Unsupervised domain adaptation via progressive positioning of target-class prototypes
Knowledge-Based Systems(2023)
摘要
Domain adaptation transfers knowledge from the source domain to the target domain. The existing methods reduce the domain discrepancy by aligning domain distribution. To align the two domains at category level, a pseudo labeling approach is often adopted. However, unreliable pseudo labels may cause negative transfer problems, which hinders further improvement of domain adaptation methods. To solve this problem, we propose a new unsupervised domain adaptation method via Progressive Positioning of Target-Class Prototypes (P2TCP), in this paper. P2TCP applies the knowledge of the source domain to locate the target class prototypes, then predicts the target samples through exploiting the structural information within the target domain. Inspired by the curriculum learning, we further propose an adaptive-dual label filtering method to improve the model with iteration by an easy-to-hard strategy. Extensive experiments reveal that our method achieves the state-of-the-art on the four benchmark datasets. Our code is available at P2TCP.
更多查看译文
关键词
unsupervised domain adaptation,progressive positioning,target-class
AI 理解论文
溯源树
样例
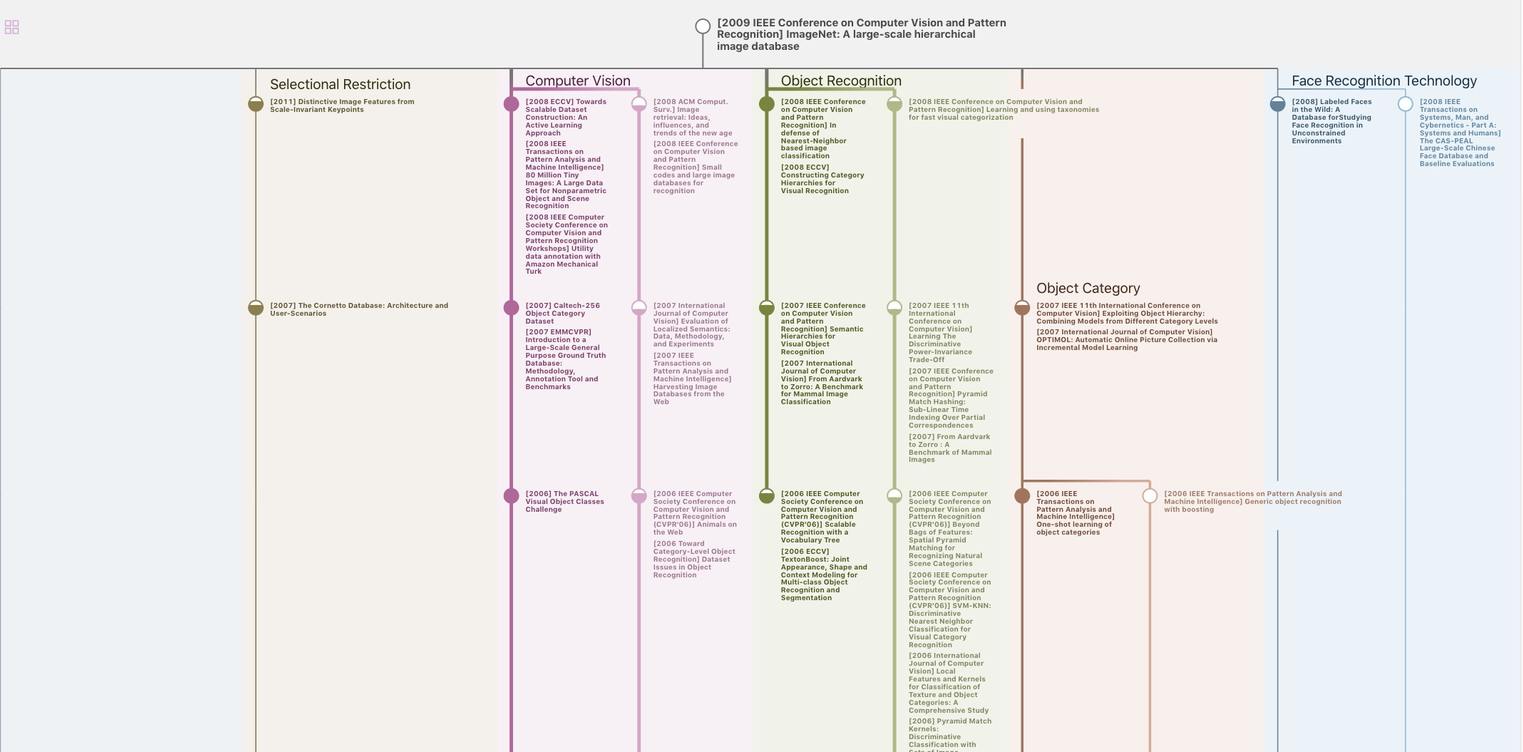
生成溯源树,研究论文发展脉络
Chat Paper
正在生成论文摘要