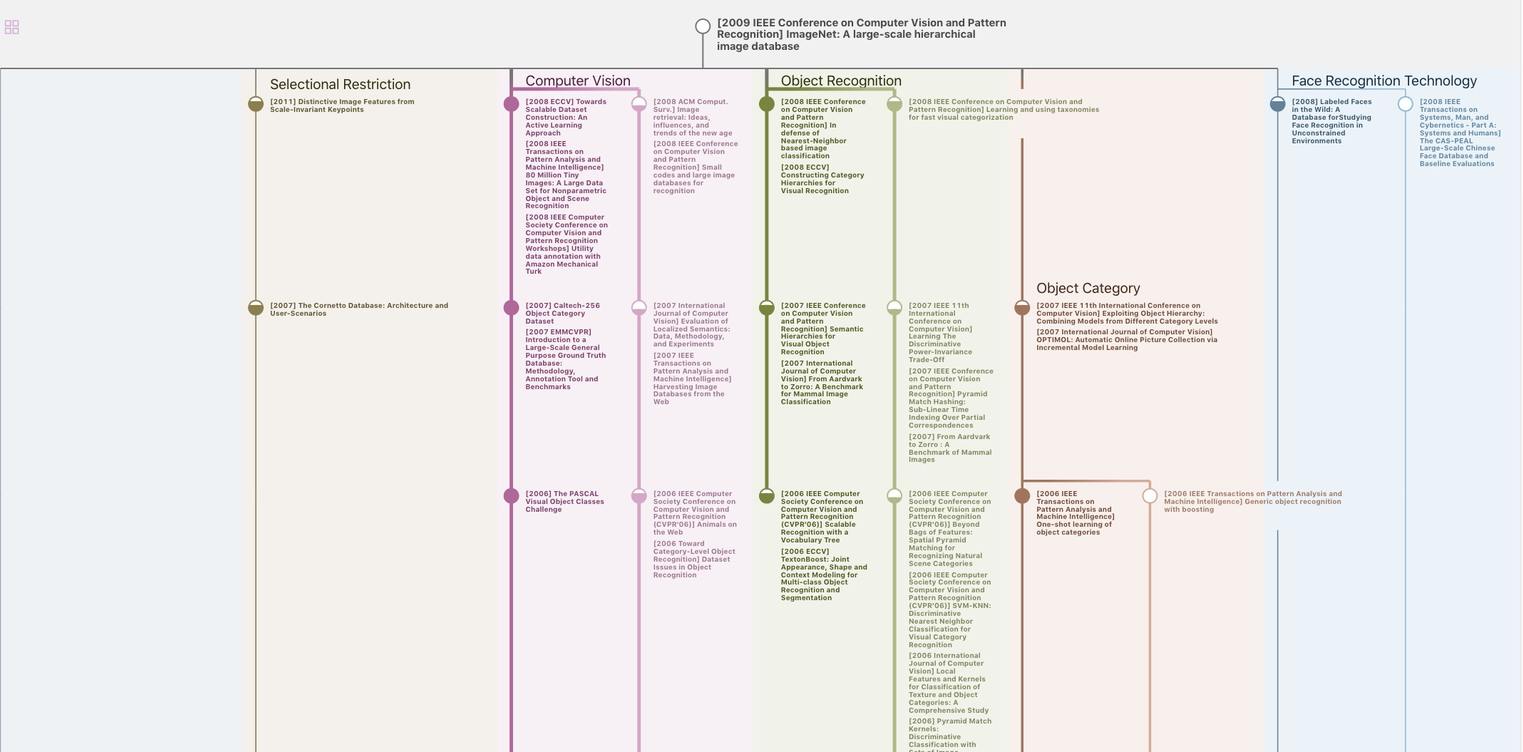
生成溯源树,研究论文发展脉络
Chat Paper
正在生成论文摘要