Disentangling high-level factors and their features with conditional vector quantized VAEs.
Pattern Recognition Letters(2023)
摘要
Two recent works have shown the benefit of modeling both high-level factors and their related features to learn disentangled representations with variational autoencoders (VAE). We propose here a novel VAEbased approach that follows this principle. Inspired by conditional VAE, the features are no longer treated as random variables over which integration must be performed. Instead, they are deterministically computed from the input data using a neural network whose parameters can be estimated jointly with those of the decoder and of the encoder. Moreover, the quality of the generated images has been improved by using discrete latent variables and a two-step learning procedure, which makes it possible to increase the size of the latent space without altering the disentanglement properties of the model. Results obtained on two different datasets validate the proposed approach that achieves better performance than the two aforementioned works in terms of disentanglement, while providing higher quality images.& COPY; 2023 Elsevier B.V. All rights reserved.
更多查看译文
关键词
Variational autoencoder,Disentangled representation learning,Generative models
AI 理解论文
溯源树
样例
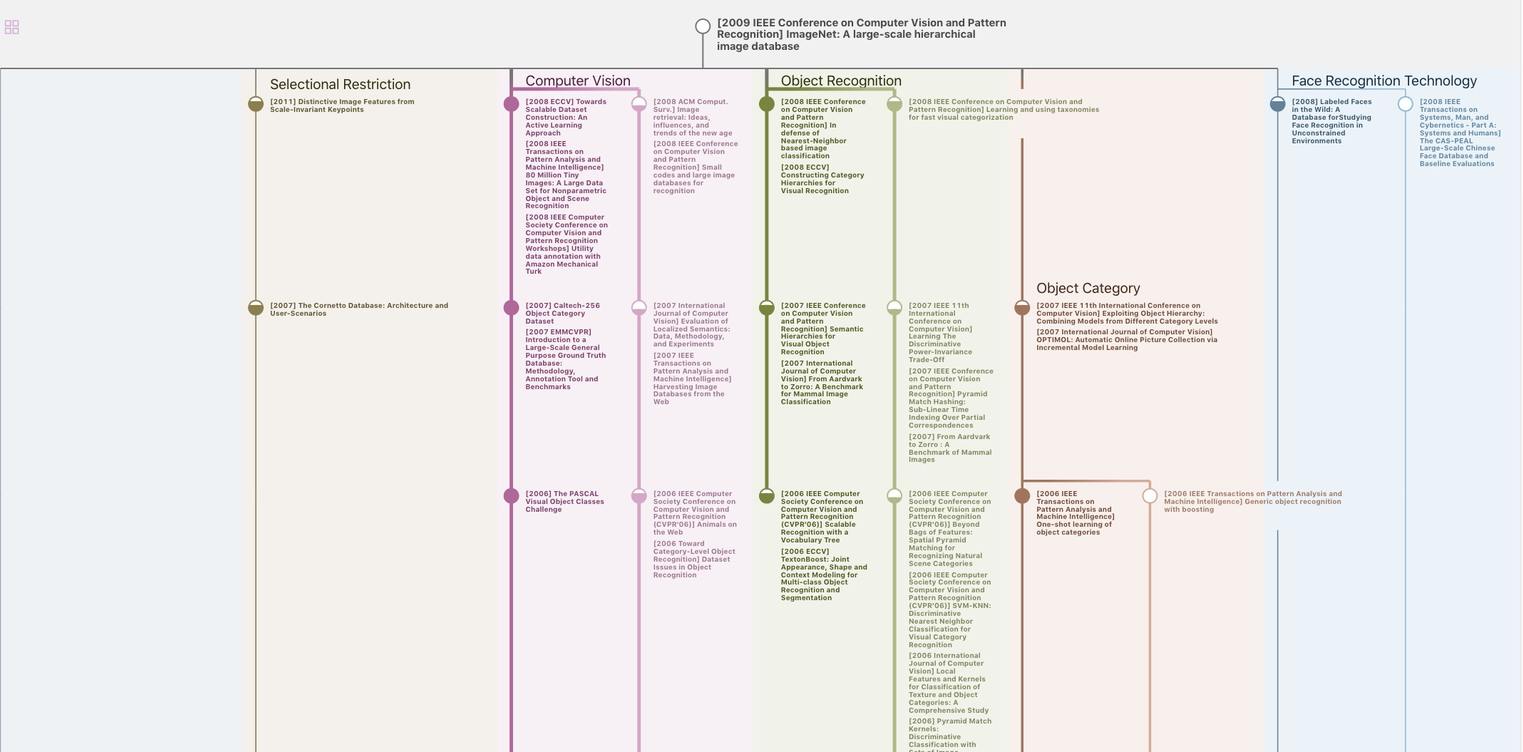
生成溯源树,研究论文发展脉络
Chat Paper
正在生成论文摘要