Deep learning based closed-loop well control optimization of geothermal reservoir with uncertain permeability
Renewable Energy(2023)
摘要
To maximize the economic benefits of geothermal energy production, it is essential to optimize geothermal reservoir management strategies, in which geologic uncertainty should be considered. In this work, we propose a closed-loop optimization framework, based on deep learning surrogates, for the well control optimization of geothermal reservoirs. In this framework, we construct a hybrid convolution–recurrent neural network surrogate, which combines the convolution neural network (CNN) and long short-term memory (LSTM) recurrent network. The convolution structure can extract spatial information of reservoir property fields and the recurrent structure can approximate sequence-to-sequence mapping. The trained model can predict time-varying production responses (rate, temperature, etc.) for cases with different permeability fields and well control sequences. In this closed-loop optimization framework, production optimization, based on the differential evolution (DE) algorithm, and data assimilation, based on the iterative ensemble smoother (IES), are performed alternately to achieve a real-time well control optimization and to estimate reservoir properties (e.g. permeability) as the production proceeds. In addition, the averaged objective function over the ensemble of geologic parameter estimates is adopted to consider geologic uncertainty in the optimization process. Geothermal reservoir production cases are examined to evaluate the performance of the proposed closed-loop optimization framework. Our results show that the proposed framework can achieve efficient and effective real-time optimization and data assimilation in the geothermal reservoir production process.
更多查看译文
关键词
geothermal reservoir,uncertain permeability,optimization,closed-loop
AI 理解论文
溯源树
样例
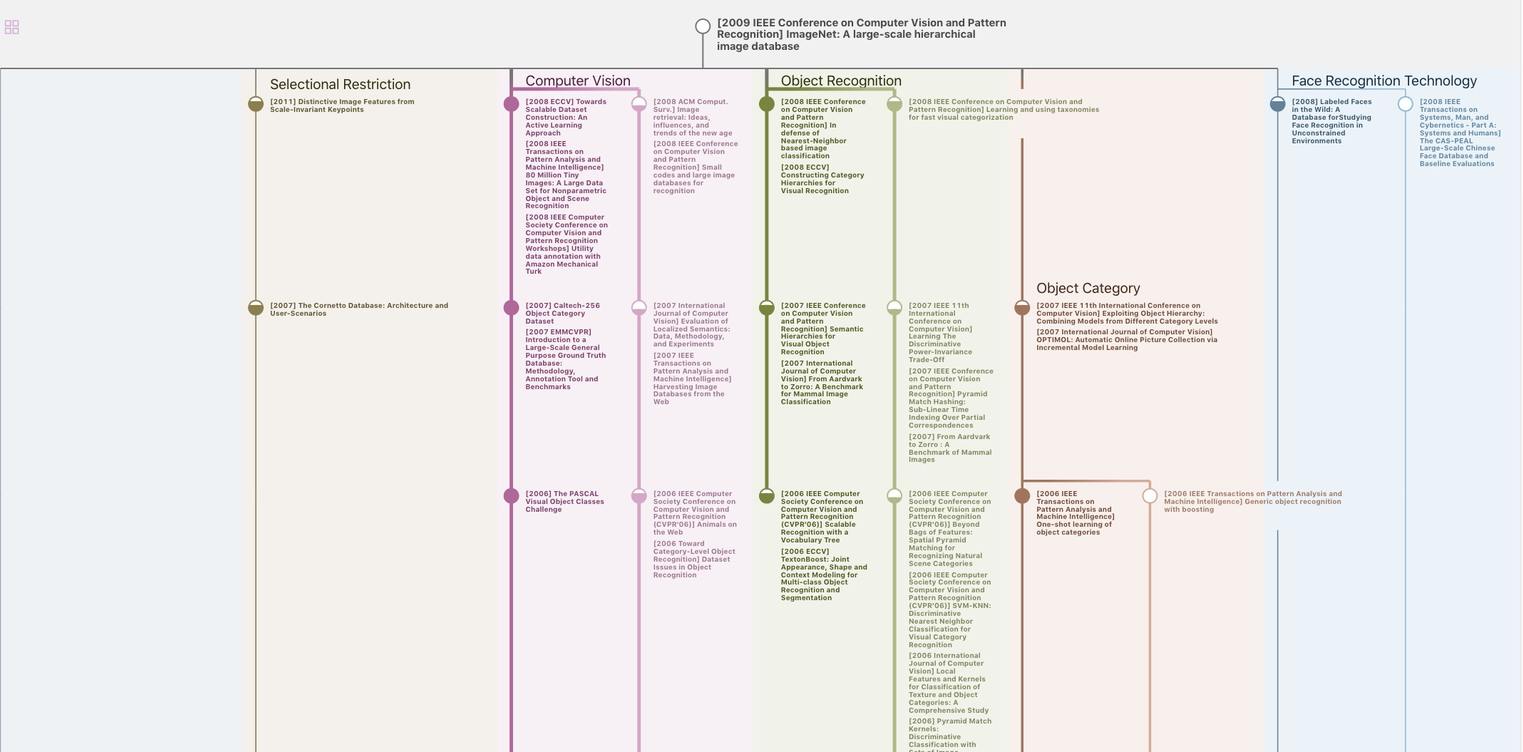
生成溯源树,研究论文发展脉络
Chat Paper
正在生成论文摘要