Differential evolution-driven traffic light scheduling for vehicle-pedestrian mixed-flow networks
Knowledge-Based Systems(2023)
摘要
This study addresses the bi-objective traffic light scheduling problem (TLSP), which aims to minimize the network-wise delay time of all vehicles and pedestrians within a predefined finite-time window. In this study, to solve this real-time optimization problem, an efficient discrete differential evolution-driven approach named DDE is proposed. The DDE includes discrete versions of mutation and crossover schemes together with a usual selection operation. Furthermore, an additional operator called greedy local search operation is combined with the search procedure of the DDE to increase the convergence speed. Finally, numerical experiments are conducted on 32 different traffic case studies generated based on the infrastructure of traffic network in Jurong area of Singapore. The optimization results produced by the DDE are compared with the optimal results achieved by the commercial GUROBI solver. The performance of the DDE is also compared with other metaheuristics namely ABC, GA, HS, Jaya, DSCA and DSCA-LS, which are designed in the literature to solve the TLSP. The performance comparison is analyzed using diverse metrics such as statistical values of optimization results, statistical analysis using the Wilcoxon signed-rank test, average relative error percentage, and convergence analysis. The comparison illustrates the significantly better and promising search ability of the DDE as compared to the other metaheuristics.
更多查看译文
关键词
light scheduling,traffic,evolution-driven,vehicle-pedestrian,mixed-flow
AI 理解论文
溯源树
样例
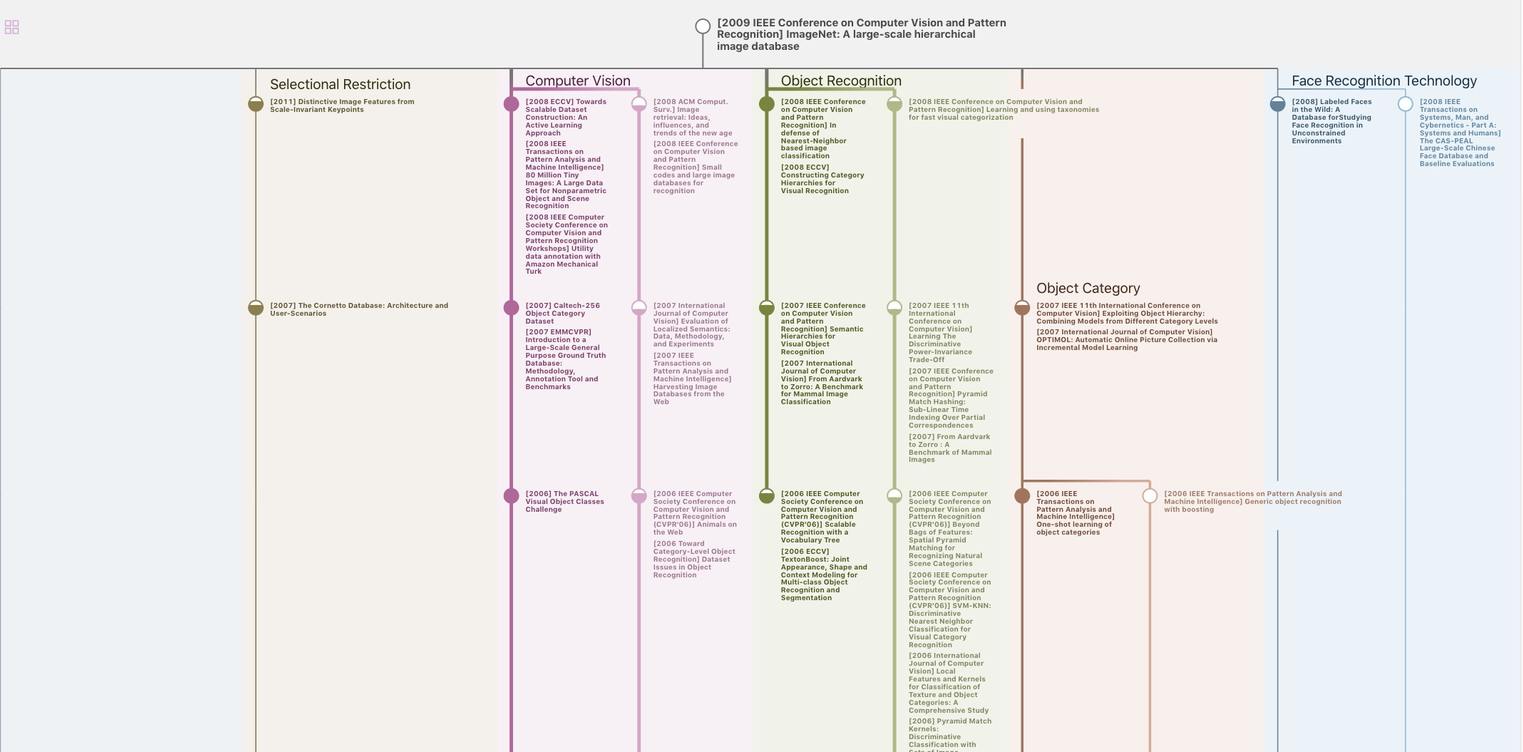
生成溯源树,研究论文发展脉络
Chat Paper
正在生成论文摘要