Robust anomaly detection for multivariate time series through temporal GCNs and attention-based VAE
Knowledge-Based Systems(2023)
摘要
Anomaly detection on multivariate time series (MTS) is of great importance in both data mining research and industrial applications. While a handful of anomaly detection models are developed for MTS data, most of them either ignore the potential correlations between different variables or overlook the different importance of variables at each time period in MTS, which leads to poor accuracy in anomaly detection. In this paper, we propose a novel unsupervised MUltivariate Time series ANomaly deTection framework (MUTANT), which simultaneously models the correlations between variables and the importance of variables at each time period. Specifically, we construct a feature graph for variables in each time window and perform graph convolutional network (GCN) to learn embeddings for all variables, which effectively captures the time-varying correlations between variables in MTS. Then, we propose an attention-based reconstruction model to learn robust latent representations to capture normal patterns of MTS by modeling the importance of variables based on time dependencies along with time dimension. Our evaluation experiments are conducted on four real-life datasets from different industrial domains. Experimental results show that MUTANT significantly outperforms state-of-the-art MTS anomaly detection methods, achieving an average anomaly detection F1-score higher than 0.96. The source code is available at https://github.com/Coac-syf/MUTANT.
更多查看译文
关键词
Anomaly detection,Multivariate time series,Unsupervised learning,Graph neural networks,Variational auto-encoder
AI 理解论文
溯源树
样例
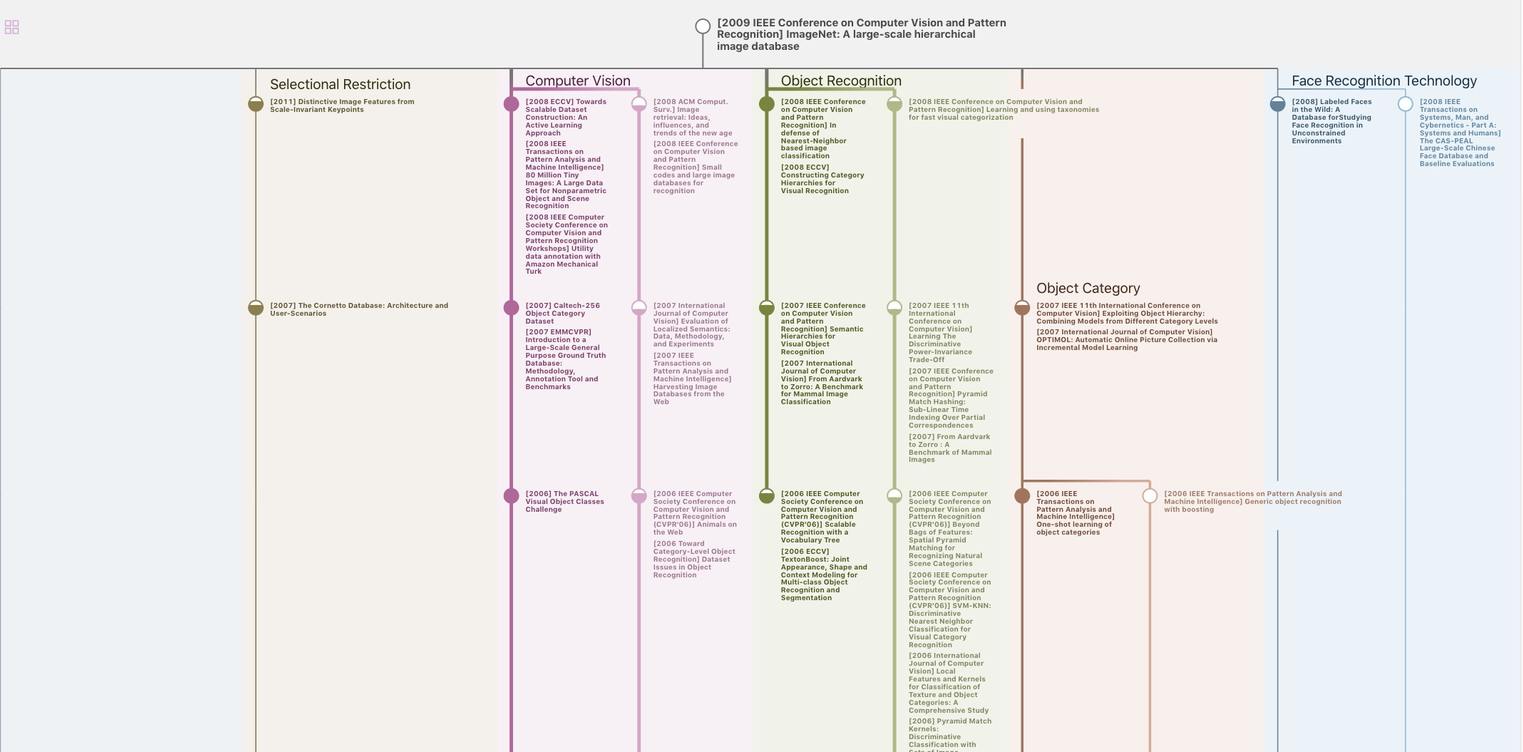
生成溯源树,研究论文发展脉络
Chat Paper
正在生成论文摘要