Multi-fidelity design optimization of solid oxide fuel cells using a Bayesian feature enhanced stochastic collocation
International Journal of Hydrogen Energy(2023)
摘要
We develop a multi-fidelity surrogate modelling approach to replace the complex and costly physics-based computer models that are often used in the optimization of solid oxide fuel cell (SOFC) performance, or the simplified models that are used in lieu of complex models. We extend multi-fidelity stochastic collocation through a feature engineering step, and eliminate the requirement for the exact low-fidelity output at the inference stage. In contrast to previous approaches, the surrogate model we develop provides detailed spatial information, rather than one or more scalar outputs. This allows for the incorporation of such information into the objective of the optimization study, with the flexibility to choose from more than one objective, such as a minimum, maximum or average. Furthermore, the detailed spatial information can be used for general design purposes, such as ensuring uniformity in reactant and potential distributions. From the results on a 3-d SOFC model, we demonstrate highly accurate predictions of multiple spatially distributed quantities at up to spatial 250,000 locations. The results are superior to state-of-the-art multi-fidelity approaches, particularly for low numbers of high fidelity training points. We use the surrogate model to optimize the SOFC performance with respect to different objectives (including with nonlinear constraints and multiple objectives), with results that are accurate and are obtained in a fraction of the time required for the full model.
更多查看译文
关键词
solid oxide fuel cells,bayesian feature,optimization,multi-fidelity
AI 理解论文
溯源树
样例
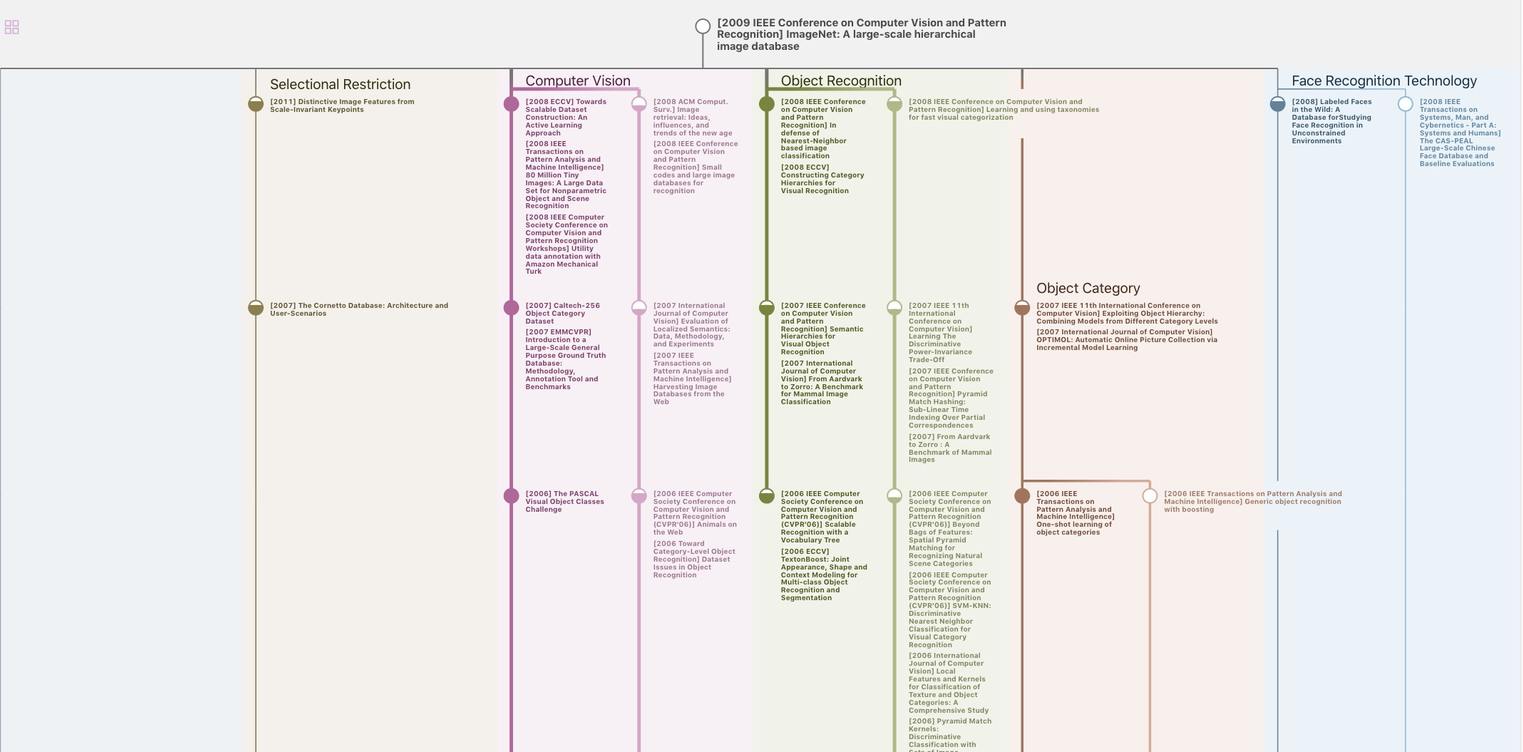
生成溯源树,研究论文发展脉络
Chat Paper
正在生成论文摘要